All Aware Robot Navigation in Human Environments Using Deep Reinforcement Learning
2023 IEEE/RSJ INTERNATIONAL CONFERENCE ON INTELLIGENT ROBOTS AND SYSTEMS (IROS)(2023)
摘要
Mobile robots functioning in human environments should behave with a secure and socially-compliant manner. Although many studies have revealed the effectiveness of Deep Reinforcement Learning (DRL) in robot navigation, most of them can only handle the presence of human as independent individuals. Failing to consider groups may lead to the robot getting stuck or behaving rudely, and omitting to separately handle obstacles from pedestrians will cause low efficiency. In this work, we present a novel all-aware neural network that utilizes DRL to process groups, obstacles, and individuals simultaneously. The proposed solution employs a new Group-Robot Interaction (GRI) subnetwork to encode the mutual effects between groups and the robot, and a modified Obstacle-Robot Unilateral interaction (ORU) subnetwork is presented to avoid obstacle collisions caused by sensing noises or motion uncertainties. In addition, the influences of a pedestrian, obstacle, and group on other pedestrians or groups, that indirectly affect the robot, are also integrated into the Human-Robot Interaction (HRI) subnetwork or GRI subnetwork respectively by using map tensors. Finally, the GRI, ORU, and HRI subnetworks are aggregated into a planning subnetwork to train and derive an all-aware robot navigation policy based on DRL. Evaluation results in both real-world and simulation experiments show that the proposed approach outperforms the current cutting-edge methods.
更多查看译文
AI 理解论文
溯源树
样例
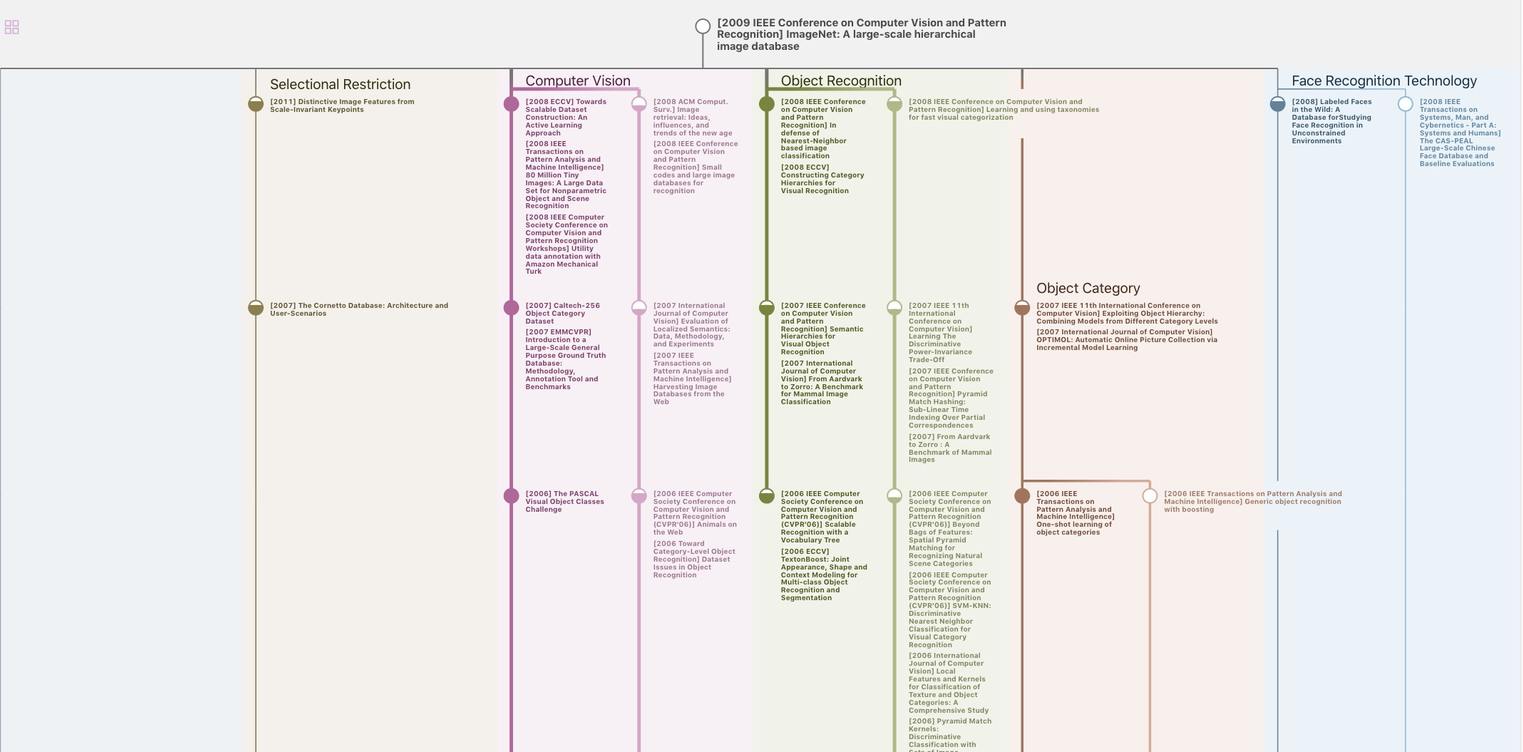
生成溯源树,研究论文发展脉络
Chat Paper
正在生成论文摘要