Risk-Constrained Reinforcement Learning for Inverter-Dominated Power System Controls
IEEE CONTROL SYSTEMS LETTERS(2023)
摘要
This letter develops a risk-aware controller for grid-forming inverters (GFMs) to minimize large frequency oscillations in GFM inverter-dominated power systems. To tackle the high variability from loads/renewables, we incorporate a mean-variance risk constraint into the classical linear quadratic regulator (LQR) formulation for this problem. The risk constraint aims to bound the time-averaged cost of state variability and thus can improve the worst-case performance for large disturbances. The resulting risk-constrained LQR problem is solved through the dual reformulation to a minimax problem, by using a reinforcement learning (RL) method termed as stochastic gradient-descent with max-oracle (SGDmax). In particular, the zero-order policy gradient (ZOPG) approach is used to simplify the gradient estimation using simulated system trajectories. Numerical tests conducted on the IEEE 68-bus system have validated the convergence of our proposed SGDmax for GFM model and corroborate the effectiveness of the risk constraint in improving the worst-case performance while reducing the variability of the overall control cost.
更多查看译文
关键词
Frequency control,grid-forming inverter (GFM),inter-area oscillations,mean-variance risk constraint,reinforcement learning (RL)
AI 理解论文
溯源树
样例
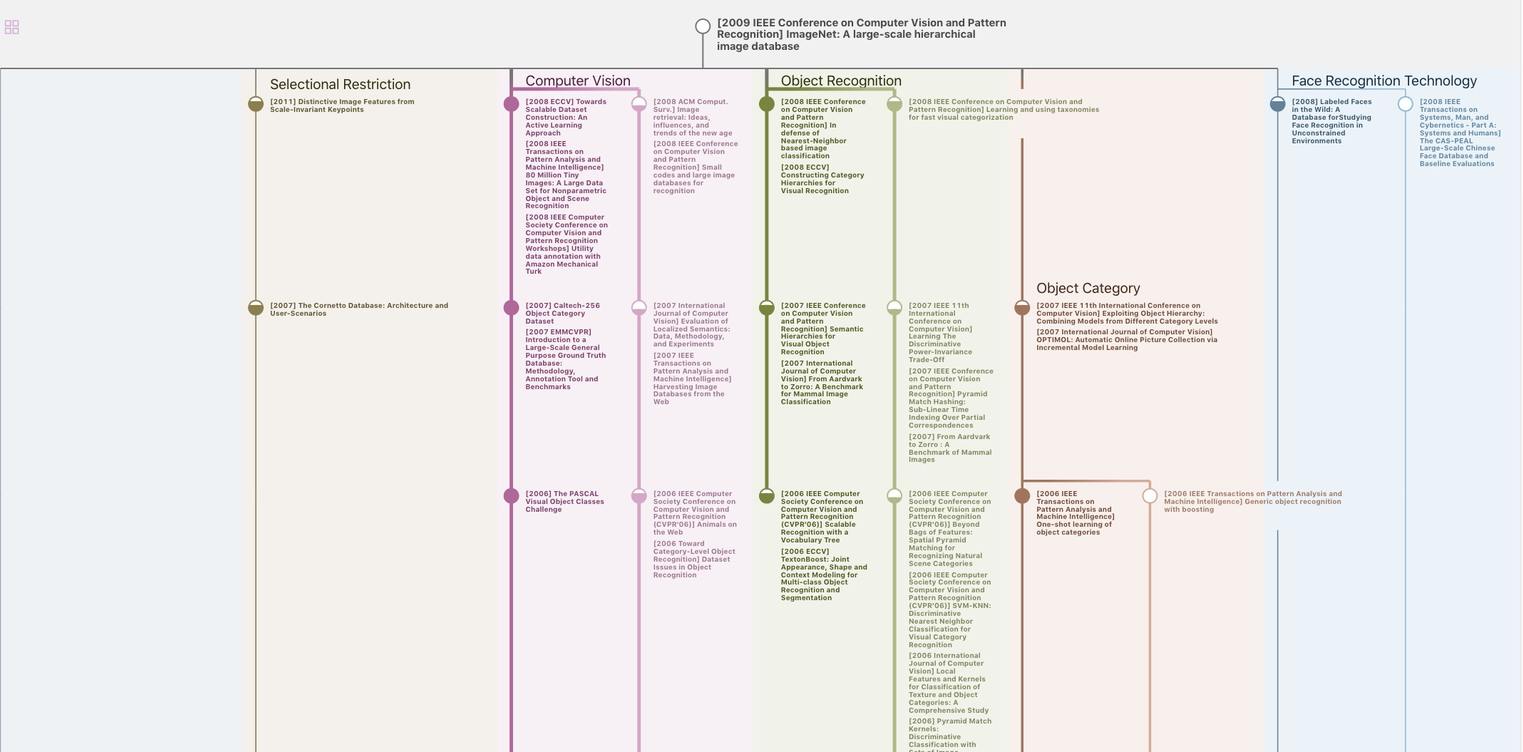
生成溯源树,研究论文发展脉络
Chat Paper
正在生成论文摘要