Augmentation Enables One-Shot Generalization In Learning From Demonstration for Contact-Rich Manipulation
2023 IEEE/RSJ INTERNATIONAL CONFERENCE ON INTELLIGENT ROBOTS AND SYSTEMS, IROS(2023)
摘要
We introduce a Learning from Demonstration (LfD) approach for contact-rich manipulation tasks, i.e., tasks in which the manipulandum's motion is constrained by contact with the environment. Our approach is motivated by the insight that even a large number of demonstrations will often not contain sufficient information to obtain a general policy for the task. To obtain general policies, our approach augments the information contained in a single demonstration. This autonomous augmentation is based on the insight that environmental constraints play a central role in generalization. We validate our approach in real-world experiments with mechanisms with multiple, interdependent articulations, including latch locks, chain locks, and drawers with handles. The extracted policies, obtained from a single augmented human demonstration, generalize to different mechanisms of the same type and in varying environmental settings.
更多查看译文
AI 理解论文
溯源树
样例
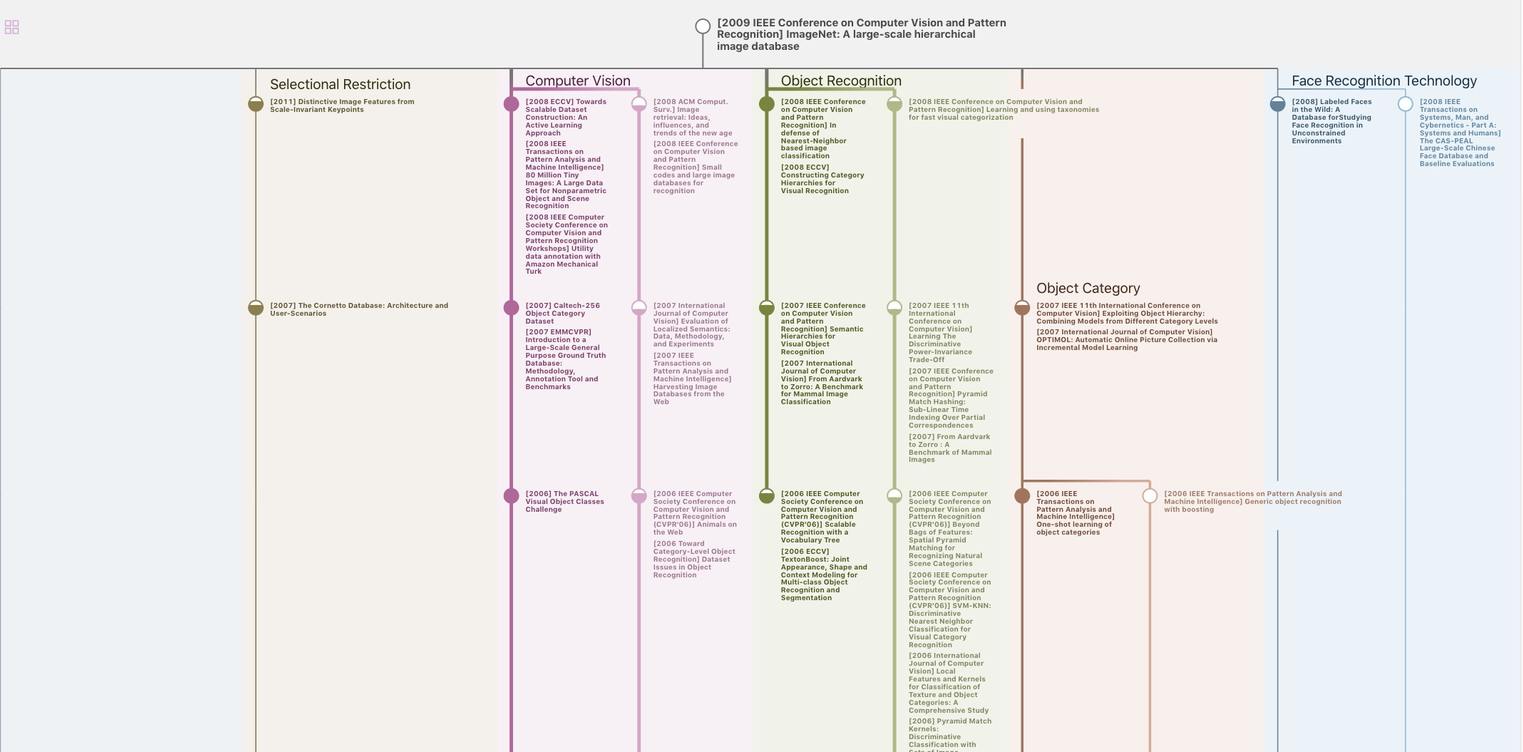
生成溯源树,研究论文发展脉络
Chat Paper
正在生成论文摘要