The Effect of Fetal Heart Rate Segment Selection on Deep Learning Models for Fetal Compromise Detection.
2023 45th Annual International Conference of the IEEE Engineering in Medicine & Biology Society (EMBC)(2023)
摘要
Monitoring the fetal heart rate (FHR) is common practice in obstetric care to assess the risk of fetal compromise. Unfortunately, human interpretation of FHR recordings is subject to inter-observer variability with high false positive rates. To improve the performance of fetal compromise detection, deep learning methods have been proposed to automatically interpret FHR recordings. However, existing deep learning methods typically analyse a fixed-length segment of the FHR recording after removing signal gaps, where the influence of this segment selection process has not been comprehensively assessed. In this work, we develop a novel input length invariant deep learning model to determine the effect of FHR segment selection for detecting fetal compromise. Using this model, we perform five times repeated five-fold cross-validation on an open-access database of 552 FHR recordings and assess model performance for FHR segment lengths between 15 and 60 minutes. We show that the performance after removing signal gaps improves with increasing segment length from 15 minutes (AUC = 0.50) to 60 minutes (AUC = 0.74). Additionally, we demonstrate that using FHR segments without removing signal gaps achieves superior performance across signal lengths from 15 minutes (AUC = 0.68) to 60 minutes (AUC = 0.76). These results show that future works should carefully consider FHR segment selection and that removing signal gaps might contribute to the loss of valuable information.
更多查看译文
AI 理解论文
溯源树
样例
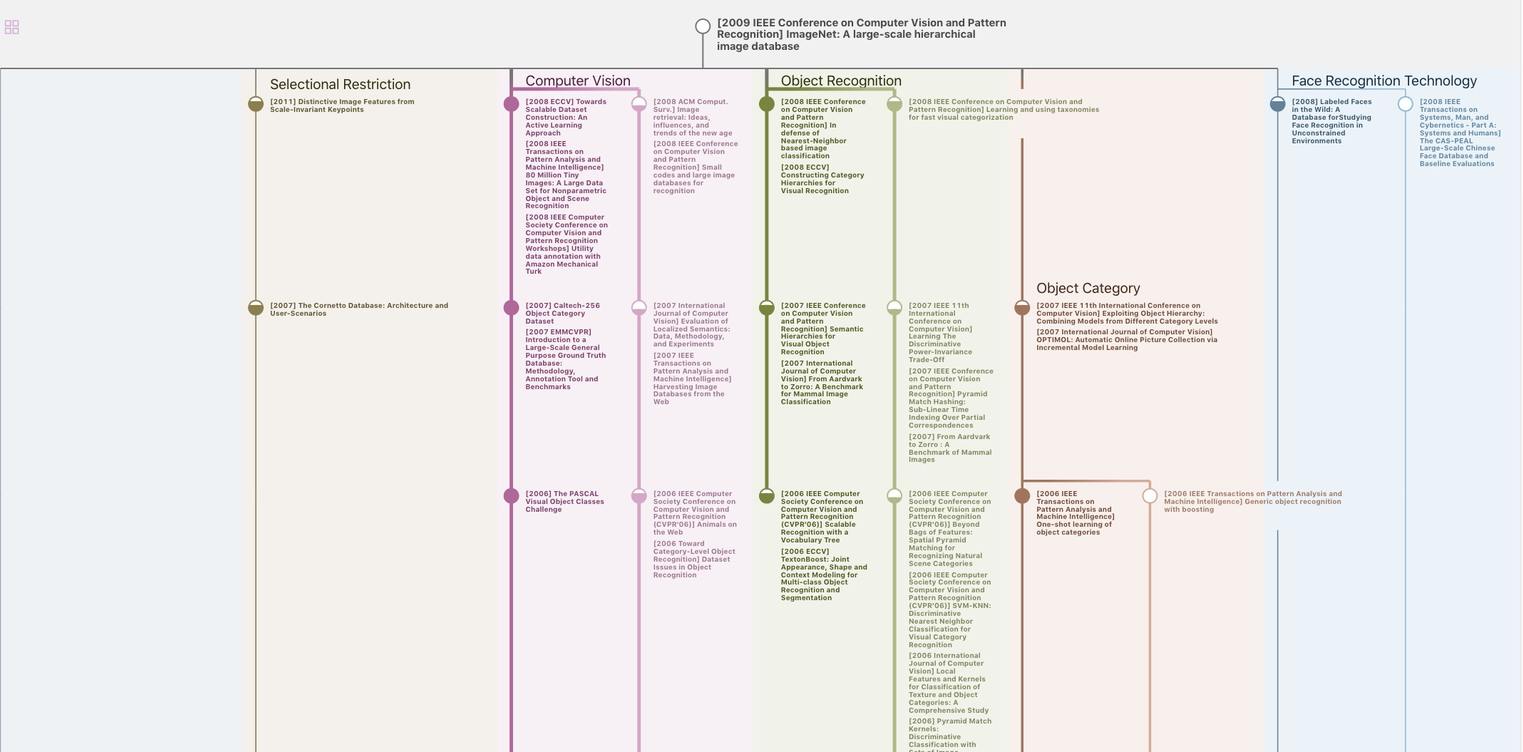
生成溯源树,研究论文发展脉络
Chat Paper
正在生成论文摘要