Risk-Sensitive Mobile Robot Navigation in Crowded Environment via Offline Reinforcement Learning
2023 IEEE/RSJ INTERNATIONAL CONFERENCE ON INTELLIGENT ROBOTS AND SYSTEMS (IROS)(2023)
摘要
Mobile robot navigation in a human-populated environment has been of great interest to the research community in recent years, referred to as crowd navigation. Currently, offline reinforcement learning (RL)-based method has been introduced to this domain, for its ability to alleviate the sim2real gap brought by online RL which relies on simulators to execute training, and its scalability to use the same dataset to train for differently customized rewards. However, the performance of the navigation policy suffered from the distributional shift between the training data and the input during deployment, since when it gets an input out of the training data distribution, the learned policy has the risk of choosing an erroneous action that leads to catastrophic failure such as colliding with a human. To realize risk sensitivity and improve the safety of the offline RL agent during deployment, this work proposes a multipolicy control framework that combines offline RL navigation policy with a risk detector and a force-based risk-avoiding policy. In particular, a Lyapunov density model is learned using the latent feature of the offline RL policy and works as a risk detector to switch the control to the risk-avoiding policy when the robot has a tendency to go out of the area supported by the training data. Experimental results showed that the proposed method was able to learn navigation in a crowded scene from the offline trajectory dataset and the risk detector substantially reduces the collision rate of the vanilla offline RL agent while maintaining the navigation efficiency outperforming the state-of-the-art methods.
更多查看译文
AI 理解论文
溯源树
样例
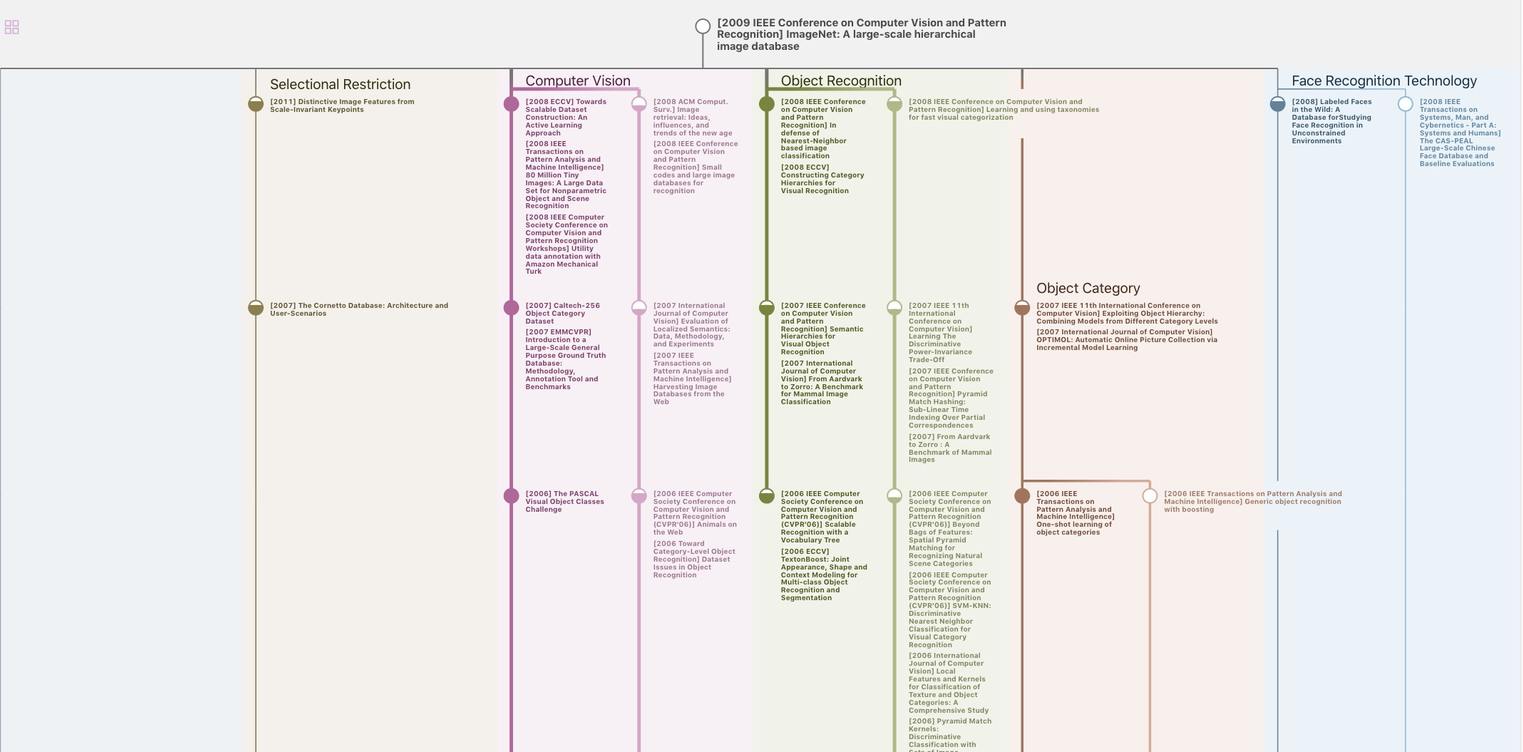
生成溯源树,研究论文发展脉络
Chat Paper
正在生成论文摘要