CycleGAN-Based Image to Image Translation for Realistic Surgical Training Phantoms
2023 45TH ANNUAL INTERNATIONAL CONFERENCE OF THE IEEE ENGINEERING IN MEDICINE & BIOLOGY SOCIETY, EMBC(2023)
摘要
Training in surgery is essential for surgeons to develop skill and dexterity. Physical training phantoms provide excellent haptic feedback and tissue properties for stitching and operating with authentic instruments and are easily available. However, they lack realistic traits and fail to reflect the complex environment of a surgical scene. Generative Adversarial Networks can be used for image-to-image translation, addressing the lack of realism in physical phantoms, by mapping patterns from the intraoperative domain onto the video stream captured during training with these surgical simulators. This work aims to achieve a successful I2I translation, from intraoperatory mitral valve surgery images onto a surgical simulator, using the CycleGAN model. Different experiments are performed - comparing the Mean Square Error Loss with the Binary Cross Entropy Loss; validating the Frechet Inception Distance as a training and image quality metric; and studying the impact of input variability on the model performance. Differences between MSE and BCE are modest, with MSE being marginally more robust. The FID score proves to be very useful in identifying the best training epochs for the CycleGAN I2I translation architecture. Carefully selecting the input images can have a great impact in the end results. Using less style variability and input images with good feature details and clearly defined characteristics enables the network to achieve better results.
更多查看译文
AI 理解论文
溯源树
样例
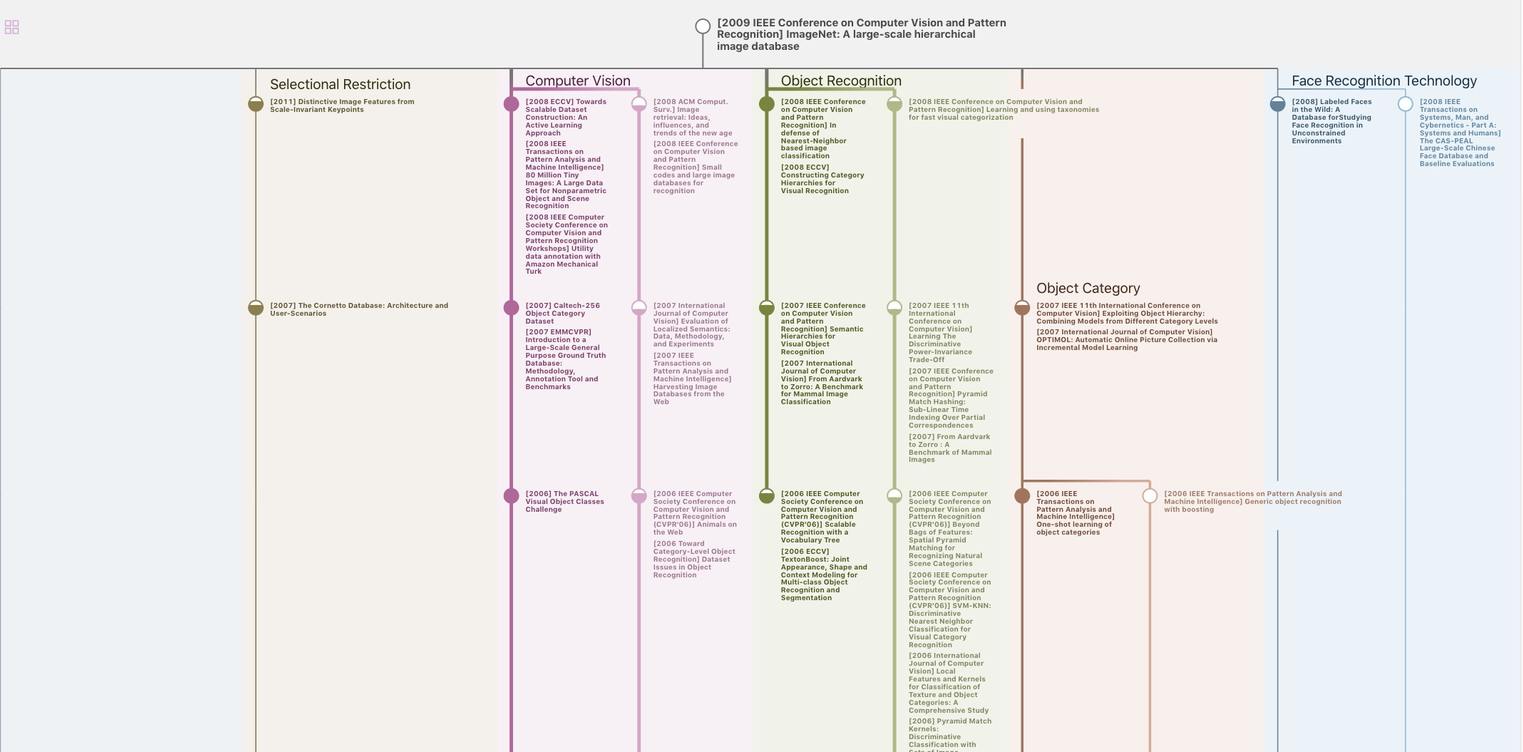
生成溯源树,研究论文发展脉络
Chat Paper
正在生成论文摘要