Multi-Modality is All You Need for Transferable Recommender Systems
CoRR(2023)
摘要
ID-based Recommender Systems (RecSys), where each item is assigned a unique
identifier and subsequently converted into an embedding vector, have dominated
the designing of RecSys. Though prevalent, such ID-based paradigm is not
suitable for developing transferable RecSys and is also susceptible to the
cold-start issue. In this paper, we unleash the boundaries of the ID-based
paradigm and propose a Pure Multi-Modality based Recommender system (PMMRec),
which relies solely on the multi-modal contents of the items (e.g., texts and
images) and learns transition patterns general enough to transfer across
domains and platforms. Specifically, we design a plug-and-play framework
architecture consisting of multi-modal item encoders, a fusion module, and a
user encoder. To align the cross-modal item representations, we propose a novel
next-item enhanced cross-modal contrastive learning objective, which is
equipped with both inter- and intra-modality negative samples and explicitly
incorporates the transition patterns of user behaviors into the item encoders.
To ensure the robustness of user representations, we propose a novel noised
item detection objective and a robustness-aware contrastive learning objective,
which work together to denoise user sequences in a self-supervised manner.
PMMRec is designed to be loosely coupled, so after being pre-trained on the
source data, each component can be transferred alone, or in conjunction with
other components, allowing PMMRec to achieve versatility under both
multi-modality and single-modality transfer learning settings. Extensive
experiments on 4 sources and 10 target datasets demonstrate that PMMRec
surpasses the state-of-the-art recommenders in both recommendation performance
and transferability. Our code and dataset is available at:
https://github.com/ICDE24/PMMRec.
更多查看译文
AI 理解论文
溯源树
样例
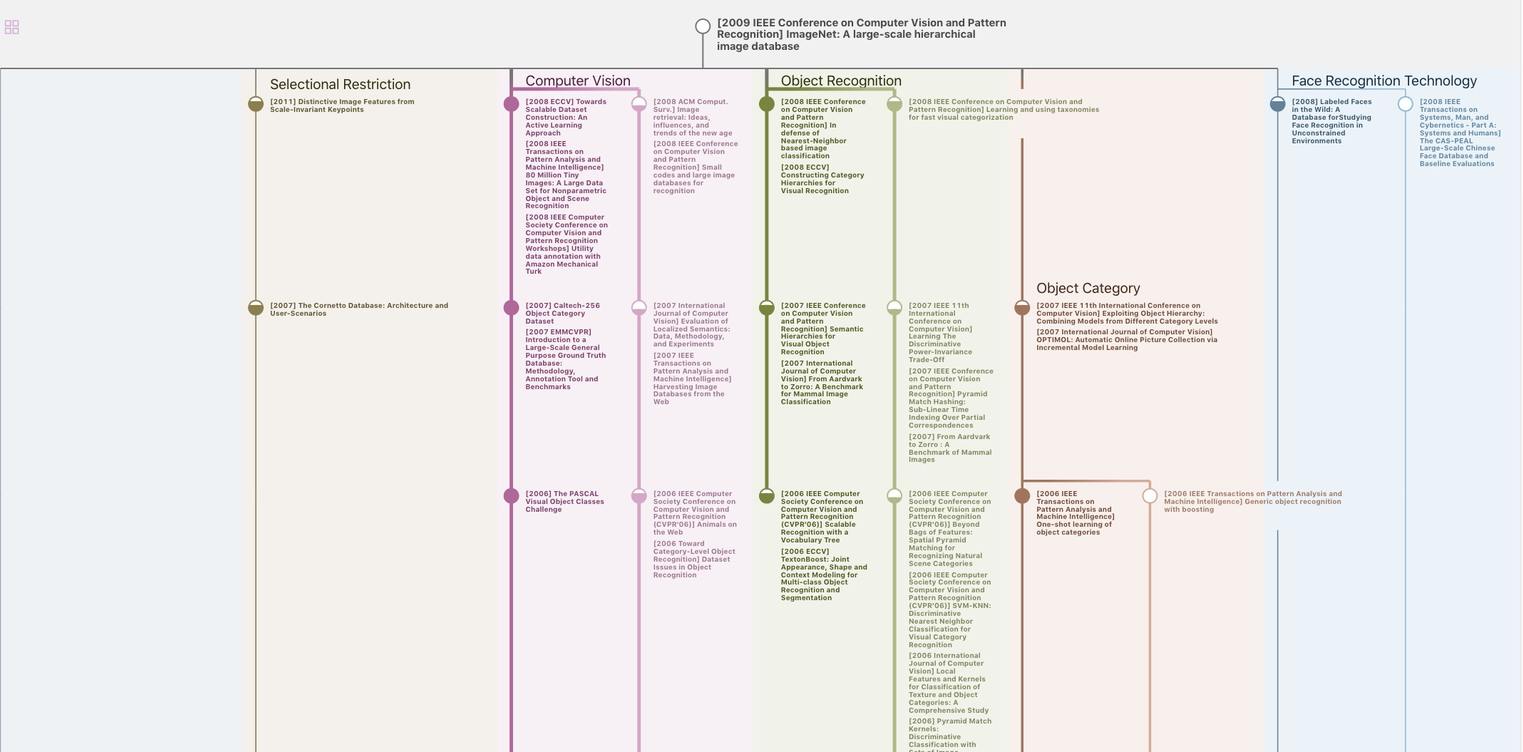
生成溯源树,研究论文发展脉络
Chat Paper
正在生成论文摘要