Data-Augmentation-Based Federated Learning
IEEE INTERNET OF THINGS JOURNAL(2023)
摘要
With the rapid growth of the number of devices generating and collecting data, dispersion becomes an important feature of data in Internet of Things. Federated learning (FL) provides a feasible way to mine information in such distributed data. It involves training machine learning models over multiple distributed participants without raw data transmission. However, due to the data heterogeneity among participants, the performance of the FL model degrades dramatically. Currently, improved methods mainly reduce data heterogeneity from the perspective of modifying the process of model training, which usually have problems, such as high-resource consumption or the need for auxiliary data. In this article, we enhance FL model from another perspective, focusing on data rather than model training. We reduce data heterogeneity by enhancing the trained local data to improve FL performance. Specifically, we propose an FL method based on data augmentation (abbreviated as FedM-UNE), implementing the classic data augmentation method MixUp in federated scenarios without transferring raw data. Furthermore, in order to adapt this method to regression tasks, we first modify MixUp by bilateral neighborhood expansion (MixUp-BNE), and then propose a federated data augmentation method named FedM-BNE based on it. Compared with the conventional FL method, both FedM-UNE and FedM-BNE increase negligible overhead. To demonstrate the effectiveness, we conduct exhaustive experiments on six data sets employing a variety of loss functions. The results indicate that FedM-UNE and FedM-BNE consistently improve the performance of the FL model. Moreover, our methods are compatible with existing FL enhancements, which yield further improvements in performance.
更多查看译文
关键词
Data augmentation,Task analysis,Distributed databases,data heterogeneity,edge computing,federated learning (FL),neighborhood expansion
AI 理解论文
溯源树
样例
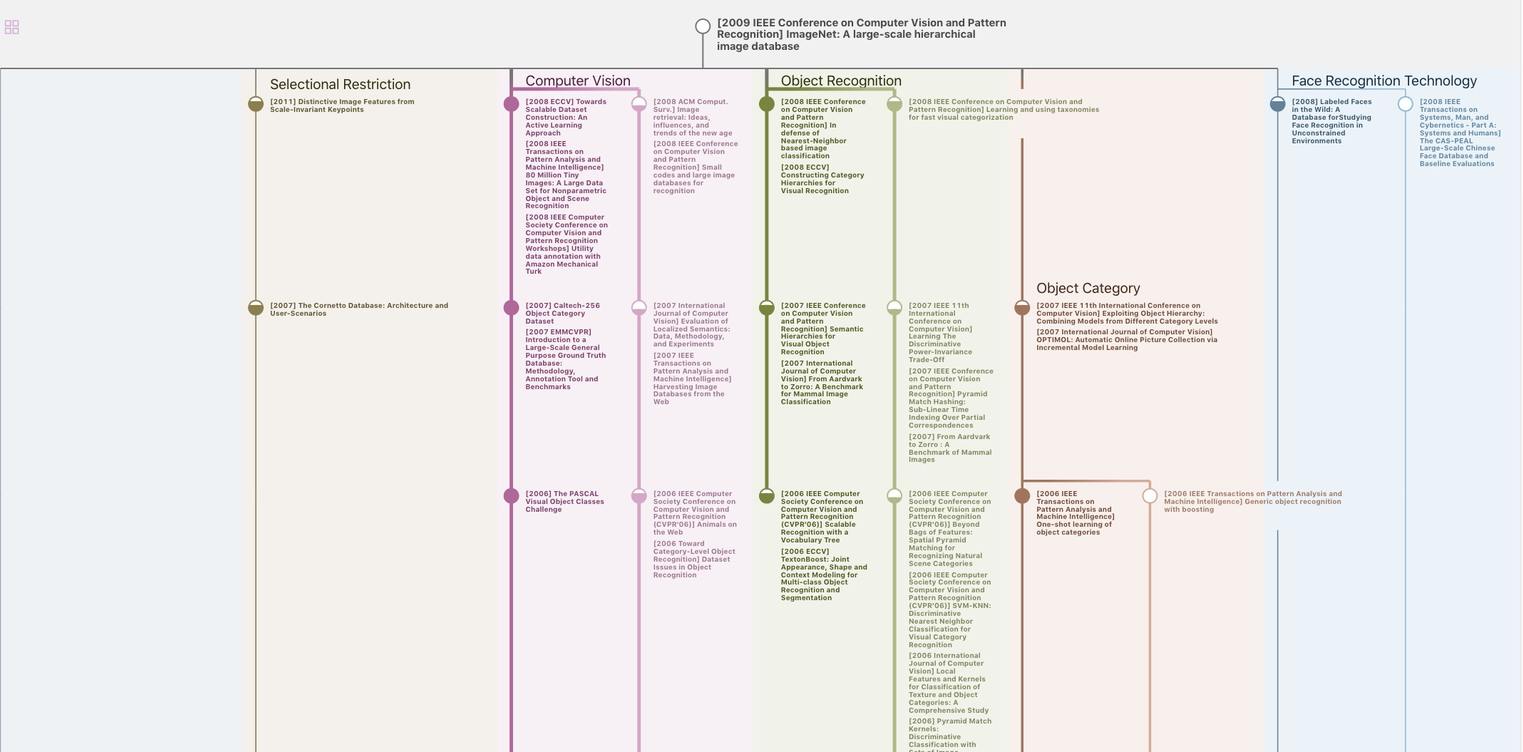
生成溯源树,研究论文发展脉络
Chat Paper
正在生成论文摘要