Reinforcement Learning Based Joint Resource Allocation and User Fairness Optimization in mmWave-NOMA HetNets
2023 31st International Conference on Electrical Engineering (ICEE)(2023)
摘要
In this paper, we propose heterogeneous network (HetNet) with mmWave and hybrid non-orthogonal multiple access (NOMA) and orthogonal multiple access (OMA) transmission, where uplink (UL) of macrocell users (MCUs) and downlink (DL) of small cell users (SCUs) share the same resource block (RB) to increase the network capacity. Also, an imperfect successive interference cancelation (I-SIC) decoding is considered due to hardware impairment of the real-world NOMA systems. We formulate the problem of joint power and resource block (RB) allocation to maximize rate-fairness and sum-rate by considering minimum quality of service (QoS) requirements. Reinforcement learning (RL) named Q-learning (QL) and deep Q-learning network (DQN) algorithms are employed to solve these problems. The results indicate that the proposed QL and DQN algorithms increase the fairness and sum-rate while low number of iterations is required.
更多查看译文
关键词
Heterogenous networks (HetNet),Non-orthogonal multiple access (NOMA),mmWave,Power allocation,Rate-fairness,Imperfect SIC,Reinforcement learning
AI 理解论文
溯源树
样例
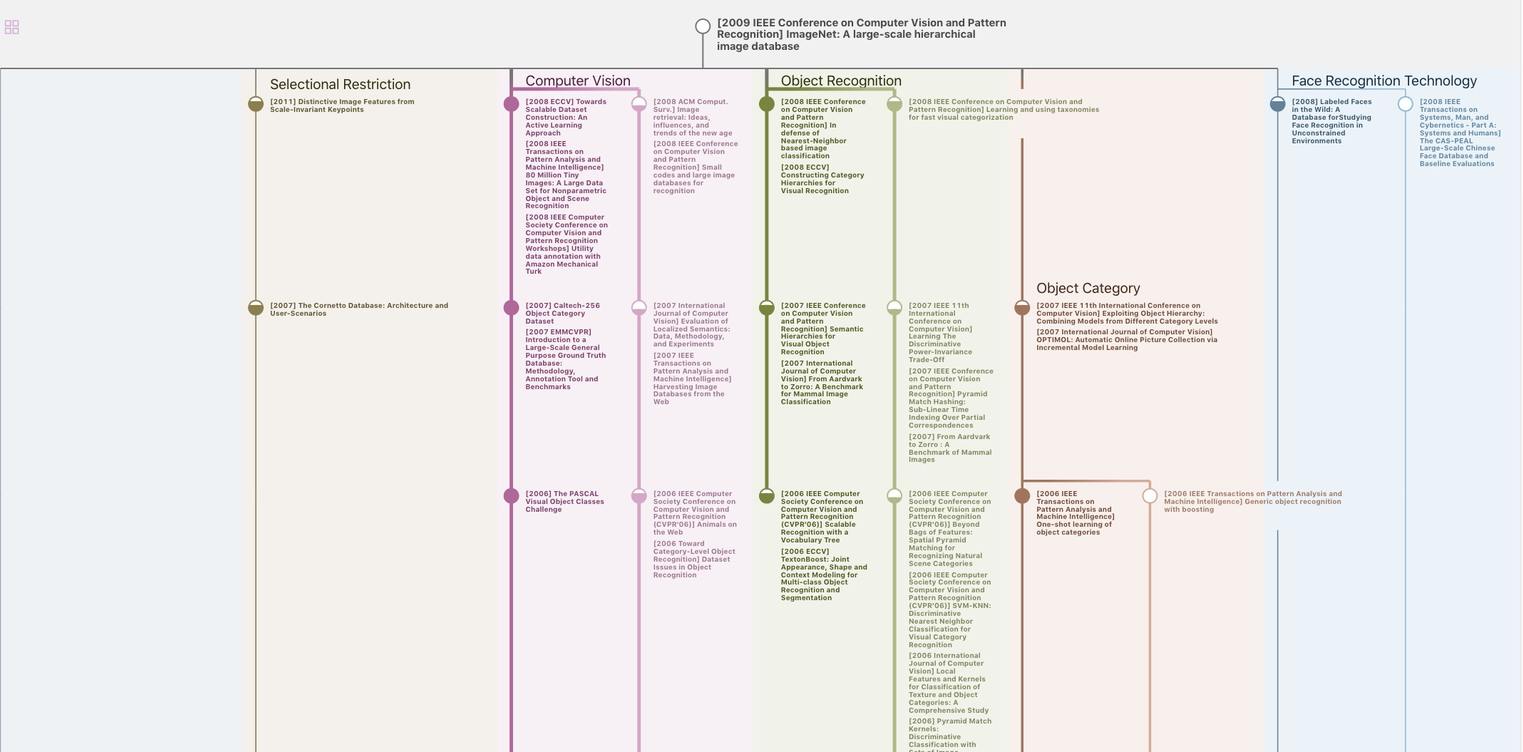
生成溯源树,研究论文发展脉络
Chat Paper
正在生成论文摘要