Multiscale differential geometry learning of networks with applications to single-cell RNA sequencing data
COMPUTERS IN BIOLOGY AND MEDICINE(2024)
摘要
Single -cell RNA sequencing (scRNA-seq) has emerged as a transformative technology, offering unparalleled insights into the intricate landscape of cellular diversity and gene expression dynamics. scRNA-seq analysis represents a challenging and cutting -edge frontier within the field of biological research. Differential geometry serves as a powerful mathematical tool in various applications of scientific research. In this study, we introduce, for the first time, a multiscale differential geometry (MDG) strategy for addressing the challenges encountered in scRNA-seq data analysis. We assume that intrinsic properties of cells lie on a family of low -dimensional manifolds embedded in the high -dimensional space of scRNA-seq data. Multiscale cell-cell interactive manifolds are constructed to reveal complex relationships in the cell-cell network, where curvature -based features for cells can decipher the intricate structural and biological information. We showcase the utility of our novel approach by demonstrating its effectiveness in classifying cell types. This innovative application of differential geometry in scRNA-seq analysis opens new avenues for understanding the intricacies of biological networks and holds great potential for network analysis in other fields.
更多查看译文
关键词
Multiscale differential geometry,scRNA-seq data,Cell interactive manifold,Machine learning,Cell type classification,Dimensionality reduction
AI 理解论文
溯源树
样例
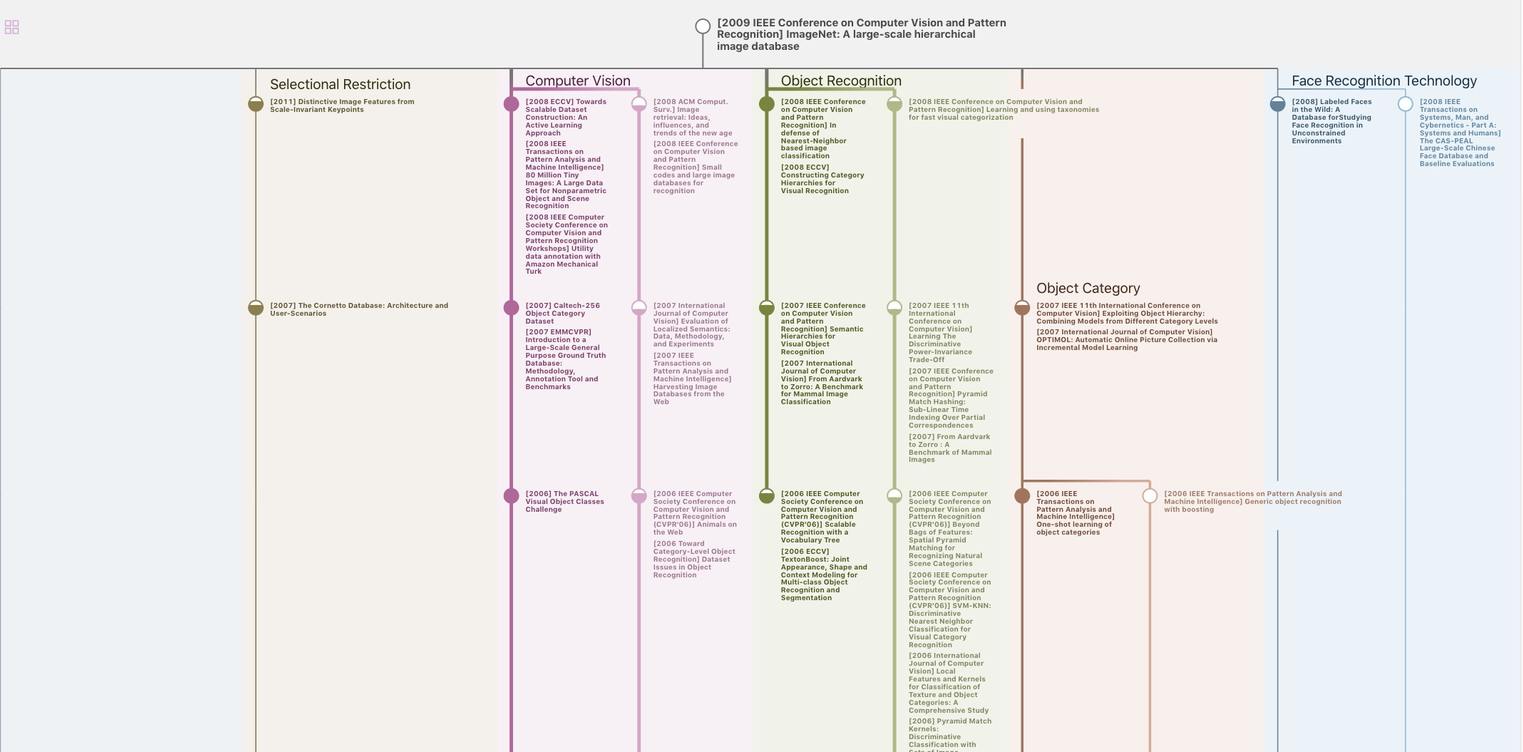
生成溯源树,研究论文发展脉络
Chat Paper
正在生成论文摘要