Development and validation of a deep learning model to predict axial length from ultra-wide field images
Eye(2023)
摘要
Background To validate the feasibility of building a deep learning model to predict axial length (AL) for moderate to high myopic patients from ultra-wide field (UWF) images. Methods This study included 6174 UWF images from 3134 myopic patients during 2014 to 2020 in Eye and ENT Hospital of Fudan University. Of 6174 images, 4939 were used for training, 617 for validation, and 618 for testing. The coefficient of determination (R 2 ), mean absolute error (MAE), and mean squared error (MSE) were used for model performance evaluation. Results The model predicted AL with high accuracy. Evaluating performance of R 2 , MSE and MAE were 0.579, 1.419 and 0.9043, respectively. Prediction bias of 64.88% of the tests was under 1-mm error, 76.90% of tests was within the range of 5% error and 97.57% within 10% error. The prediction bias had a strong negative correlation with true AL values and showed significant difference between male and female ( P < 0.001). Generated heatmaps demonstrated that the model focused on posterior atrophy changes in pathological fundus and peri-optic zone in normal fundus. In sex-specific models, R 2 , MSE, and MAE results of the female AL model were 0.411, 1.357, and 0.911 in female dataset and 0.343, 2.428, and 1.264 in male dataset. The corresponding metrics of male AL models were 0.216, 2.900, and 1.352 in male dataset and 0.083, 2.112, and 1.154 in female dataset. Conclusions It is feasible to utilize deep learning models to predict AL for moderate to high myopic patients with UWF images.
更多查看译文
AI 理解论文
溯源树
样例
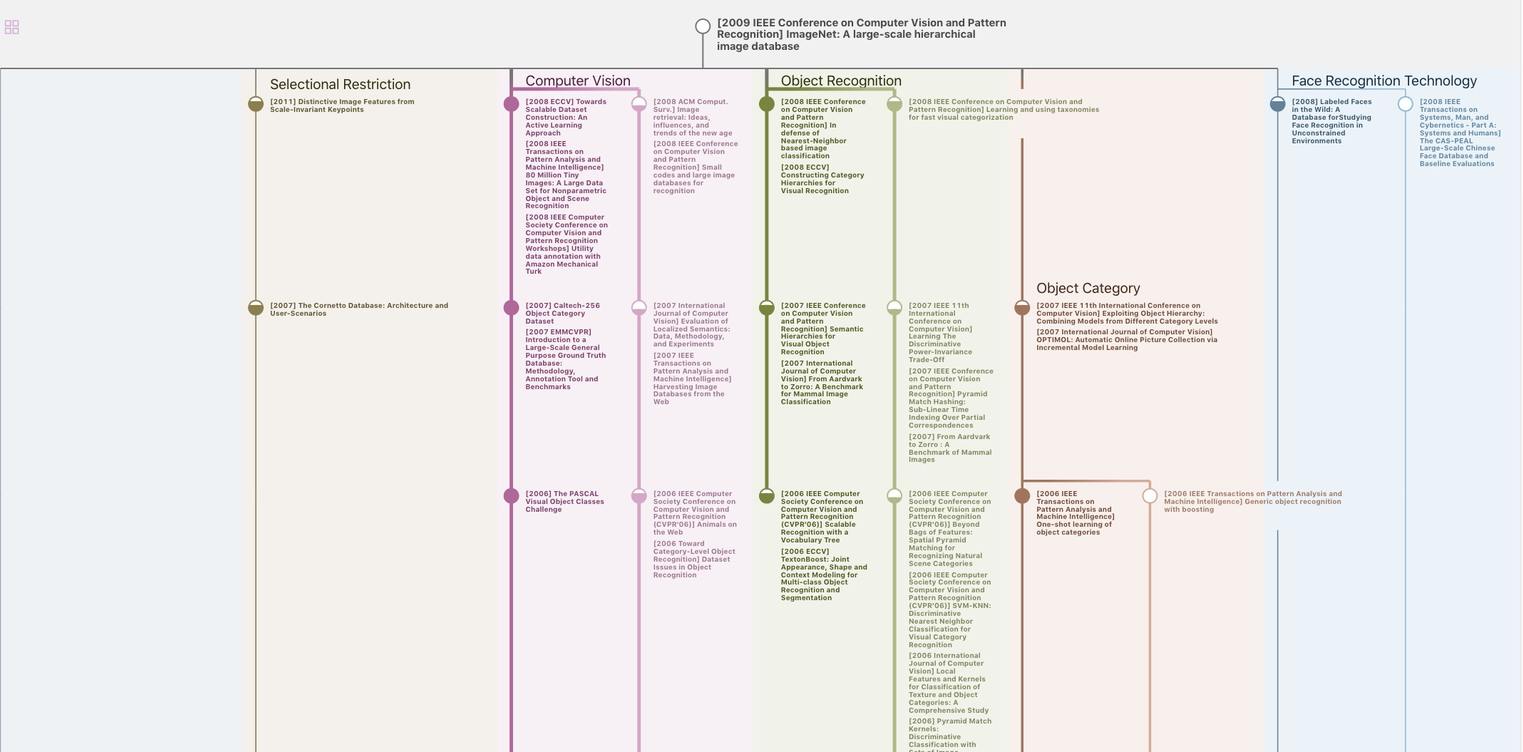
生成溯源树,研究论文发展脉络
Chat Paper
正在生成论文摘要