State-space Model Based Inverse Reinforcement Learning for Reward Function Estimation in Brain-machine Interfaces
2023 45TH ANNUAL INTERNATIONAL CONFERENCE OF THE IEEE ENGINEERING IN MEDICINE & BIOLOGY SOCIETY, EMBC(2023)
摘要
The use of reinforcement learning (RL) in brain machine interfaces (BMIs) is considered to be a promising method for neural decoding. One key component of RL-based BMIs is the reward signal, which is used to guide decoders to update the parameters. However, designing effective and efficient rewards can be challenging, especially for complex tasks. Inverse reinforcement learning (IRL) is a method that has been proposed to estimate the internal reward function from subjects' neural activity. However, multi-channel neural activity, which may encode many sources of information, builds a large dimensions of state-action space, making it difficult to directly apply IRL methods in BMI systems. In this paper, we propose a state-space model based inverse Q-learning (SSM-IQL) method to improve the performance of the existing IRL method. The state-space model is designed to extract hidden brain state from high-dimensional neural activity. We tested the proposed method on real data collected from rats during a two-lever discrimination task. Preliminary results show that SSM-IQL provides a more accurate and stable estimation of the internal reward function than the traditional IQL algorithm. This suggests that the use of state-space model in IRL method has potential to improve the design of RL-based BMIs.
更多查看译文
关键词
Brain machine interface,inverse reinforcement learning,state-space model
AI 理解论文
溯源树
样例
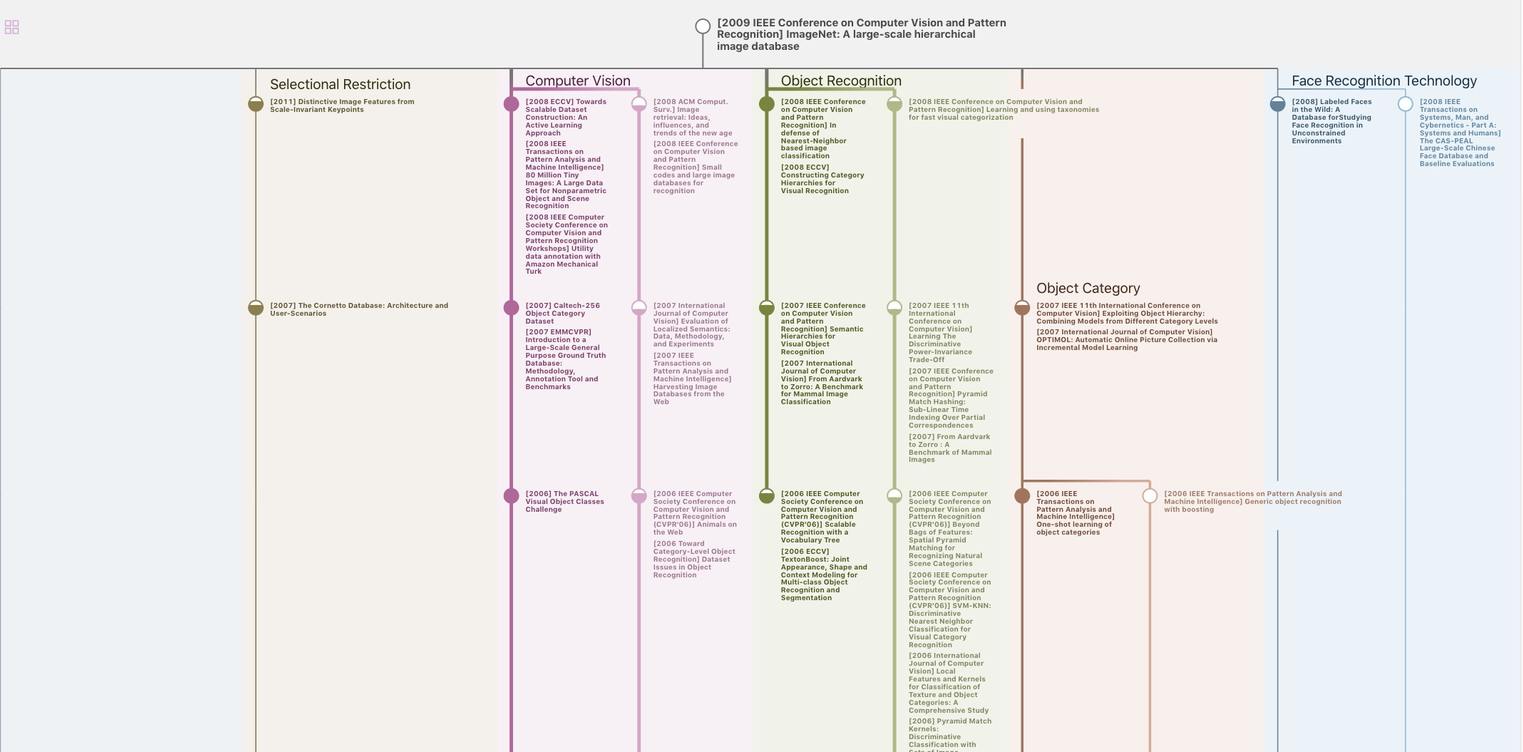
生成溯源树,研究论文发展脉络
Chat Paper
正在生成论文摘要