Deep learning-based dynamic parametric image generation with static perfusion computed tomography
2023 IEEE Nuclear Science Symposium, Medical Imaging Conference and International Symposium on Room-Temperature Semiconductor Detectors (NSS MIC RTSD)(2023)
摘要
Myocardial blood flow can reflect the hemodynamic status of the myocardium and play a crucial role in the diagnosis of myocardial ischemia. However, the derivation of myocardial blood flow requires a series of dynamic-computed tomography perfusion scans, which lead to high radiation exposure. We explore a convolutional neural network-based parametric image generation method for accessing parametric images without dynamic scans. With a simulated static computed tomography perfusion scan as the input, the network can generate corresponding myocardial blood flow. With the assistance of bolus triggering, this method can realize accessibility of myocardial blood flow even without dynamic computed tomography perfusion, therefore reducing the radiation dosage in radiology examination and significantly boosting the duration of postprocessing. First, we pretrained the network with the averaged dynamic computed tomography perfusion scans for better default parameters. Fine-tuning with the simulated static computed tomography perfusion scans was then implemented for desired end-to-end image translation. The performance of the issued image translation method was evaluated by using the usual image rating criteria and a clinical functional assessment. The results illustrated that the synthetic image quality is fine and that the clinical functionality of the synthetic myocardial blood flow is satisfactory. The proposed method showed great potential in dose reduction; the effective dosage was reduced by 90% in our cases and the postprocessing time was shortened from 19 minutes to approximately 10 seconds.
更多查看译文
AI 理解论文
溯源树
样例
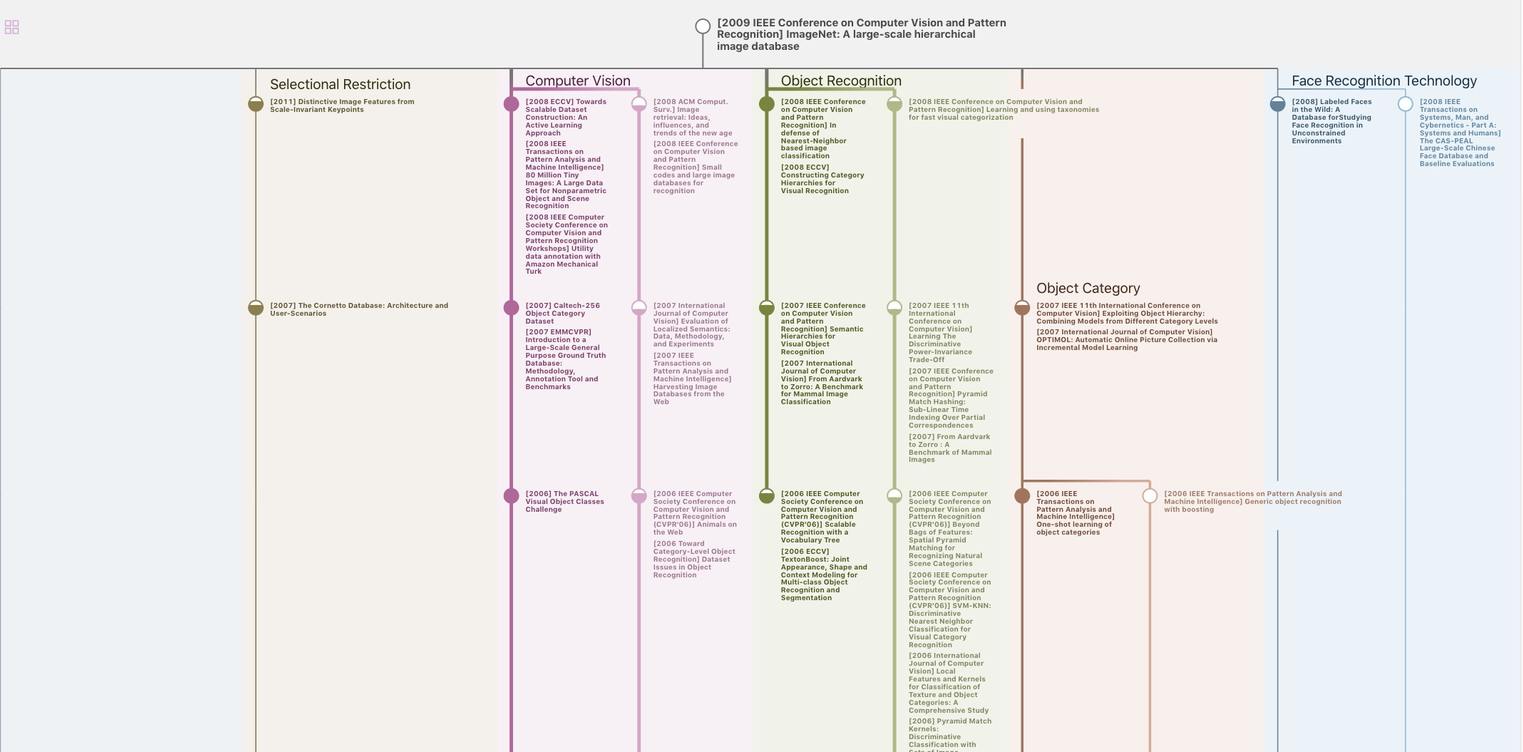
生成溯源树,研究论文发展脉络
Chat Paper
正在生成论文摘要