An Attentive Inductive Bias for Sequential Recommendation beyond the Self-Attention
AAAI 2024(2024)
摘要
Sequential recommendation (SR) models based on Transformers have achieved remarkable successes. The self-attention mechanism of Transformers for computer vision and natural language processing suffers from the oversmoothing problem, i.e., hidden representations becoming similar to tokens. In the SR domain, we, for the first time, show that the same problem occurs. We present pioneering investigations that reveal the low-pass filtering nature of self-attention in the SR, which causes oversmoothing. To this end, we propose a novel method called Beyond Self-Attention for Sequential Recommendation (BSARec), which leverages the Fourier transform to i) inject an inductive bias by considering fine-grained sequential patterns and ii) integrate low and high-frequency information to mitigate oversmoothing. Our discovery shows significant advancements in the SR domain and is expected to bridge the gap for existing Transformer-based SR models. We test our proposed approach through extensive experiments on 6 benchmark datasets. The experimental results demonstrate that our model outperforms 7 baseline methods in terms of recommendation performance. Our code is available at https://github.com/yehjin-shin/BSARec.
更多查看译文
关键词
DMKM: Recommender Systems,DMKM: Mining of Spatial, Temporal or Spatio-Temporal Data
AI 理解论文
溯源树
样例
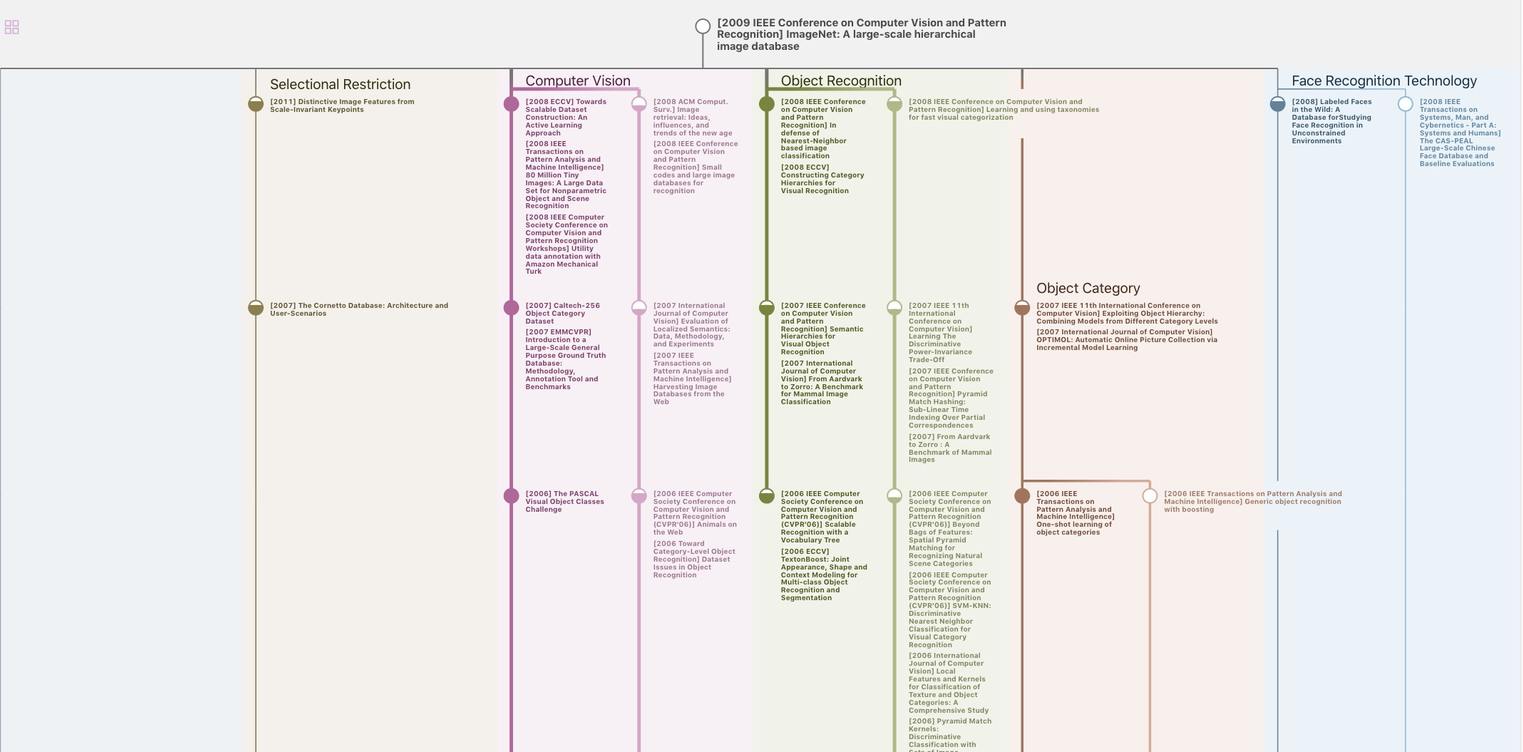
生成溯源树,研究论文发展脉络
Chat Paper
正在生成论文摘要