Hand Force Estimation from Acoustic Myography Using Deep Wavelet Scattering Transform and Long Short-Term Memory
2023 45TH ANNUAL INTERNATIONAL CONFERENCE OF THE IEEE ENGINEERING IN MEDICINE & BIOLOGY SOCIETY, EMBC(2023)
摘要
The ability to estimate user intention from surface electromyogram (sEMG) signals is a crucial aspect in the design of powered prosthetics. Recently, researchers have been using regression techniques to connect the user's intent, as expressed through sEMG signals, to the force applied at the fingertips in order to achieve a natural and accurate form of control. However, there are still challenges associated with processing sEMG signals that need to be overcome to allow for widespread and clinical implementation of upper limb prostheses. As a result, alternative modalities functioning as promising control signals have been proposed as source of control input rather than the sEMG, such as Acoustic Myography (AMG). In this study, six high sensitivity array microphones were used to acquire AMG signals, with custom-built 3D printed microphone housing. To tackle the challenge of extracting the relevant information from AMG signals, the Wavelet Scattering Transform (WST) was utilized. alongside a Long Short-Term Memory (LSTM) neural network model for predicting the force from the AMG. The subjects were asked to use a hand dynamometer to measure the changes in force and correlate that to the force predicted by using the AMG features. Seven subjects were recruited for data collection in this study, using hardware designed by the research team. the performance results showed that the WST-LSTM model can be robustly utilized across varying window sizes and testing schemes, to achieve average NRMSE results of approximately 8%. These pioneering results suggest that AMG signals can be utilized to reliably estimate the force levels that the muscles are applying.
更多查看译文
AI 理解论文
溯源树
样例
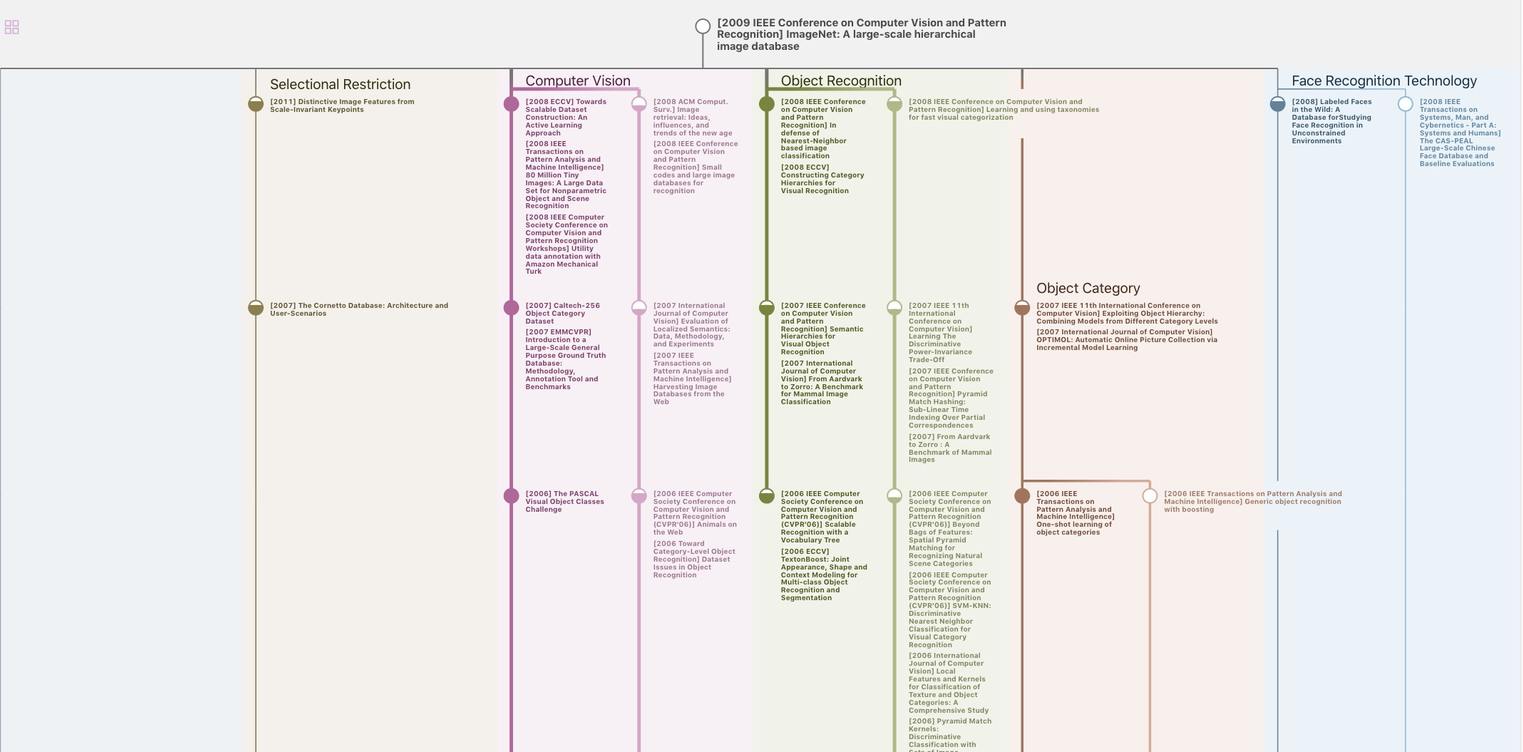
生成溯源树,研究论文发展脉络
Chat Paper
正在生成论文摘要