Cohort-based Power Scaling and Gradient Recovery for Over-The-Air Federated Learning
2023 IEEE 98TH VEHICULAR TECHNOLOGY CONFERENCE, VTC2023-FALL(2023)
摘要
Federated learning (FL) enables edge devices (EDs) to collaboratively train a single machine learning (ML) model maintained by an edge server (ES) without sharing their raw data contents, thereby facilitating distributed ML while protecting data privacy. In fact, FL can be implemented in wireless environments by means of the over-the-air (OTA) computation, which takes advantage of the waveform-superposition property of wireless signals to receive local gradients from EDs without the needs of increased bandwidth. Despite the existing works devoted to power control and client selection in OTA-FL systems, they mostly neglect how to construct cohorts (i.e., a subset of EDs that have similar channel coefficients) to elevate the quality of the aggregated local gradients. In this paper, we investigate the problem of gradient aggregation and recovery in OTA-FL systems. To cope with this problem, we propose the cohort-based power scaling and gradient recovery (CRAIC) algorithm, where we first construct cohorts based on uplink channel coefficients, and then we adjust the transmit powers of EDs and recover the aggregated local gradients in a cohort basis. Simulation results show that our proposed solution outperforms several comparison schemes, and we further evaluate how it performs under various parameter settings.
更多查看译文
关键词
Federated learning,over-the-air computation,gradient aggregation,gradient recovery,power scaling,cohort
AI 理解论文
溯源树
样例
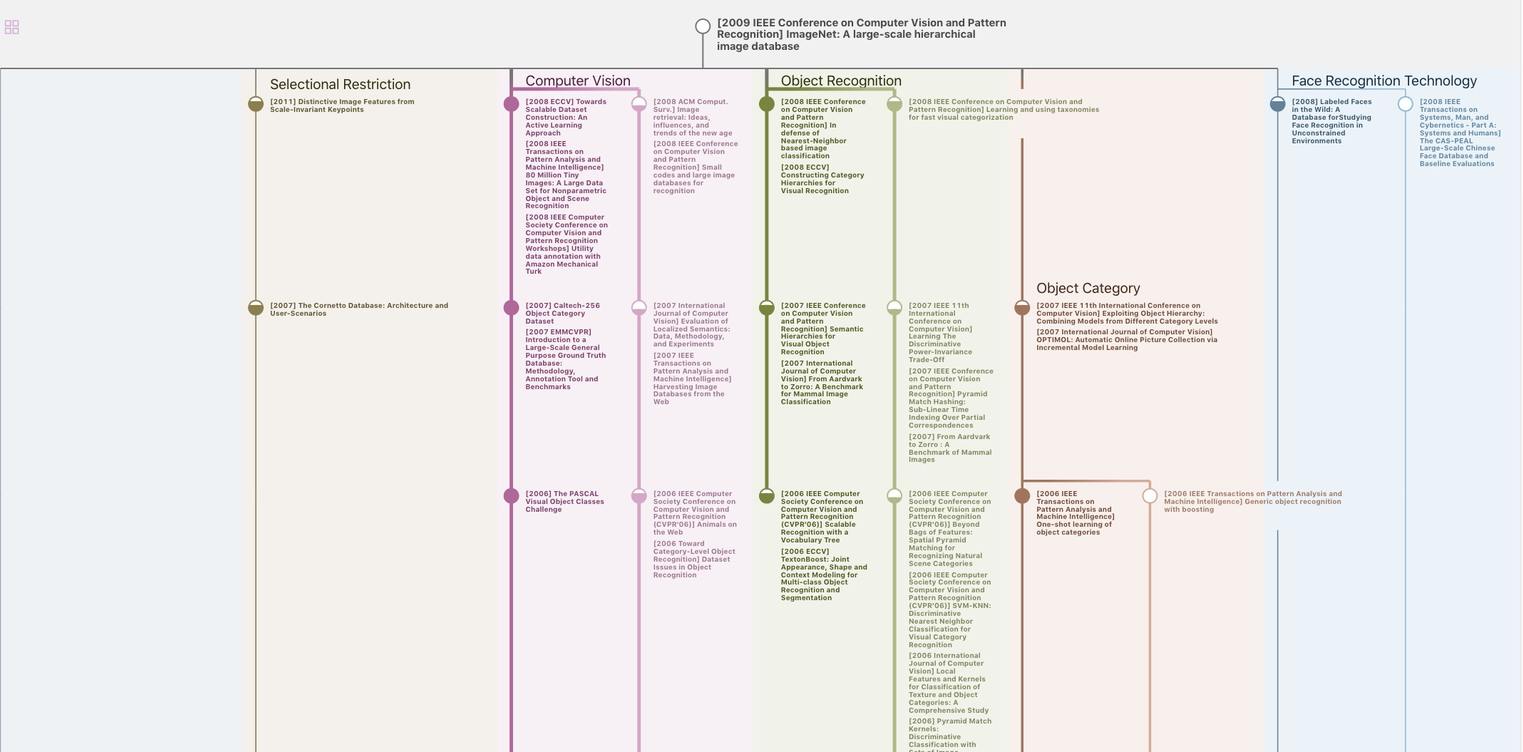
生成溯源树,研究论文发展脉络
Chat Paper
正在生成论文摘要