Evaluating Differential Privacy in Federated Continual Learning
2023 IEEE 98TH VEHICULAR TECHNOLOGY CONFERENCE, VTC2023-FALL(2023)
摘要
In recent years, the privacy-protecting framework Differential Privacy (DP) has achieved remarkable success and has been widely studied. However, there is a lack of work on DP in the area of Federated Continual Learning (FCL), which is a combination of Federated Learning (FL) and Continual Learning (CL). This paper presents a formal definition of DP-FCL and evaluates several DP-FCL methods based on Gaussian DP (GDP) and Individual DP (IDP). The experimental results indicate that gradient modification based CL strategies are not practical in DP-FCL. To the best of our knowledge, this is the first work to experimentally study DP-FCL, which can provide a reference for future research in this area.
更多查看译文
关键词
differential privacy,federated learning,continual learning,privacy,federated continual learning,deep learning
AI 理解论文
溯源树
样例
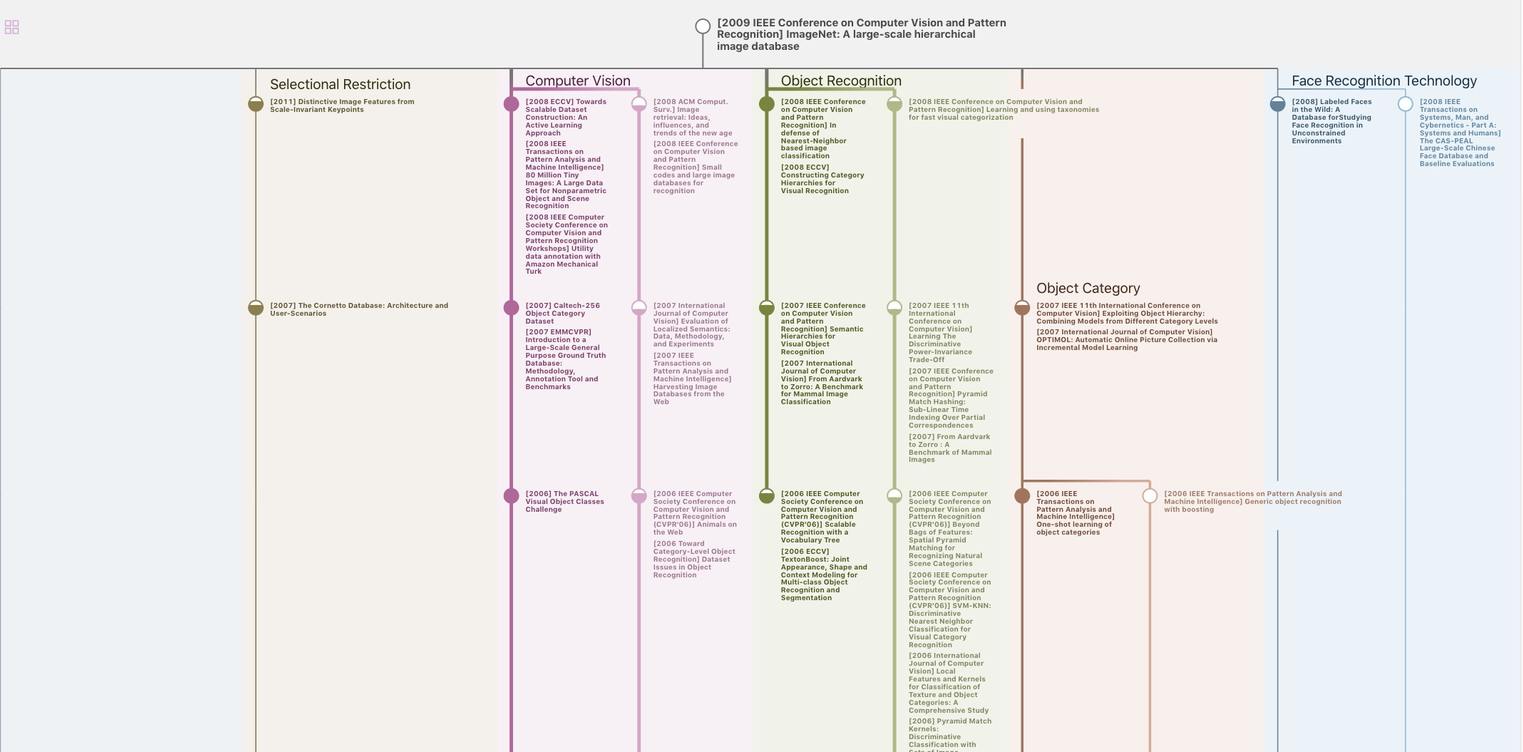
生成溯源树,研究论文发展脉络
Chat Paper
正在生成论文摘要