A novel random spectral similar component decomposition method and its application to gear fault diagnosis
MECHANICAL SYSTEMS AND SIGNAL PROCESSING(2024)
摘要
Sparse random mode decomposition (SRMD) is a decomposition approach established by combining sparse random feature model with clustering algorithm. It is not subject to the sampling process of signal and can mitigate mode mixing. However, the performance of SRMD is limited by its own hyperparameters, and it is prone to derive inaccurate clustering decomposition results when processing strong noise interference signal. To overcome these defects, a novel method called random spectral similar component decomposition (RSSCD) is proposed. In RSSCD, the time-frequency localized features produced by randomization and sparsification are adopted to represent the input signal. Subsequently, the initial signal components formed by sparse random features are taken as a whole, and the spectral similarity criterion is defined to adaptively form independent random components (RCs), thus improving the accuracy of decomposition. Furthermore, RSSCD is applied to gear fault diagnosis, and a fault significance measure (FSM) index is designed to guide the selection of parameter in sparse random feature representation, which ensures the fault information richness of the required RCs. Finally, the feasibility and effectiveness of RSSCD are fully validated by simulation signals and two experimental cases. The results indicate that RSSCD has excellent decomposition performance and fault feature extraction ability.
更多查看译文
关键词
Random spectral similar component,decomposition,Spectral similarity criterion,Fault significance measure,Gear fault diagnosis
AI 理解论文
溯源树
样例
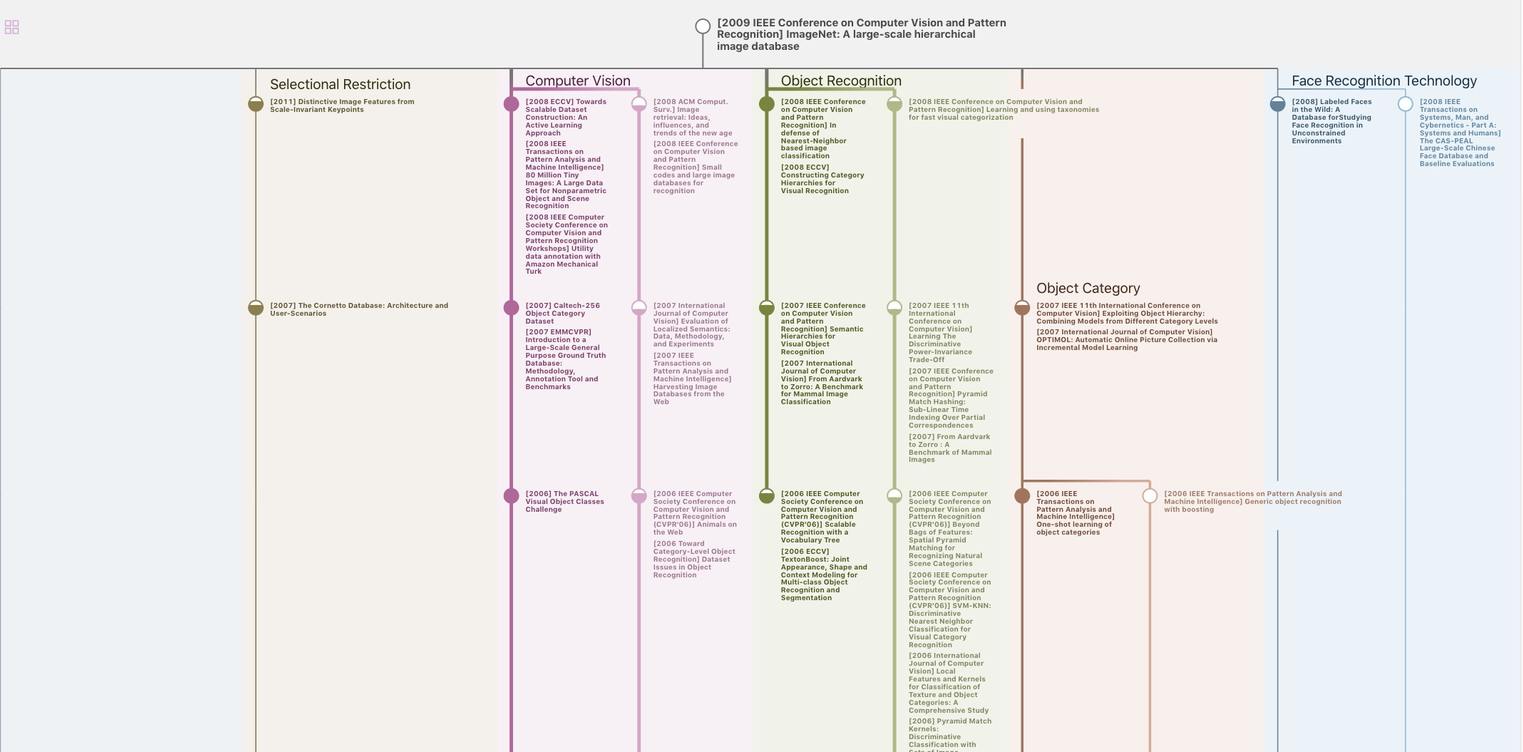
生成溯源树,研究论文发展脉络
Chat Paper
正在生成论文摘要