Applying Neural Manifold Constraint on Point Process Model for Neural Spike Prediction
2023 45TH ANNUAL INTERNATIONAL CONFERENCE OF THE IEEE ENGINEERING IN MEDICINE & BIOLOGY SOCIETY, EMBC(2023)
摘要
Neural prostheses can compensate for functional losses caused by blocked neural pathways by modeling neural activities among cortical areas. Existing methods generally utilize point process models to predict neural spikes from one area to another, and optimize the model by maximizing the log-likelihood between model predictions and recorded activities of individual neurons. However, single-neuron recordings can be distorted, while neuron population activity tends to reside within a stable subspace called the neural manifold, which reflects the connectivity and correlation among output neurons. This paper proposes a neural manifold constraint to modify the loss function for model training. The constraint term minimizes the distance from model predictions to the empirical manifold to amend the model predictions from distorted recordings. We test our methods on synthetic data with distortion on output spike trains and evaluate the similarity between model predictions and original output spike trains by the Kolmogorov-Smirnov test. The results show that the models trained with constraint have higher goodness-of-fit than those trained without constraint, which indicates the potential better approach for neural prostheses in noisy environments.
更多查看译文
AI 理解论文
溯源树
样例
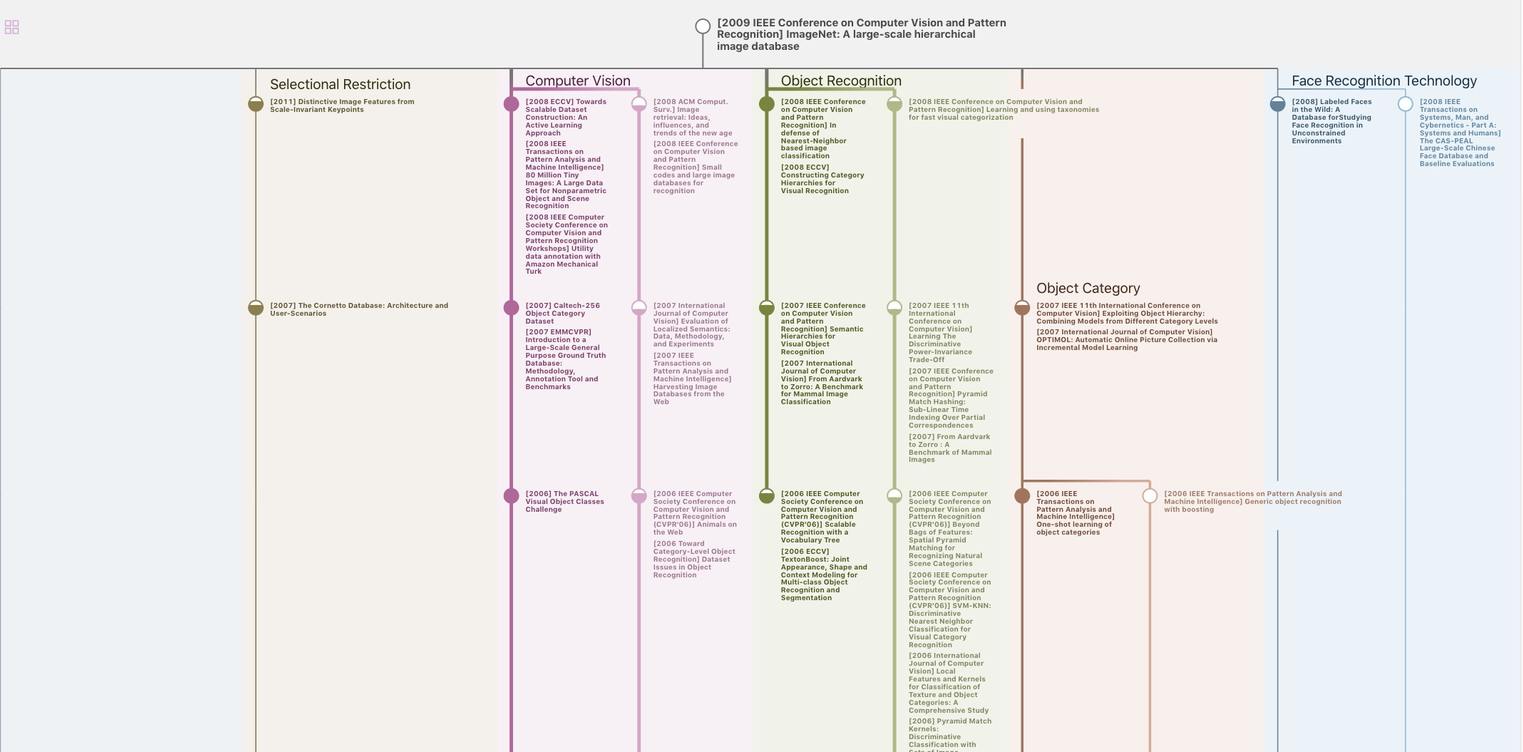
生成溯源树,研究论文发展脉络
Chat Paper
正在生成论文摘要