DOMR: Toward Deep Open-World Malware Recognition
IEEE TRANSACTIONS ON INFORMATION FORENSICS AND SECURITY(2024)
摘要
Deep learning has been widely used for Android malware family recognition, but current deep learning-based approaches make the closed-world assumption that malware families encountered during testing are available at training phase. Unfortunately, this assumption is often violated in practice due to the constant emergence of novel categories and the huge cost of collecting abundant training classes, causing serious failures to the existing approaches. Accordingly, a new problem setting for Android malware family recognition is introduced, i.e., deep open-world malware recognition that poses two critical tasks: 1) Open recognition, aiming to not only classify malware from known families (present in training) but detect malware from unknown families (absent in training); 2) Incremental update, aiming to learn about the detected unknown/new categories without retraining from scratch and catastrophically forgetting the previously learned known/old classes. This paper formalizes the problem and proposes a novel solution called DOMR to address the above two tasks in a unified framework. The core of DOMR is an episode-based representation learning scheme that mimics the open-world setting through episodic training to learn a generalizable representation. The key insight is that the training process following the open-world setting forces the representation to accumulate experience in open recognition, thereby facilitating both the classification of known family instances and the detection of unknown family instances at inference. Given this representation, multiple one-vs-rest classifiers are subsequently built to make the final recognition decision through an aggregative strategy. Comparative experiments show that DOMR outperforms start-of-the-art methods, with macro-averaged F1-scores obtained on two datasets reaching 80.88% and 56.17% in the open case, and 79.34% and 49.55% in the incremental case, respectively. Ablation studies further analyze the effectiveness of DOMR in achieving the open recognition and incremental update goals.
更多查看译文
关键词
Android malware,malware family recognition,open-world malware recognition,deep learning,meta-learning
AI 理解论文
溯源树
样例
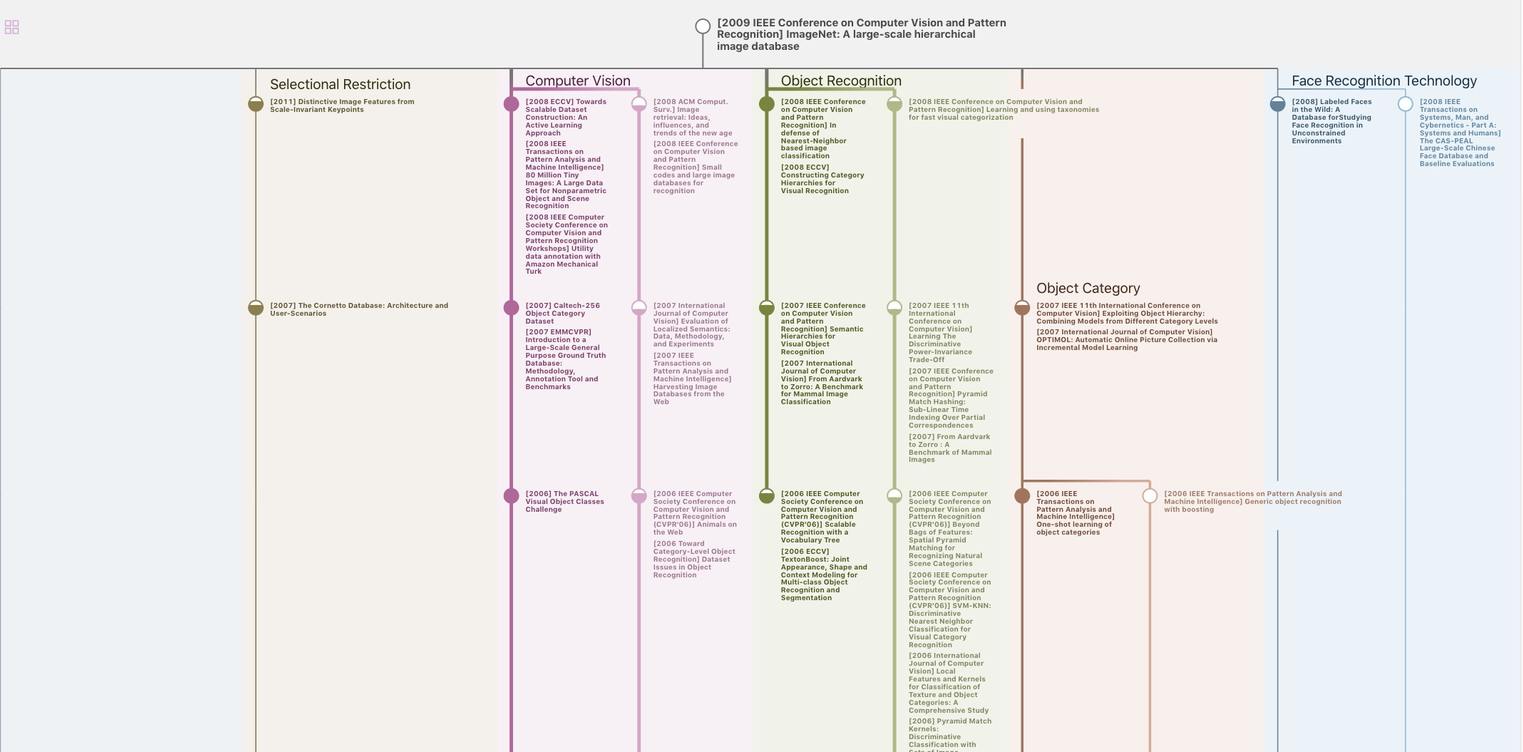
生成溯源树,研究论文发展脉络
Chat Paper
正在生成论文摘要