Multiple fault recognition for chemical processes based on TSK-type neural networks with nonlinear consequences
Granular Computing(2023)
摘要
Fault recognition systems are developed to characterize normal conditions and detect different faults in a process plant, which is important for early warning and diagnosis, especially in chemical processes. This paper proposes a novel fault recognition method via TSK fuzzy system combined with a neural network. In the proposed method, one-dimensional convolution is used to represent the TSK systems to extract fault information and latent fault features. TSK fuzzy system employs the consequences of a nonlinear vector function family, enhancing the expressive capacity of fuzzy rules for multi-dimensional time series data. A TSK fuzzy system, combined with a neural network classifier, is used for fault recognition. The effectiveness of this model is verified on the Tennessee Eastman process (TEP) dataset, which is a benchmark for chemical industrial processes.
更多查看译文
关键词
Takagi-Sugeno-Kang(TSK),Fuzzy classifier,Fuzzy neural network,Fault recognition,Tennessee Eastman process
AI 理解论文
溯源树
样例
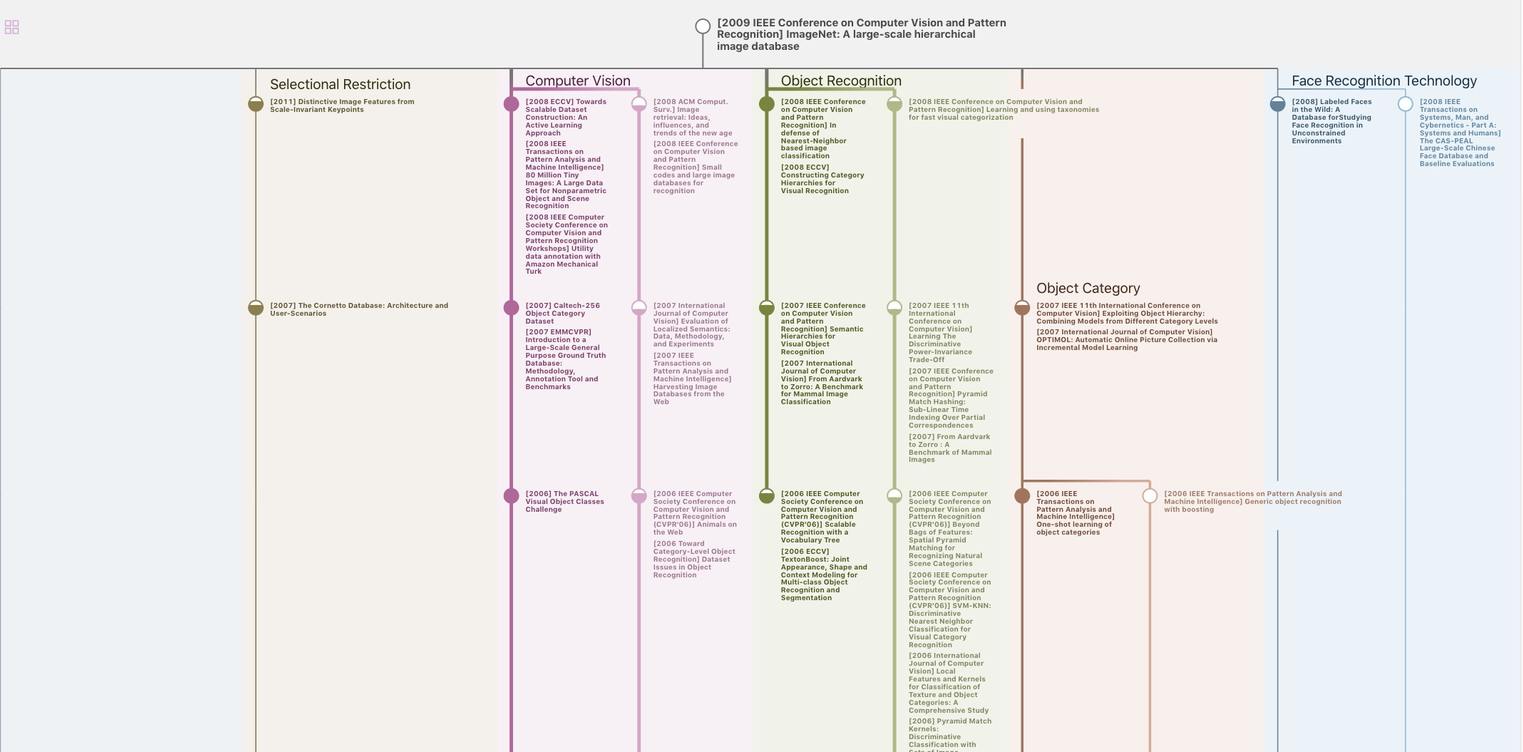
生成溯源树,研究论文发展脉络
Chat Paper
正在生成论文摘要