Generating Scenarios from High-Level Specifications for Object Rearrangement Tasks
2023 IEEE/RSJ INTERNATIONAL CONFERENCE ON INTELLIGENT ROBOTS AND SYSTEMS (IROS)(2023)
摘要
Rearranging objects is an essential skill for robots. To quickly teach robots new rearrangements tasks, we would like to generate training scenarios from high-level specifications that define the relative placement of objects for the task at hand. Ideally, to guide the robot's learning we also want to be able to rank these scenarios according to their difficulty. Prior work has shown how generating diverse scenario from specifications and providing the robot with easy-to-difficult samples can improve the learning. Yet, existing scenario generation methods typically cannot generate diverse scenarios while controlling their difficulty. We address this challenge by conditioning generative models on spatial logic specifications to generate spatially-structured scenarios that meet the specification and desired difficulty level. Our experiments showed that generative models are more effective and data-efficient than rejection sampling and that the spatially-structured scenarios can drastically improve training of downstream tasks by orders of magnitude.
更多查看译文
AI 理解论文
溯源树
样例
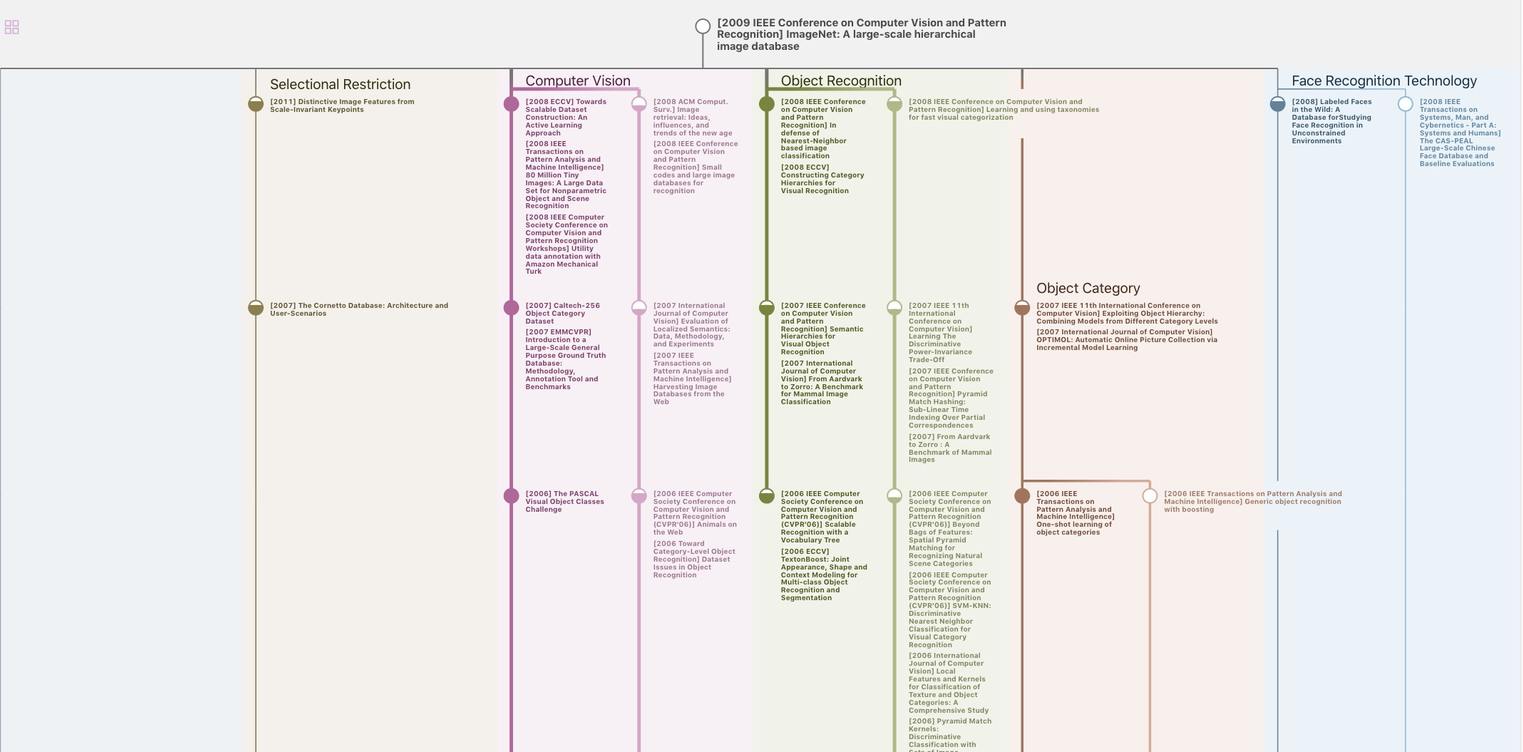
生成溯源树,研究论文发展脉络
Chat Paper
正在生成论文摘要