Few-shot Segmentation and Semantic Segmentation for Underwater Imagery
2023 IEEE/RSJ INTERNATIONAL CONFERENCE ON INTELLIGENT ROBOTS AND SYSTEMS (IROS)(2023)
摘要
This paper tackles image segmentation problems for underwater environments. First, we introduce a novel underwater animal-centric dataset with dense pixel-level annotations containing diverse fine-grained animal categories to mitigate the lack of diverse categories in the existing benchmarks. Then, we solve two image segmentation tasks using underwater images in this dataset: (i) few-shot segmentation, and (ii) semantic segmentation. For the segmentation task in a few-shot learning framework, we propose a novel attention-guided deep neural network architecture by infusing attention modules in various stages of our proposed network. We systematically explore how the learned attention maps can improve few-shot segmentation performance for underwater imagery. Finally, we assess the semantic segmentation problem on our proposed dataset by benchmarking it with two state-of-the-art semantic segmentation methods. We believe our new problem setup, i.e., few-shot segmentation for underwater environments, will be a valuable addition to the existing underwater semantic segmentation task. We believe our novel dataset will pave the way for developing better algorithms and exploring new research directions for marine robotics and underwater image understanding. We publicly release our dataset and the code to advance image understanding research in underwater environments: https://github.com/Imran2205/uwsnet.
更多查看译文
AI 理解论文
溯源树
样例
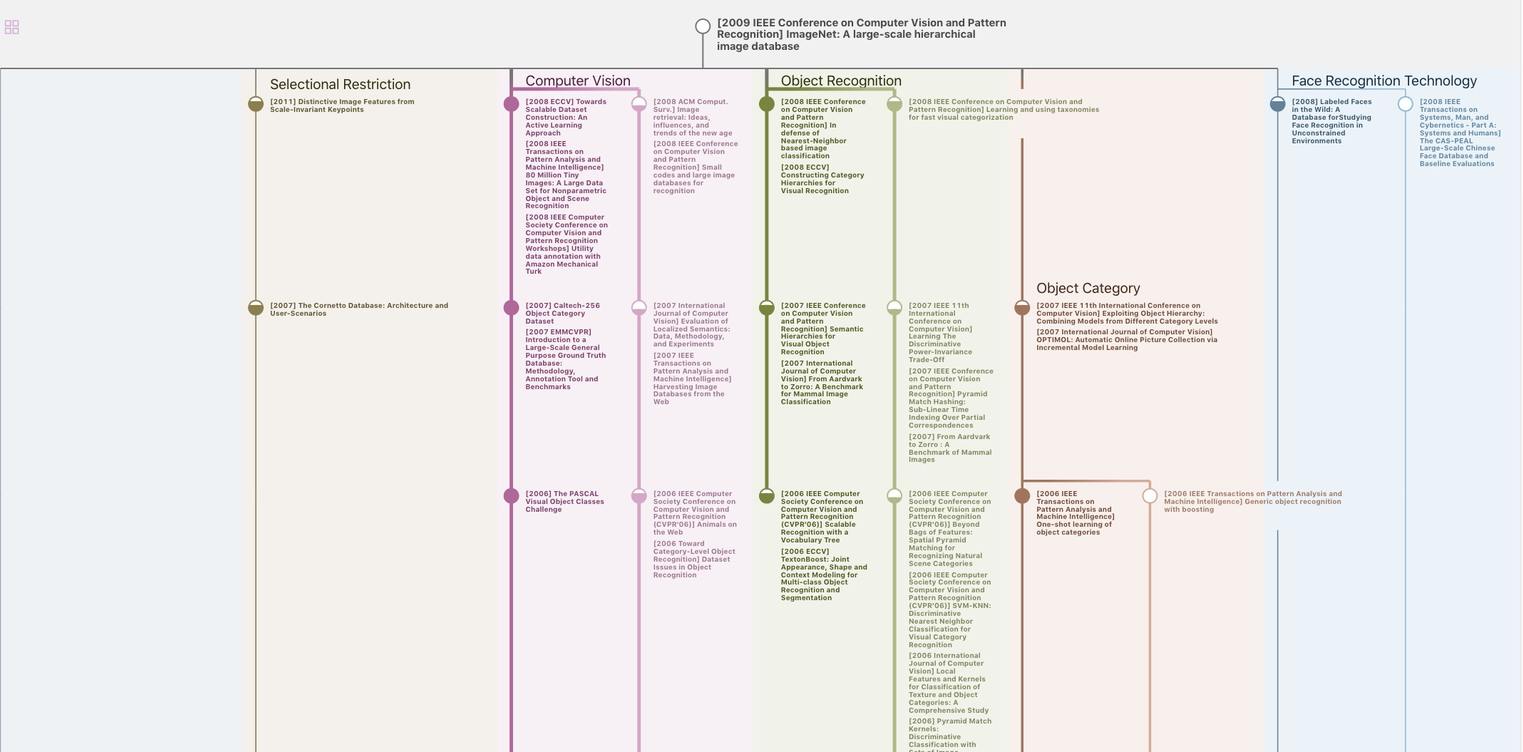
生成溯源树,研究论文发展脉络
Chat Paper
正在生成论文摘要