Temporal Speciation Network for Few-Shot Object Detection
IEEE TRANSACTIONS ON MULTIMEDIA(2023)
摘要
Recently, few-shot object detection (FSOD) has become an increasing research focus, which can largely alleviate the heavy dependency on expensive annotations in the traditional object detection task. However, existing FSOD approaches fail to generate sufficient high-quality positive region proposals which are the key to detection performance, due to the lack of informative knowledge from base classes and non-specific alteration for novel classes. To address the problem, this paper presents a simple yet effective few-shot object detection framework referred to as Temporal Speciation Network (TeSNet) with an evolving training, which improves the diversity and rationality of positive proposal generation. Our TeSNet, imitating the natural evolution which relies on inheritation and mutation, correspondingly consists of two key components: a Selective Recombination Module (SRM) for effectively inheriting from base classes and a Mutational Region Proposal Network (MRPN) for flexibly mutating according to the unique traits of novel samples. Specifically, SRM selects and reorganizes relevant base categories, and further instantiates diverse individuals to ensure the diversity of positive proposals. MRPN adapts the parameters trained on base classes aiming for accurately locating positive proposals. Extensive experiments are conducted on several commonly-used datasets, in which our TeSNet achieves state-of-the-art results and outperforms baselines by large margin.
更多查看译文
关键词
Proposals,Training,Object detection,Task analysis,Detectors,Measurement,Feature extraction,Few-shot object detection,natural evolution,selective recombination module,mutational RPN,evolving training
AI 理解论文
溯源树
样例
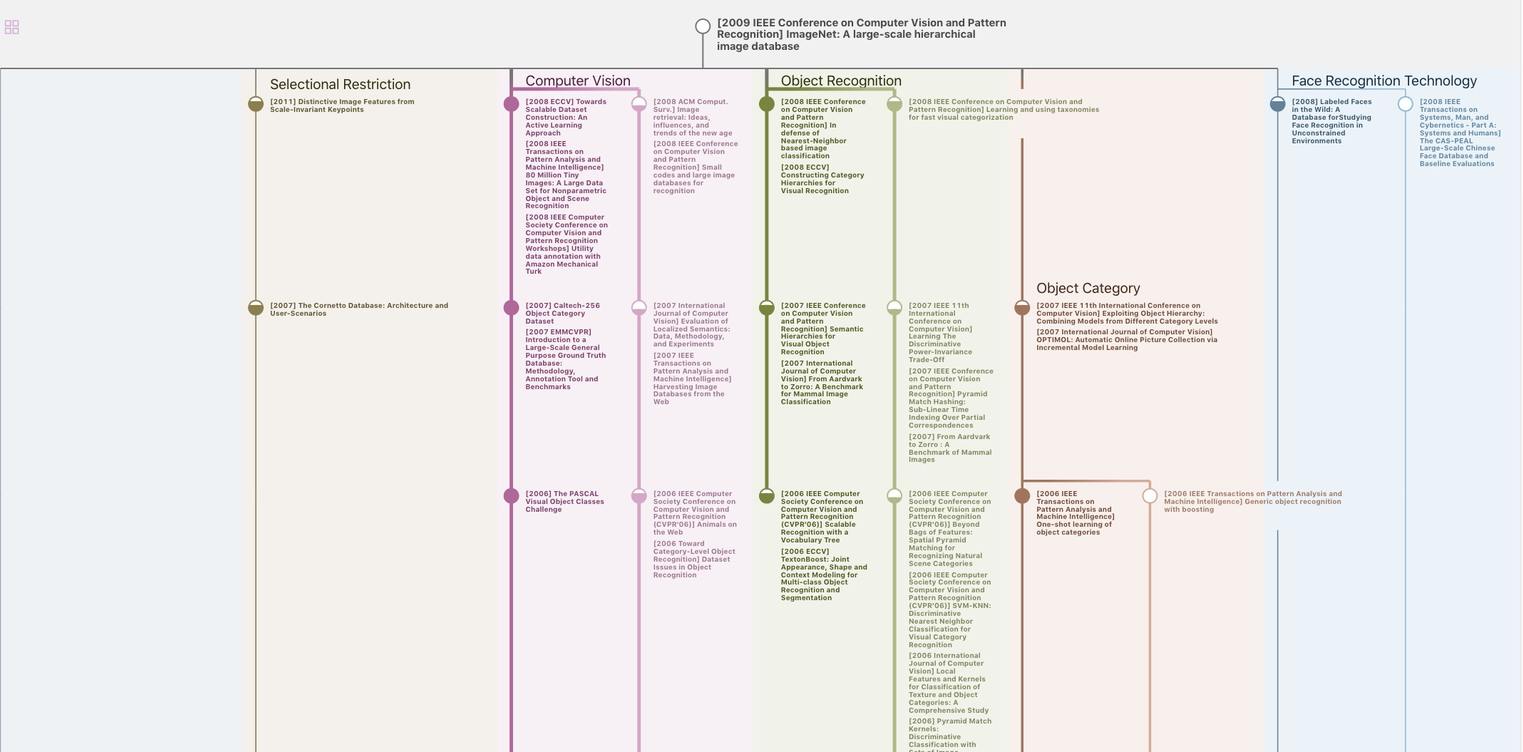
生成溯源树,研究论文发展脉络
Chat Paper
正在生成论文摘要