Domain and Patient Adversarial Multi-Task Learning for Arrhythmia Classification
2023 45TH ANNUAL INTERNATIONAL CONFERENCE OF THE IEEE ENGINEERING IN MEDICINE & BIOLOGY SOCIETY, EMBC(2023)
摘要
Manual screening of electrocardiograms (ECGs) for heart arrhythmias by clinicians is time-consuming and laborintensive. A machine learning model for the automated diagnosis of heart arrhythmia from ECG signals can facilitate improved diagnosis, greater accessibility and earlier intervention for patients. The potential of such models is limited however by the small size of clinical datasets available for training. Methods that can be trained with multiple datasets to classify heart arrhythmia are needed to overcome this problem. In this paper, we propose using adversarial multi-task learning (AMTL) to extract domain and patient invariant features from two electrocardiogram databases. We further investigated the influence of beat segmentation location and beat normalization on domain invariance. Our proposed methods were tested on the MIT-BIH Arrhythmia and the St Petersburg INCART 12-lead Arrhythmia Databases. The domain adversarial models achieved a higher accuracy and average F1 score than their counterparts without domain adversarial learning. In particular, the patient and domain adversarial model improved the F1 scores on the two tested databases from 70% and 74% to 77% each.
更多查看译文
AI 理解论文
溯源树
样例
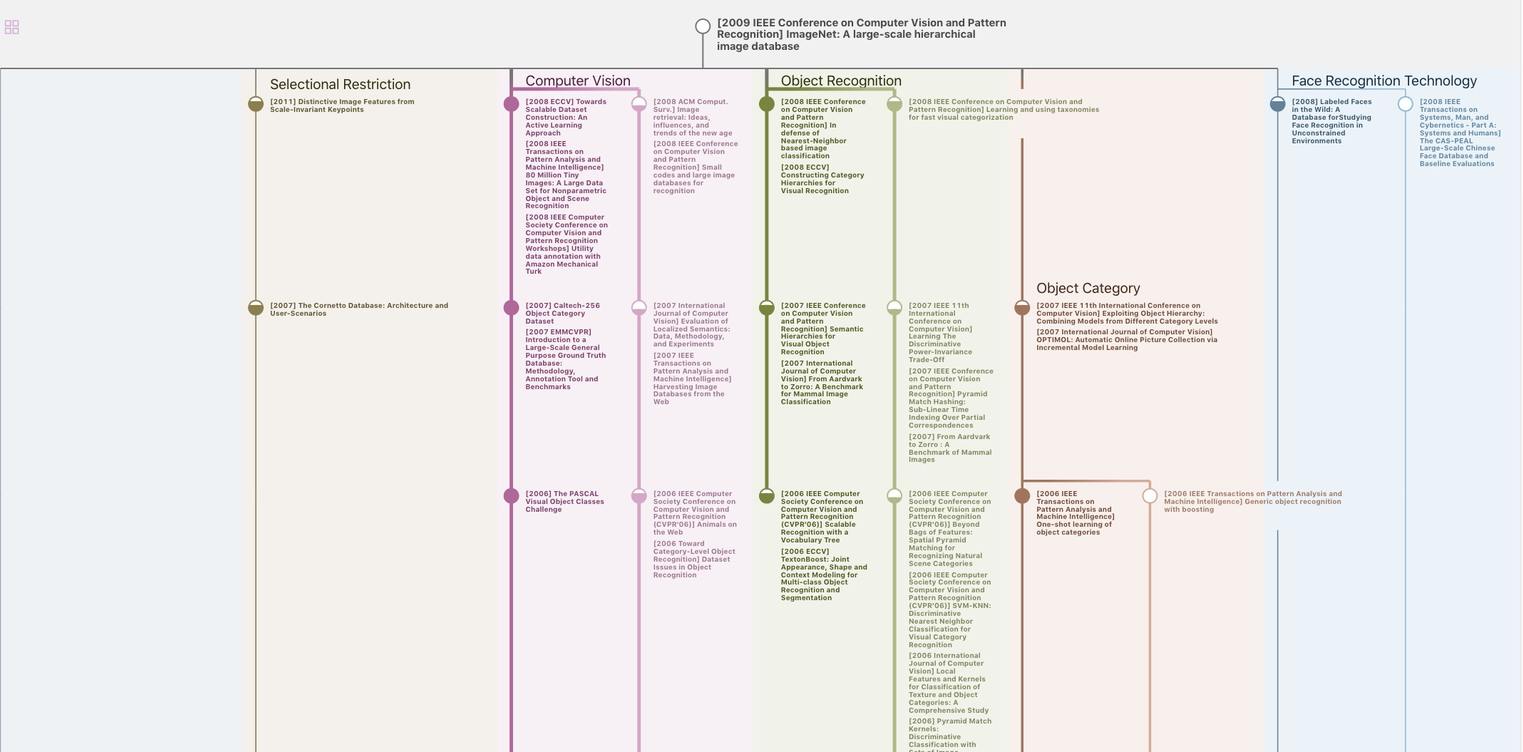
生成溯源树,研究论文发展脉络
Chat Paper
正在生成论文摘要