Model-based Adversarial Imitation Learning from Demonstrations and Human Reward
2023 IEEE/RSJ INTERNATIONAL CONFERENCE ON INTELLIGENT ROBOTS AND SYSTEMS, IROS(2023)
摘要
Reinforcement learning (RL) can potentially be applied to real-world robot control in complex and uncertain environments. However, it is difficult or even unpractical to design an efficient reward function for various tasks, especially those large and high-dimensional environments. Generative adversarial imitation learning (GAIL) - a general model-free imitation learning method, allows robots to directly learn policies from expert trajectories in large and high-dimensional environments. However, GAIL is still sample inefficient in terms of environmental interaction. In this paper, to solve this problem, we propose a model-based adversarial imitation learning from demonstrations and human reward (MAILDH), a novel model-based interactive imitation framework combining the advantages of GAIL, interactive RL and model-based RL. We tested our method in eight physics-based discrete and continuous control tasks for RL. Our results show that MAILDH can greatly improve the sample efficiency and robustness compared to the original GAIL.
更多查看译文
AI 理解论文
溯源树
样例
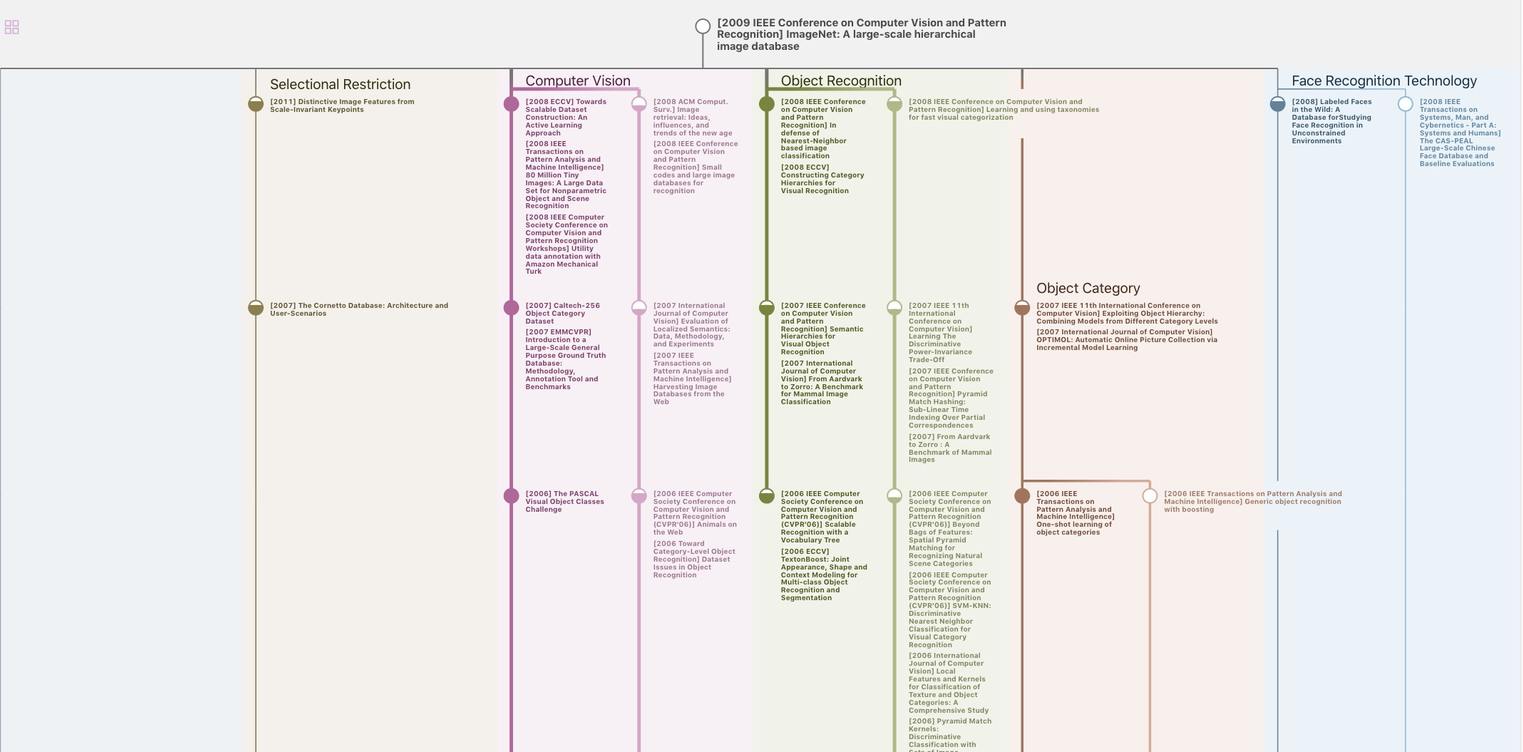
生成溯源树,研究论文发展脉络
Chat Paper
正在生成论文摘要