DMCL: Robot Autonomous Navigation Via Depth Image Masked Contrastive Learning
2023 IEEE/RSJ INTERNATIONAL CONFERENCE ON INTELLIGENT ROBOTS AND SYSTEMS (IROS)(2023)
摘要
Achieving high performance in deep reinforcement learning relies heavily on the ability to obtain good state representations from pixel inputs. However, learning an observation-space-to-action-space mapping from high-dimensional inputs is challenging in reinforcement learning, particularly when dealing with consecutive depth images as input states. In addition, we observe that the consecutive inputs of depth images are highly correlated for the autonomous navigation of a mobile robot, which inspires us to capture temporal correlations between consecutive inputs and infer scene change relationships. To this end, we propose a novel end-to-end robot vision navigation method dubbed DMCL, which obtains good spatial-temporal state representation via Depth image Masked Contrastive Learning. It reconstructs the latent representation from consecutive depth images masked in both spatial and temporal dimensions, resulting in a complete environment state representation. To obtain the optimal navigation policy, we leverage the Soft Actor-Critic reinforcement learning in conjunction with the above representation learning. Extensive experiments demonstrate that the proposed DMCL outperforms representative state-of-the-art methods. The source code will be made publicly available.
更多查看译文
AI 理解论文
溯源树
样例
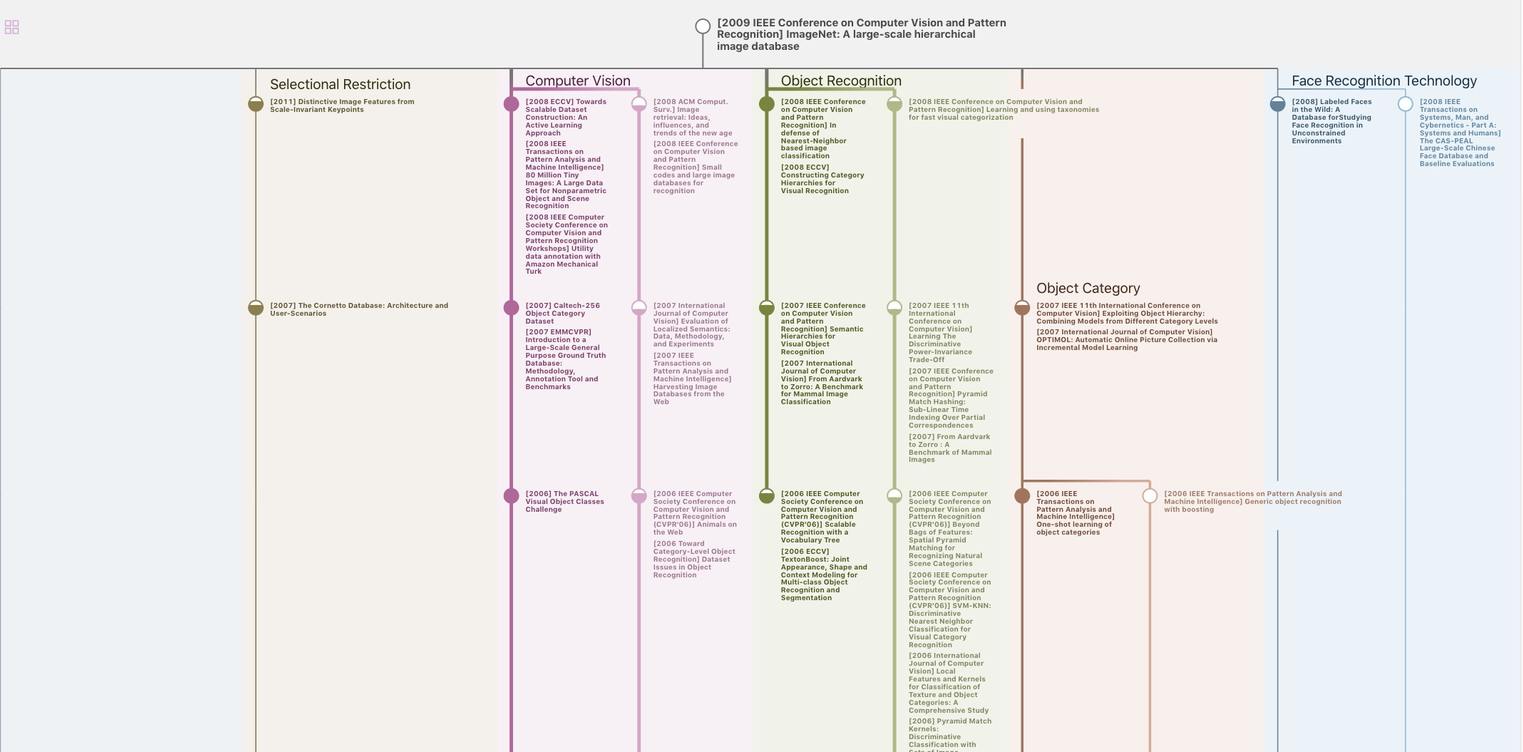
生成溯源树,研究论文发展脉络
Chat Paper
正在生成论文摘要