An Improved Co-Optimization of Component Sizing and Energy Management for Hybrid Powertrains Interacting With High-fidelity Model
IEEE TRANSACTIONS ON VEHICULAR TECHNOLOGY(2023)
摘要
Due to the ignorance of dynamic characteristics of powertrains, existing frameworks for co-optimizing component sizing and energy management of hybrid powertrains may lead to unsatisfactory performance. To design an alternative framework and provide a promising solution, this article proposes a novel co-optimization methodology that incorporates the high-fidelity model (HFM) of plug-in hybrid electric vehicles (PHEVs). The present methodology employs the maps of engines and electric motors during the optimization process. In addition, based on the Pareto optimization method, an adaptive non-dominated sorting genetic algorithm-III (NSGA-III) with chaos sequence (NSGA-CS) is proposed. By setting an objective function that concerns the fuel consumption, battery state of health (SOH), and manufacturing cost, the co-optimization effect of NSGA-CS with HFM (NSGA-CS & HFM) is compared with that of NSGA-III with Willans model (NSGA-CS & WM) using the real-time control strategy. Under the NEDC-WLTC driving cycle, compared with the original vehicle, the NSGA-CS & HFM approach can reduce fuel consumption, battery SOH loss, and system costs by 7.4%, 8.0%, and 6.3%, respectively. The effectiveness of the optimized parameters is verified in the hardware-in-loop (HIL) experiment. These results suggest that the novel co-optimization methodology proposed in this article is effective in optimizing the powertrain parameters and control strategies and can further promote the energy-saving potential of HEV/PHEVs.
更多查看译文
关键词
High-fidelity models,pareto-based optimization,hybrid powertrains,component sizing,energy management
AI 理解论文
溯源树
样例
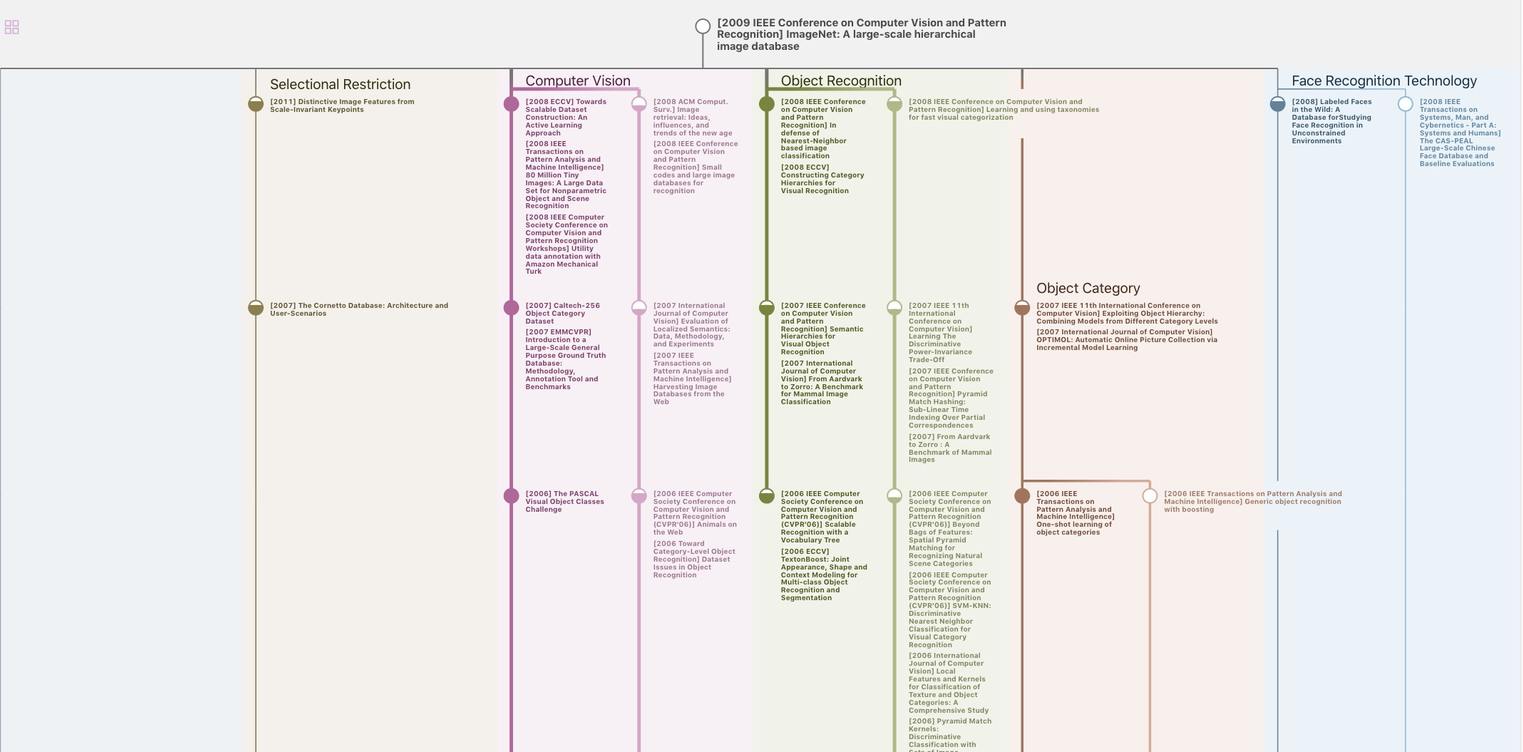
生成溯源树,研究论文发展脉络
Chat Paper
正在生成论文摘要