Fast sparse twin learning framework for large-scale pattern classification
ENGINEERING APPLICATIONS OF ARTIFICIAL INTELLIGENCE(2024)
摘要
Lately, twin support vector machine and its variants have received extensive attention and in-depth research in the field of large-scale pattern classification. However, they may lead to high computational cost, which greatly affects their development and research. Aiming to solve this problem, this paper a novel fast sparse twin learning framework for large-scale data classification is proposed. In this learning framework, by introducing sparse constraints into the dual problem, the number of support vectors can be effectively reduced in dual space, so as to improve the computational speed of the model. Importantly, the learning framework is not only sparsity for large-scale samples classification, but also insensitive to sample noise, thus it is stable for resampling. In addition, we use the modified Newton's method to deal with the optimization problem with sparse constraints. Numerical experiments are carried out on ten large-scale datasets. The results show that the proposed learning framework for large-scale data classification problems has significant advantages and comparability with other learning methods in terms of computational speed and classification accuracy.
更多查看译文
关键词
Large-scale datasets,Twin support vector machine,Twin parametric,Sparsity,Modified newton's method
AI 理解论文
溯源树
样例
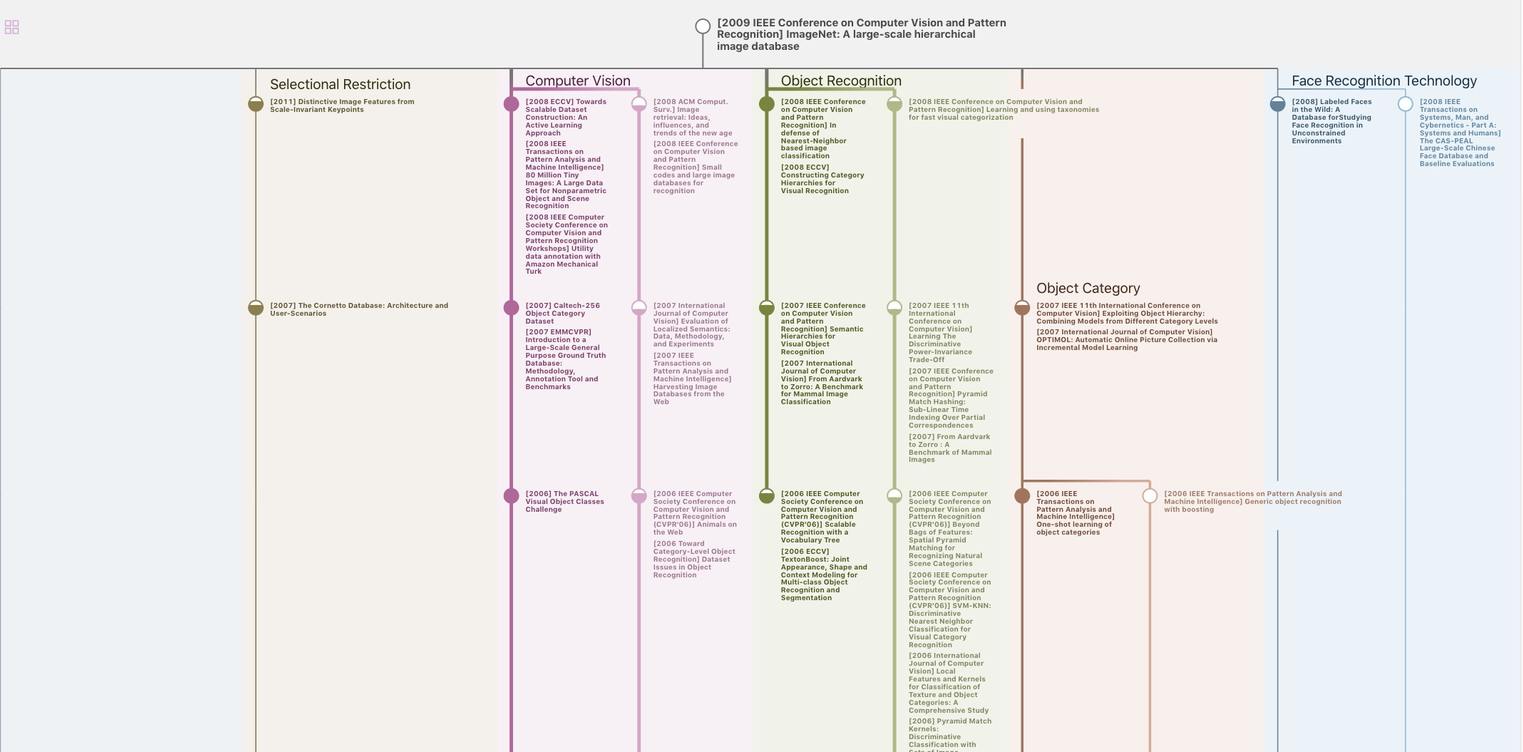
生成溯源树,研究论文发展脉络
Chat Paper
正在生成论文摘要