X-RIM: Extreme Recurrent Independent Mechanisms for Noise-resistant and Interpretable Stroke Risk Prediction
2023 45TH ANNUAL INTERNATIONAL CONFERENCE OF THE IEEE ENGINEERING IN MEDICINE & BIOLOGY SOCIETY, EMBC(2023)
摘要
Stroke is a leading cause of mortality and long-term disability worldwide. An accurate stroke risk prediction is crucial for its early detection and prevention. Using deep learning to exploit patients' time-series electronic health records (EHRs) has been shown as a promising and efficient solution for such a prediction. Although time-series data could be more informative than a single cross-section in time, real-world time-series EHRs usually have a significantly high missing rate due to irregular patient visits. This could undermine sequential data's benefits unless a proper deep-learning model design is adopted. Furthermore, deep models have long been challenged for their interpretability, which is especially crucial for medical applications. In this study, we propose an extreme design based on the concept of recurrent independent mechanisms (RIM), termed extreme RIM (X-RIM). With no need for imputation, X-RIM utilizes the information of each input feature's temporal records through independent recurrent modules. Experiments on real-world data from the National Taiwan University Hospital showed that, in terms of the area under the precision-recall curve (AUPRC), the area under the receiver-operating characteristics curve (AUROC), and Youden Index, X-RIM (AUPRC: 0.210; AUROC: 0.764; Youden: 0.373) outperformed the classic risk score CHA2DS2-VASc (AUPRC: 0.103; AUROC: 0.650; Youden: 0.223) and other benchmarks in stroke risk prediction. Additional experiments also indicate that individual feature contributions to a prediction could be evaluated intuitively under X-RIM's independent structure to enhance interpretability.
更多查看译文
AI 理解论文
溯源树
样例
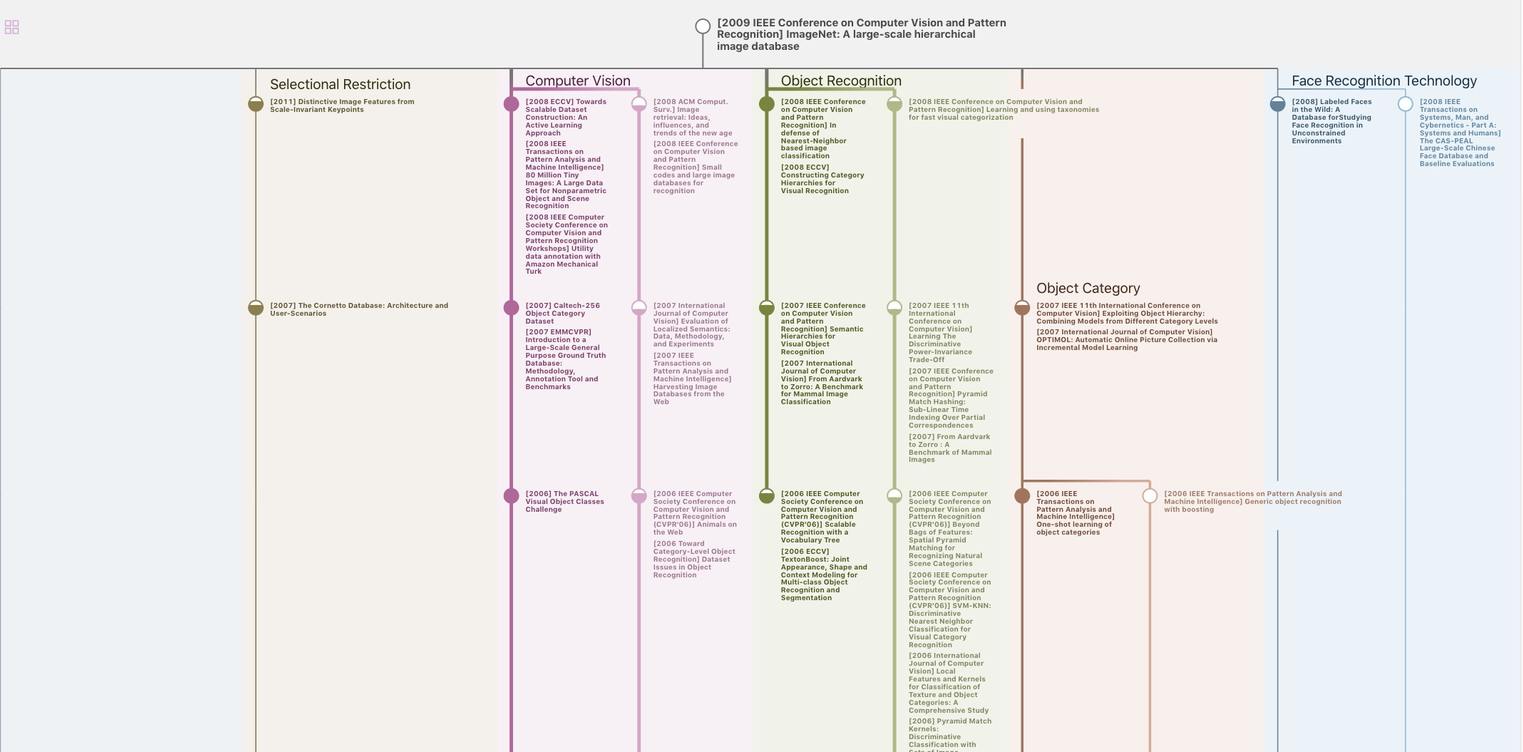
生成溯源树,研究论文发展脉络
Chat Paper
正在生成论文摘要