Real-time Failure-adaptive Control for Dynamic Robots
2023 IEEE/RSJ INTERNATIONAL CONFERENCE ON INTELLIGENT ROBOTS AND SYSTEMS (IROS)(2023)
摘要
The human world is full of risks that threaten failure of robotic tasks. Dynamic robots, such as agile drones and walking bipeds, are particularly susceptible to failure because their time to make critical decisions is short. This work seeks a control algorithm which adapts to failures and reprioritizes robot behavior automatically, all at real-time speeds. Our failure-adaptive control framework learns failure probabilities from in situ experience and minimizes the risk of future failures using fast online planners (i.e. model predictive control). By reasoning about probabilities of failure, more imminent risks are automatically prioritized by the framework without manually tuning weighting factors. Further, our low-order probability model is learned using fast convex optimizations, allowing for immediate learning from triggered failures during operation. We demonstrate the framework's capability to learn and plan in real time (< 20 ms) in highly dynamic scenarios with micro-aerial vehicles (i.e. drones). We conduct two experiments: a chase-avoid task, and a chase-avoid-track task. In both scenarios, a single failure causes a categorical shift in robot behavior and the drone will adapt, plan, and execute a non-failing strategy within one second post-failure.
更多查看译文
AI 理解论文
溯源树
样例
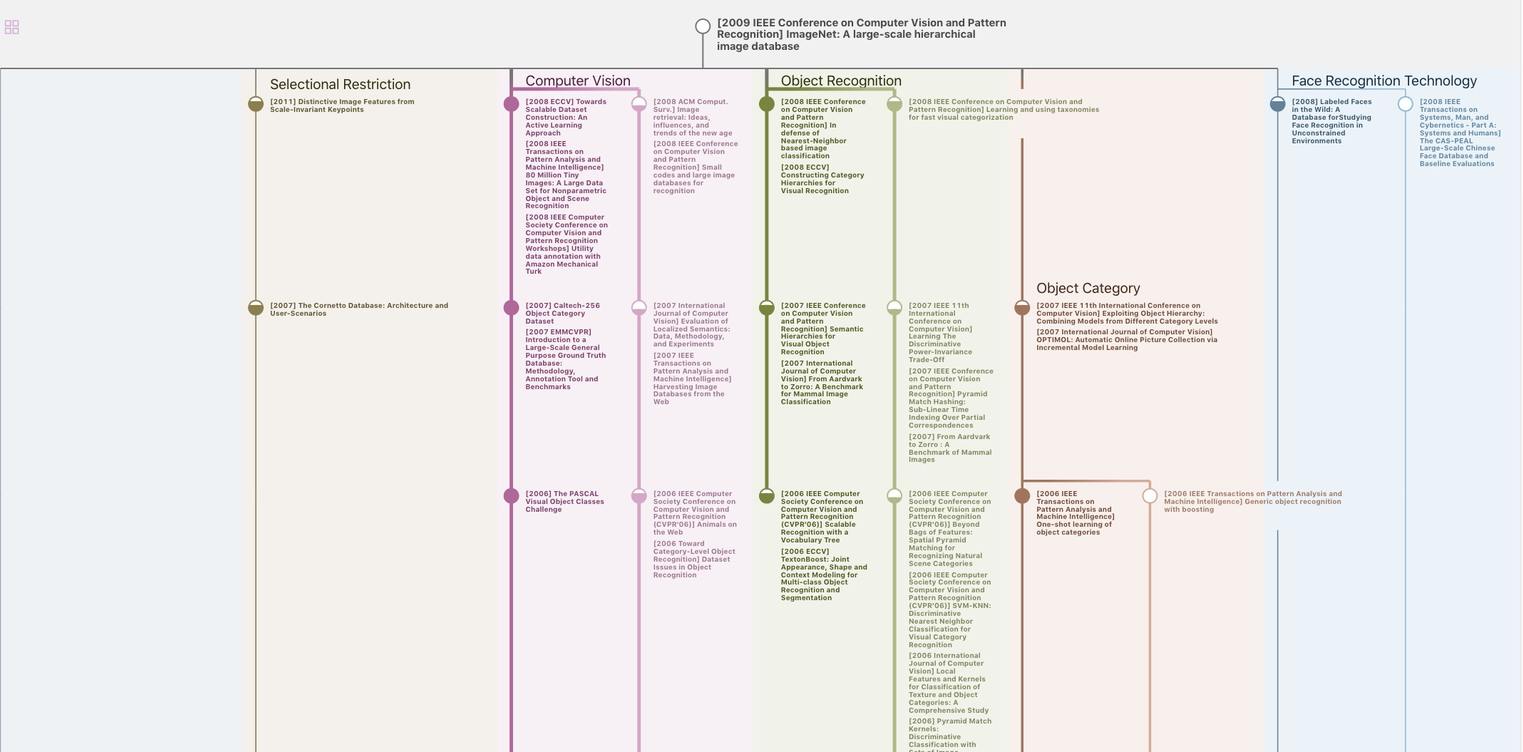
生成溯源树,研究论文发展脉络
Chat Paper
正在生成论文摘要