A Kernel Reinforcement Learning Decoding Framework Integrating Neural and Feedback Signals for Brain Control
2023 45TH ANNUAL INTERNATIONAL CONFERENCE OF THE IEEE ENGINEERING IN MEDICINE & BIOLOGY SOCIETY, EMBC(2023)
摘要
Brain-Machine Interfaces (BMIs) have the potential to allow subjects to brain control (BC) external devices, where their brain signals could be translated to the action of the neuro-prosthesis by reinforcement learning (RL) based decoder. During the BC task, feedback cues are provided to guide subject's learning. Subjects will adapt the neural signals according to the feedback cues. Concurrently, the RL decoding parameters are adjusted when the subject explores the BC task through trial and error, leading to a co-adaptive process between the subject and the decoder. However, when subjects receive the feedback cues and enhance their learning, the decoder does not actively utilize the feedback cues. If the RL decoder could integrate both neural signals and feedback cues, the training efficiency of the BC task would increase. A major challenge is the different temporal scales of neural signals and feedback cues, making it difficult to integrate them into a single decoder. In this paper, we propose a novel kernel RL decoding method as the first attempt to combine two signals with different temporal scales for RL decoding. The neural signals and the feedback cues comprise the decoding input, which is then projected into individual Reproducing Kernel Hilbert Spaces (RKHSs) respectively. These two RKHSs form a joint feature space, where the action of the neuro-prosthesis could be decoded linearly. We evaluate the proposed method on a simulated brain control cursor-reaching task. Our proposed method is compared with the kernel RL that only uses neural signals as the input. The proposed method has a faster learning speed and better decoding accuracy. The results demonstrate that our proposed method has successfully integrated the information of the feedback cue and facilitates the training procedure for the BC task.
更多查看译文
AI 理解论文
溯源树
样例
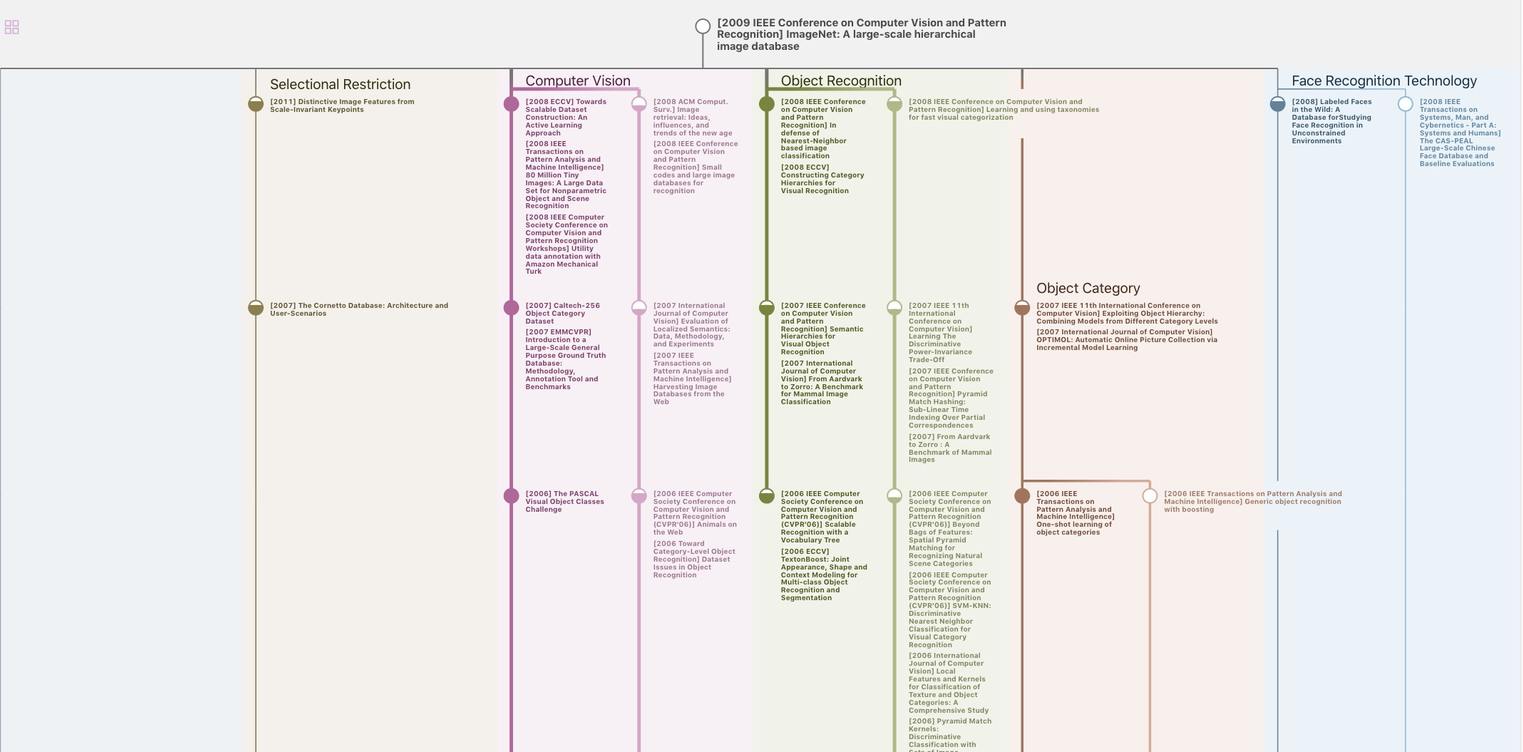
生成溯源树,研究论文发展脉络
Chat Paper
正在生成论文摘要