Assessing the Generalizability of a Deep Learning-based Automated Atrial Fibrillation Algorithm
2023 45TH ANNUAL INTERNATIONAL CONFERENCE OF THE IEEE ENGINEERING IN MEDICINE & BIOLOGY SOCIETY, EMBC(2023)
摘要
Automated detection of atrial fibrillation (AF) from electrocardiogram (ECG) traces remains a challenging task and is crucial for telemonitoring of patients after stroke. This study aimed to quantify the generalizability of a deep learning (DL)-based automated ECG classification algorithm. We first developed a novel hybrid DL (HDL) model using the PhysioNet/CinC Challenge 2017 (CinC2017) dataset (publicly available) that can classify the ECG recordings as one of four classes: normal sinus rhythm (NSR), AF, other rhythms (OR), and too noisy (TN) recordings. The (pre)trained HDL was then used to classify 636 ECG samples collected by our research team using a handheld ECG device, CONTEC PM10 Portable ECG Monitor, from 102 (age: 68 +/- 15 years, 74 male) outpatients of the Eastern Heart Clinic and inpatients in the Cardiology ward of Prince of Wales Hospital, Sydney, Australia. The proposed HDL model achieved average test F-1-score of 0.892 for NSR, AF, and OR, relative to the reference values, on the CinC2017 dataset. The HDL model also achieved an average F-1-score of 0.722 (AF: 0.905, NSR: 0.791, OR: 0.471 and TN: 0.342) on the dataset created by our research team. After retraining the HDL model on this dataset using a 5-fold cross validation method, the average F-1-score increased to 0.961. We finally conclude that the generalizability of the HDL-based algorithm developed for AF detection from short-term single-lead ECG traces is acceptable. However, the accuracy of the pre-trained DL model was significantly improved by retraining the model parameters on the new dataset of ECG traces.
更多查看译文
AI 理解论文
溯源树
样例
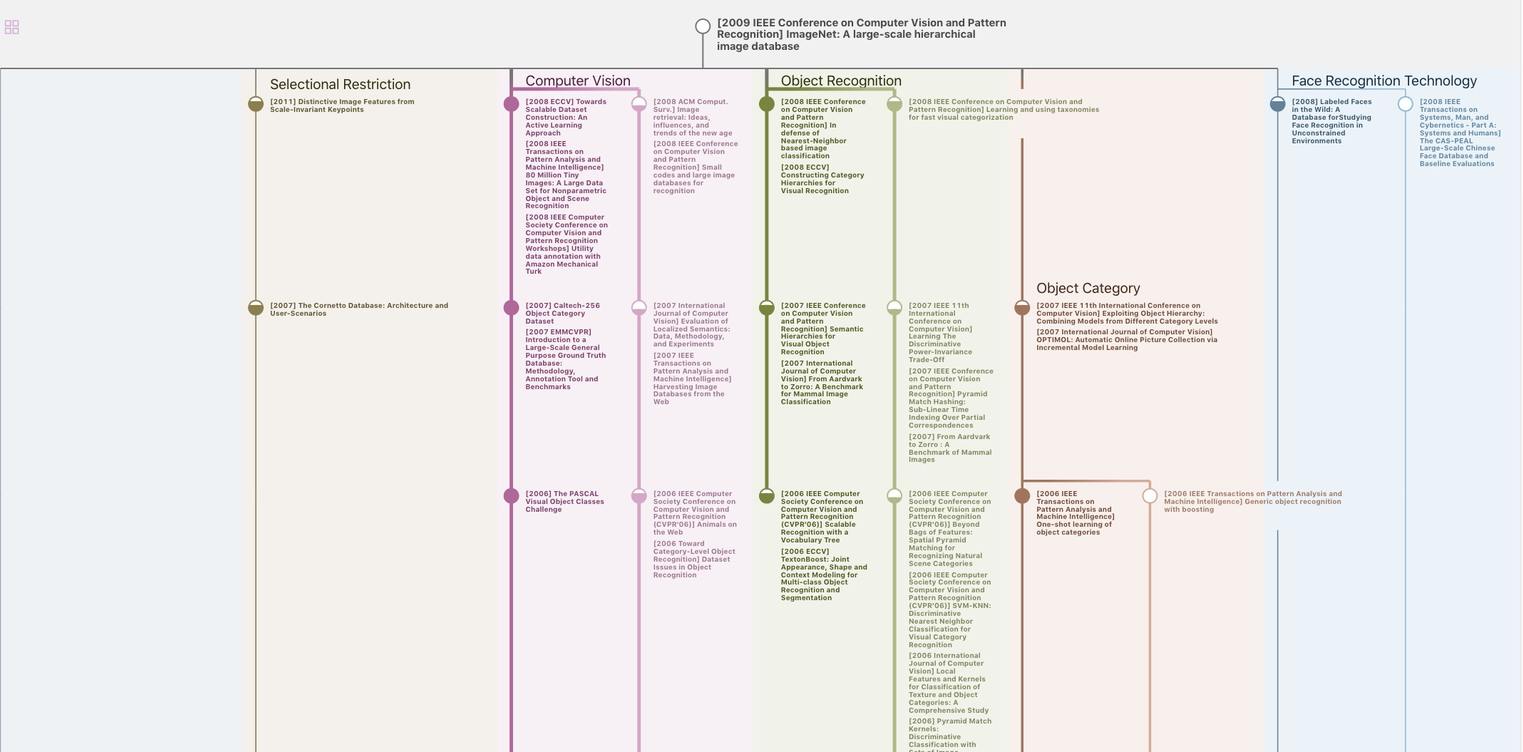
生成溯源树,研究论文发展脉络
Chat Paper
正在生成论文摘要