Black-box attacks on dynamic graphs via adversarial topology perturbations
NEURAL NETWORKS(2024)
摘要
Research and analysis of attacks on dynamic graph is beneficial for information systems to investigate vulnerabilities and strength abilities in resisting malicious attacks. Existing attacks on dynamic graphs mainly focus on rewiring original graph structures, which are often infeasible in real-world scenarios. To address this issue, we adopt a novel strategy by injecting both fake nodes and links to attack dynamic graphs. Based on that, we present the first study on attacking dynamic graphs via adversarial topology perturbations in a restricted black-box setting, in which downstream graph learning tasks are unknown. Specifically, we first divide dynamic graph structure perturbations into three sub-tasks and transform them as a sequential decision making process. Then, we propose a hierarchical reinforcement learning based black-box attack (HRBBA) framework to model three sub-tasks as attack policies. In addition, an imperceptible perturbation constraint to guarantee the concealment of attacks is incorporated into HRBBA. Finally, HRBBA is optimized based on the actor-critic process. Extensive experiments on four real-world dynamic graphs show that the performance of diverse dynamic graph learning methods (victim methods) on tasks like link prediction, node classification and network clustering can be substantially degraded under HRBBA attack.
更多查看译文
关键词
Graph representation,Dynamic graph,Node injection,Adversarial attack,Reinforcement learning
AI 理解论文
溯源树
样例
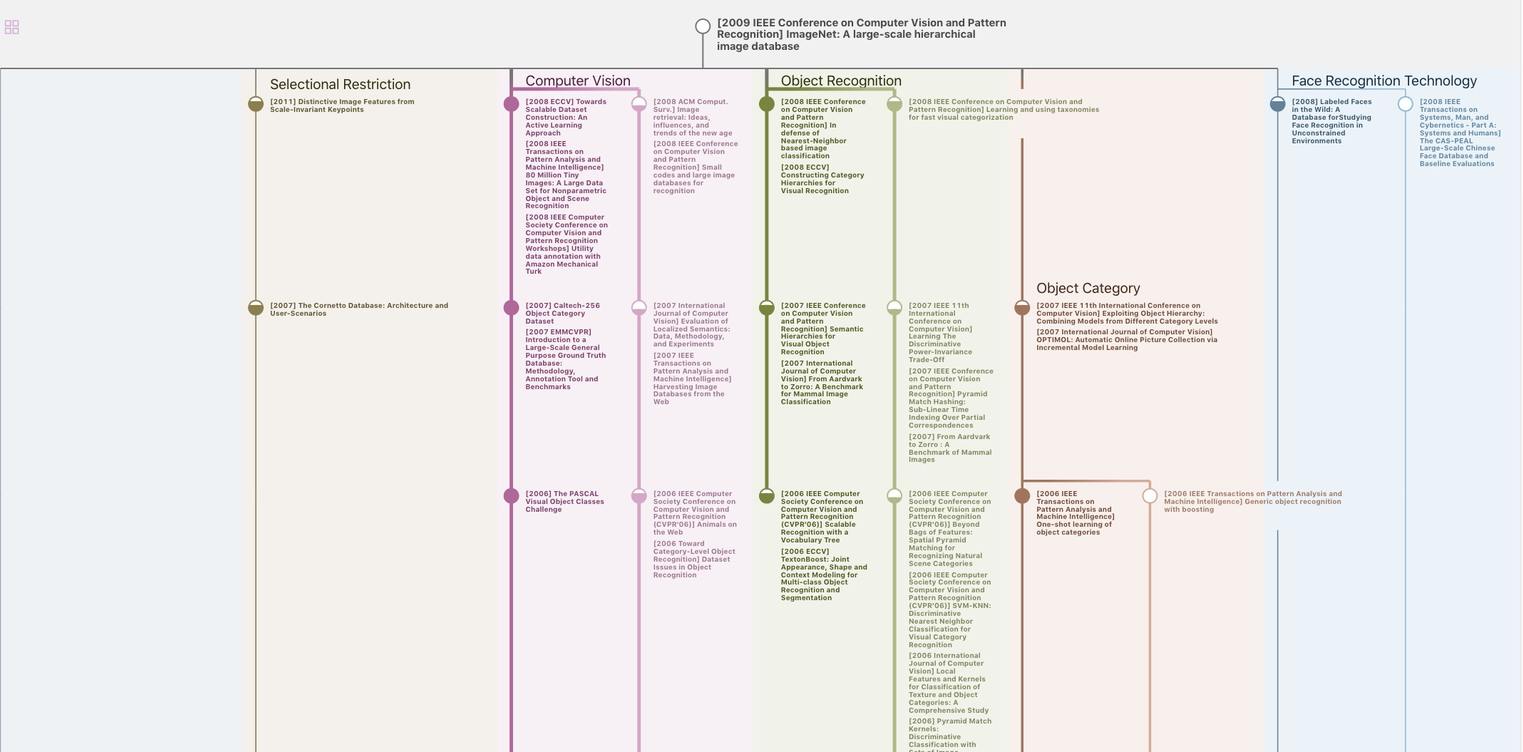
生成溯源树,研究论文发展脉络
Chat Paper
正在生成论文摘要