RA-HGNN: Attribute completion of heterogeneous graph neural networks based on residual attention mechanism
EXPERT SYSTEMS WITH APPLICATIONS(2024)
摘要
Heterogeneous graphs, which are also called heterogeneous information networks, analyze the different types of nodes in an information network and the different types of links between them to accurately tell the difference between different semantics. In recent years, there have been several GNN-based models to process heterogeneous graph data and achieve good performance. The model faces the challenge of first considering how to deal with the challenges posed by embedding different types of nodes in a heterogeneous graph; secondly, analyzing the node attribute information, which requires satisfying all nodes with attributes, which is not easy to achieve due to the existence of individual nodes and their neighbors that do not carry attributes. Previous network structures have added attributes to nodes by handcrafted methods, thus neglecting the overall learnability of the model, which in turn leads to poor performance. This paper analyzes the reasons for this phenomenon and aims to design a learning-competent heterogeneous graph neural networks(HGNN) framework. The understanding in this study embeds different types of nodes into the same feature space for node embedding, using the topological embedding of heterogeneous graphs as a guide to complete the process of complementing non-attributed nodes through learnable ways in the model and the use of residual attention mechanisms to handle attributes between nodes. Therefore, this paper proposes a general framework for Attribute Completion of Heterogeneous Graph Neural Network Based on Residual Attention Mechanism (RA-HGNN) , and combines it with other GNN models to enable end-to-end execution of the entire model. Experimental verification was completed on real-world data sets to prove the feasibility of the model, and the experimental results showed state-of-the-art performance.
更多查看译文
关键词
Graph neural networks,Heterogeneous information networks,Residual attention mechanism,Attribute completion
AI 理解论文
溯源树
样例
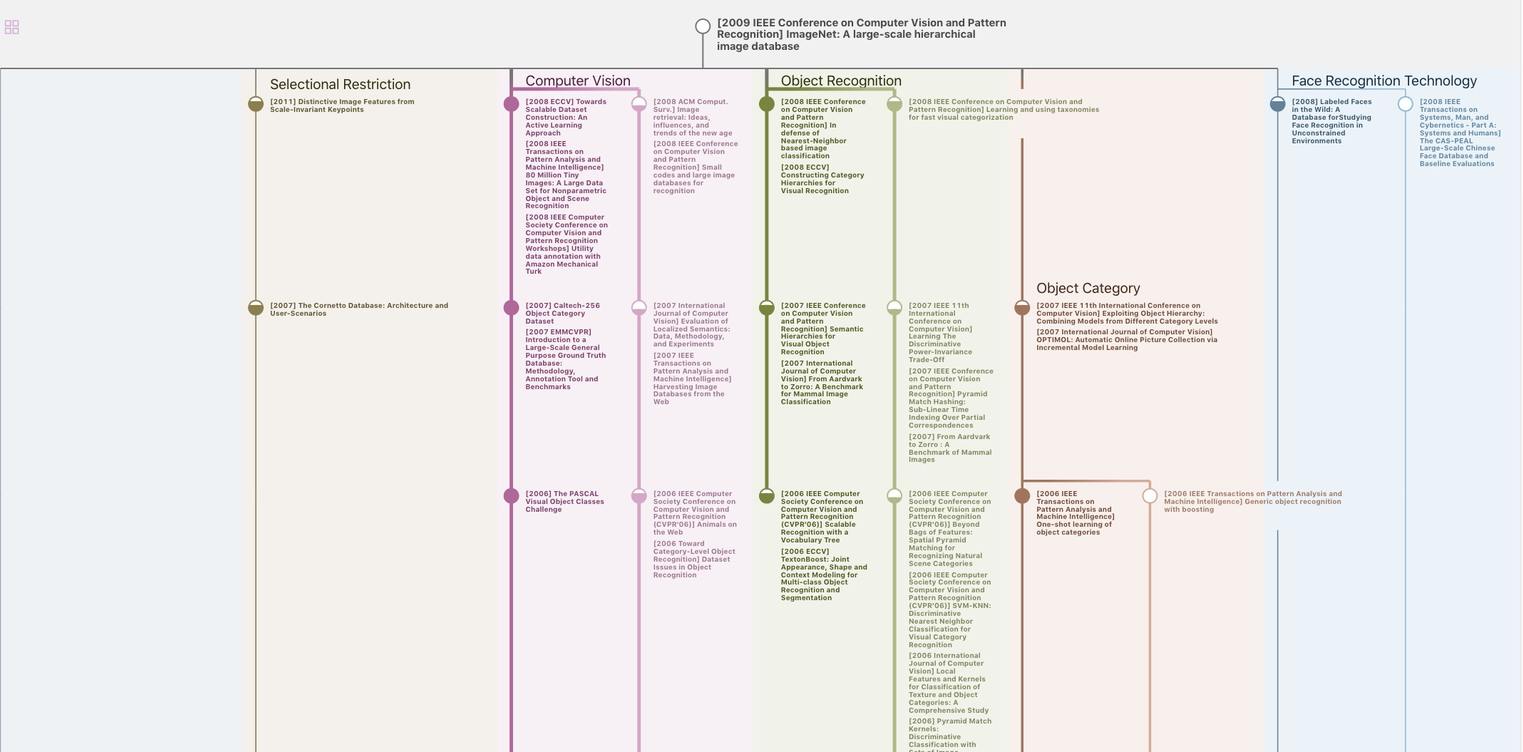
生成溯源树,研究论文发展脉络
Chat Paper
正在生成论文摘要