Tracking the Dynamic Neural Connectivity via Conjugate Gradient Optimization.
2023 45th Annual International Conference of the IEEE Engineering in Medicine & Biology Society (EMBC)(2023)
摘要
Neural connectivity describes how neuron populations coordinate and create cognitive and behavioral functions. Neural connectivity performs dynamics where its population spiking responses to stimuli or intention change over time. Brain-machine interface (BMI) provides a framework for studying dynamical neural connectivity. In BMI, point process is a powerful technique in analyzing the single neuronal tuning. And generalized linear mode (GLM) as an encoding model can incorporate the tuning in kinematics and the neural connectivity. Quantification and tracking of dynamic neural connectivity can contribute to the elucidation of the generation of brain functions in a computational way. However, most of the previous work focused on single neuronal adaptation to kinematics. When a neuron is significantly modulated by some other neurons in some tasks, the shape of the log likelihood function for single neuronal observations can be narrowed in some dimensions. And the existing gradient-based methods are not able to reach the optimum in a fast and adaptive searching way. In this work, to maximize the likelihood of observations and obtain the dynamic neural connectivity tuning parameters, we proposed a conjugate gradient-based encoding model (CGE). We illustrate CGE for likelihood function using the real experimental data under manual control and brain control. The results show that the proposed CGE has better performance in tracking the dynamic neural connectivity tuning parameters and modeling neural encoding.Clinical Relevance- Not directly related.
更多查看译文
AI 理解论文
溯源树
样例
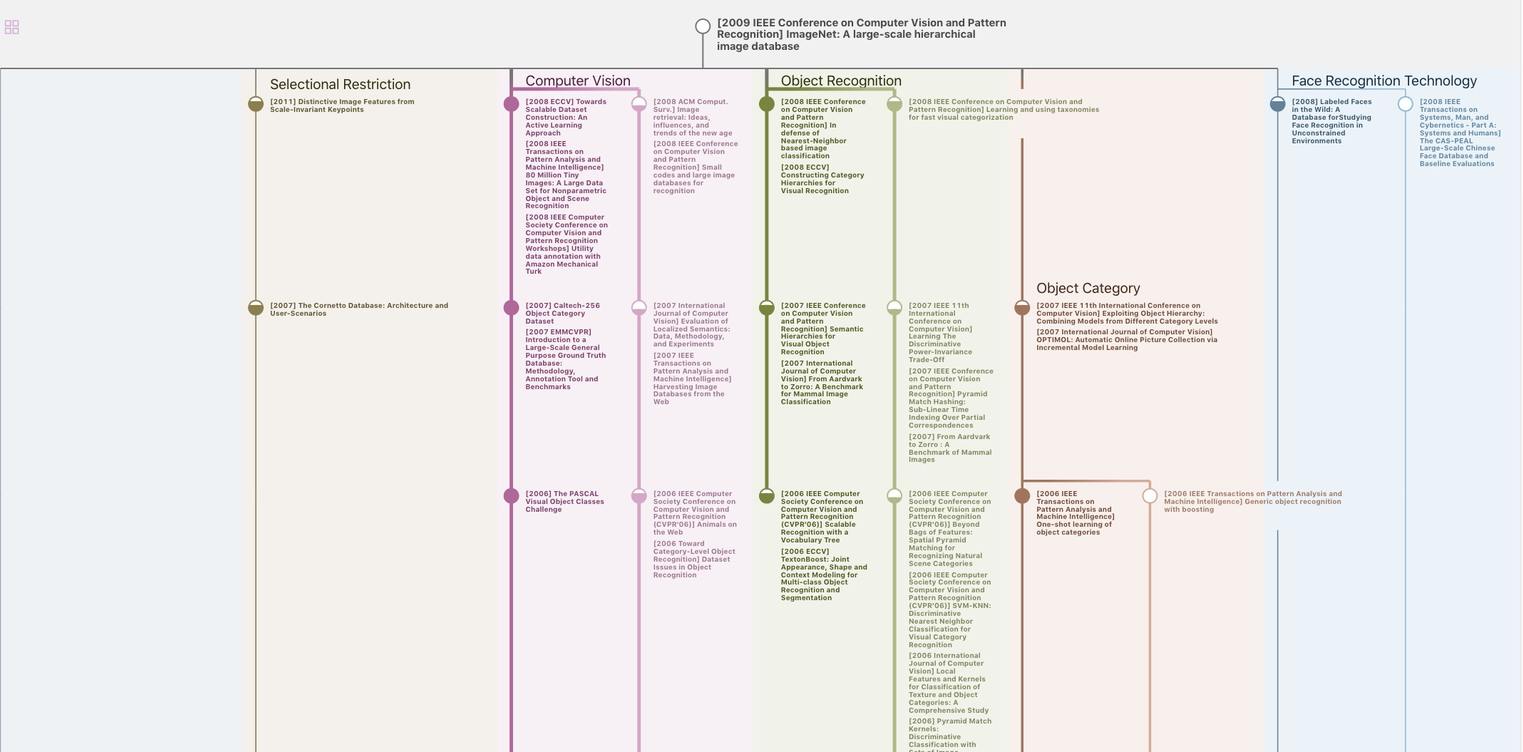
生成溯源树,研究论文发展脉络
Chat Paper
正在生成论文摘要