Dual Variable Actor-Critic for Adaptive Safe Reinforcement Learning
2023 IEEE/RSJ INTERNATIONAL CONFERENCE ON INTELLIGENT ROBOTS AND SYSTEMS (IROS)(2023)
摘要
Satisfying safety constraints in reinforcement learning (RL) is an important issue, especially in real-world applications. Many studies have approached safe RL with the Lagrangian method, which introduces dual variables. However, applying a trained policy with the optimal dual variable to a new environment can be hazardous since the optimal value of the dual variable, which represents a level of safety, depends on the environmental setting. To this end, we propose a new framework, dual variable actor-critic (DVAC), that solves the safe RL problem by simultaneously training a single policy over different safety levels. We introduce a universal policy and universal Q-function, which have a dual variable as an argument. Then, we extend the soft actor-critic so that the universal policy is guaranteed to converge to the Pareto optimal policy sets. We evaluate the proposed method in simulation and real-world environments. The universal policy learned with the proposed method ranges from extremely safe to high performance according to the dual variables, and is nearly Pareto optimal compared to policies learned with the baseline methods. In addition, the agent is able to adapt to environments with unseen state distributions without additional training by identifying a suitable dual variable using the proposed method.
更多查看译文
AI 理解论文
溯源树
样例
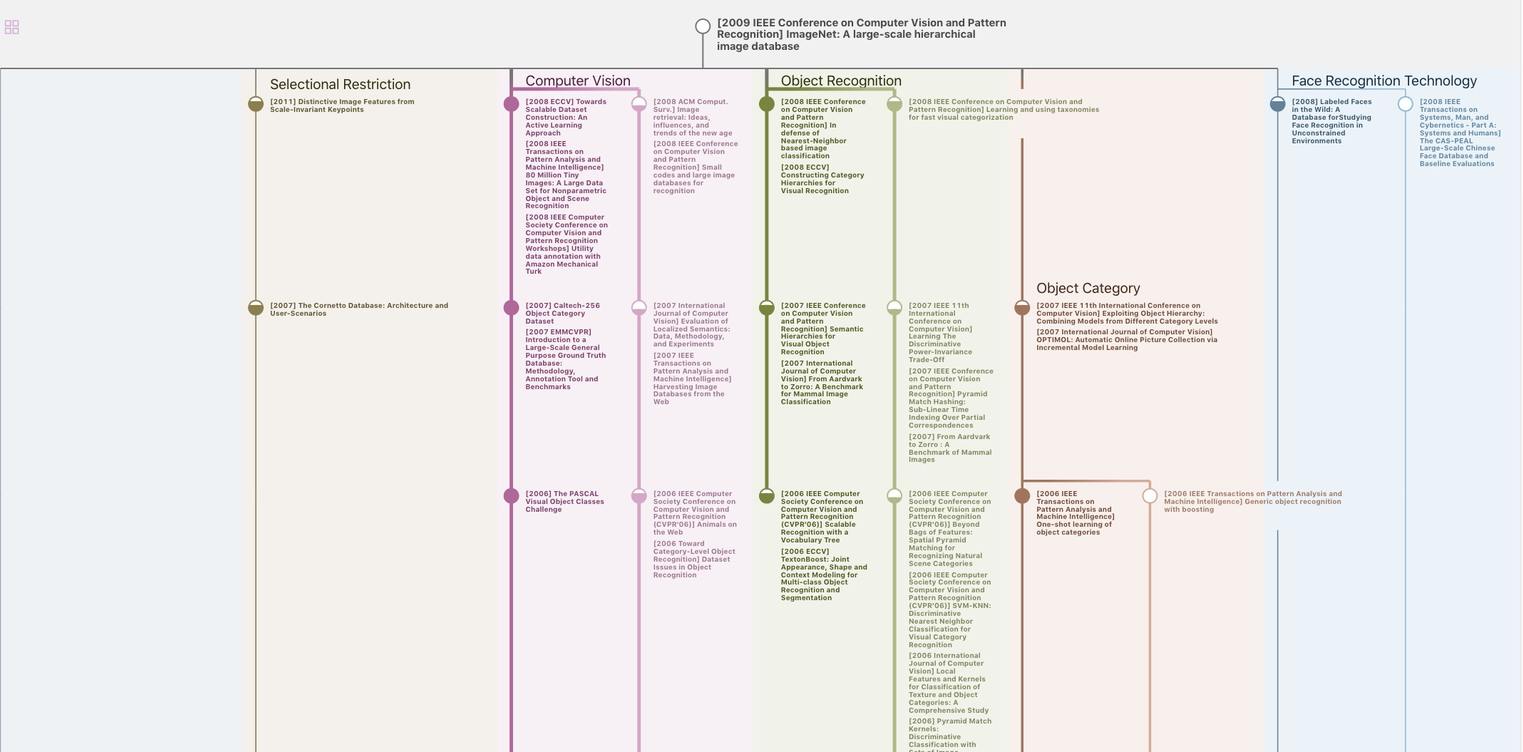
生成溯源树,研究论文发展脉络
Chat Paper
正在生成论文摘要