Resilient Federated Learning under Byzantine Attack in Distributed Nonconvex Optimization with 2-f Redundancy
2023 62ND IEEE CONFERENCE ON DECISION AND CONTROL, CDC(2023)
摘要
We study the problem of Byzantine fault tolerance in a distributed optimization setting, where there is a group of N agents communicating with a trusted centralized coordinator. Among these agents, there is a subset of f agents that may not follow a prescribed algorithm and may share arbitrarily incorrect information with the coordinator. The goal is to find the optimizer of the aggregate cost functions of the honest agents. We will be interested in studying the local gradient descent method, also known as federated learning, to solve this problem. However, this method often returns an approximate value of the underlying optimal solution in the Byzantine setting. Recent work showed that by incorporating the so-called comparative elimination (CE) filter at the coordinator, one can provably mitigate the detrimental impact of Byzantine agents and precisely compute the true optimizer in the convex setting. The focus of the present work is to provide theoretical results to show the convergence of local gradient methods with the CE filter in a nonconvex setting. We will also provide a number of numerical simulations to support our theoretical results.
更多查看译文
AI 理解论文
溯源树
样例
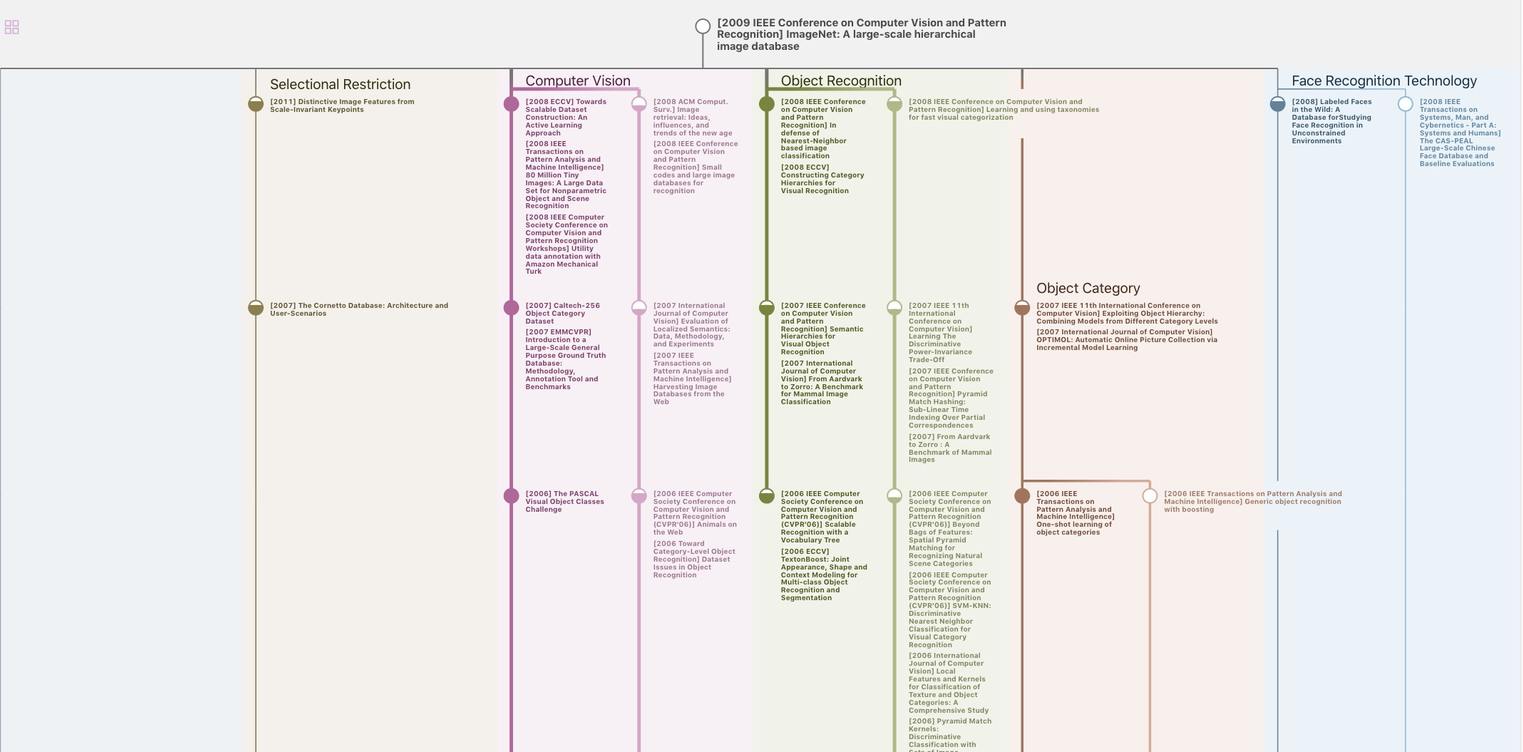
生成溯源树,研究论文发展脉络
Chat Paper
正在生成论文摘要