Serial Dependence Analysis of Time Series Using Distance Correlation and Monte Carlo Bootstrap Hypothesis Test.
2023 14th International Conference on Information, Intelligence, Systems & Applications (IISA)(2023)
摘要
Linear correlation test statistics, e.g., Pearson's correlation coefficient, dominated the time series analysis for decades offering significant achievements as well as featuring limitations. In that framework, the econometric time series were a subset of sequential random variables that pointed out the necessity of extending the time series analysis in the non-linear serial dependence domain. The introduction of distance correlation (dCorr) in 2007 showed potential in that direction. However, the complexity of the accompanying mathematical expressions and restrictive assumptions, e.g., of independent and identically distributed or strictly stationary time series, resulted in relatively slow progress. To tackle this complexity and restrictions, past research works assuming stationary time series introduced various bootstrap procedures to derive the upper 95% confidence bound of the null hypothesis test. In this paper, we introduce a bootstrap procedure based on Monte Carlo simulations instead, providing both upper and lower bounds of null hypothesis. We also discuss distinct types of white noises and how these are related to the null hypothesis. The derived null hypothesis bounds are estimated regardless of the type of the observed time series, and therefore in the case of non-stationary time series as well. The efficiency of the proposed method is demonstrated with three econometric variables, which can be used in currency exchange rates applications.
更多查看译文
关键词
time series,serial dependence,Monte Carlo simulation,distance correlation,white noise,currency exchange rates
AI 理解论文
溯源树
样例
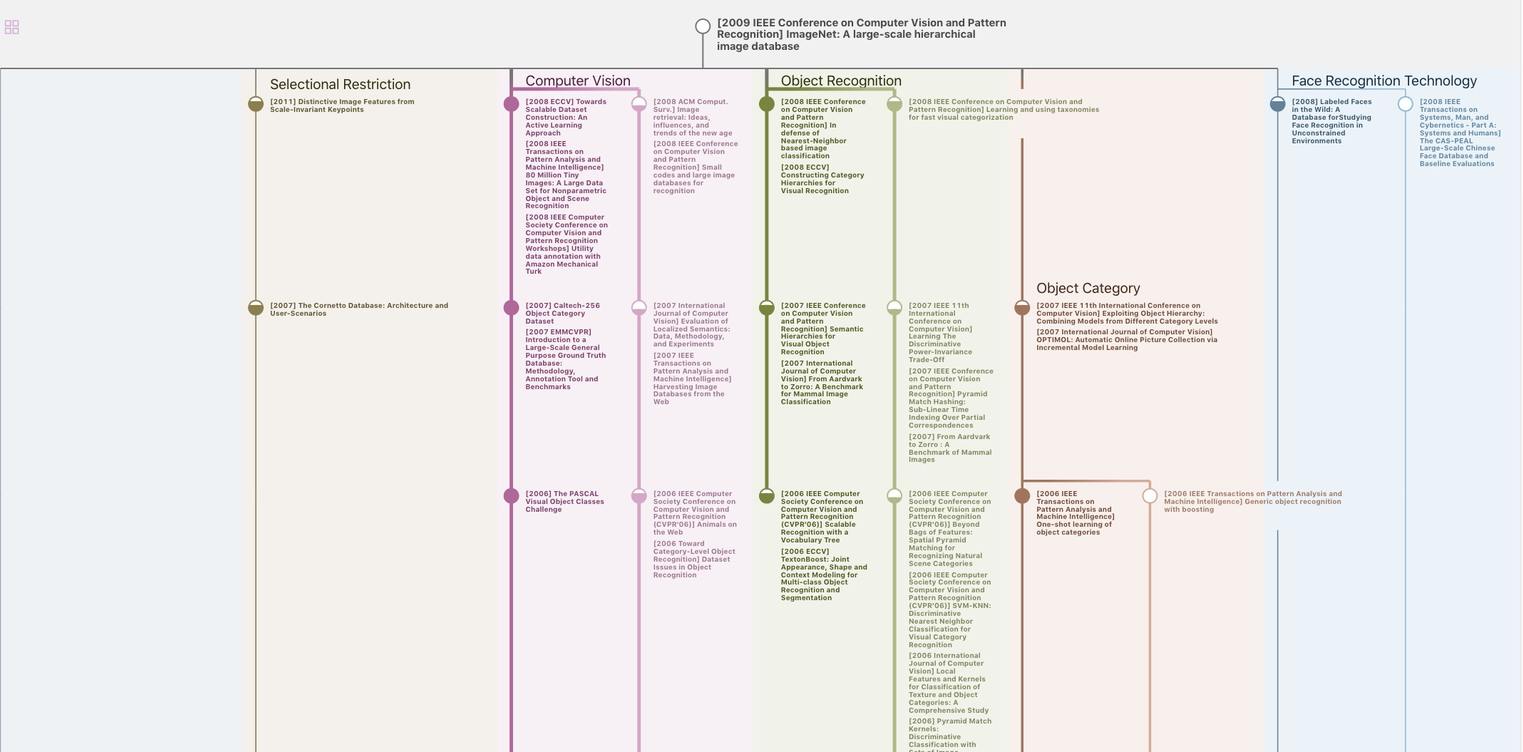
生成溯源树,研究论文发展脉络
Chat Paper
正在生成论文摘要