A novel semi-blind source separation framework towards maximum signal-to-interference ratio
SIGNAL PROCESSING(2024)
摘要
For the independence-based blind source separation (BSS) methods, effective modeling of the source signals is important. When applying the auxiliary function techniques, different source models normally result in different forms of the weighted covariance matrices, which in turn are used to compute the demixing filters. This paper proposes a new algorithmic framework termed minimum variance independent component analysis (MVICA), which is rigorously derived from the conventional BSS methods by determining the weighted covariance matrices with the maximum signal-to-interference ratio (SIR) criterion. The maximum-SIR weighted covariance matrix is approximately proved to be the interference covariance matrix. A deep neural network-supported implementation of the proposed MVICA algorithm is subsequently presented. Furthermore, we give a revealing insight into the relationships between MVICA and the existing speech separation approaches including conventional BSS methods and several mainstream beamforming techniques. Experimental results show the superiority of MVICA over the state-of-the-art BSS techniques and beamformers under various conditions, in terms of not only SIR but also signal-to-distortion ratio, speech intelligibility and perceptual quality and automatic speech recognition accuracy.
更多查看译文
关键词
Blind source separation,Independent component analysis,Maximum SIR,Deep neural network
AI 理解论文
溯源树
样例
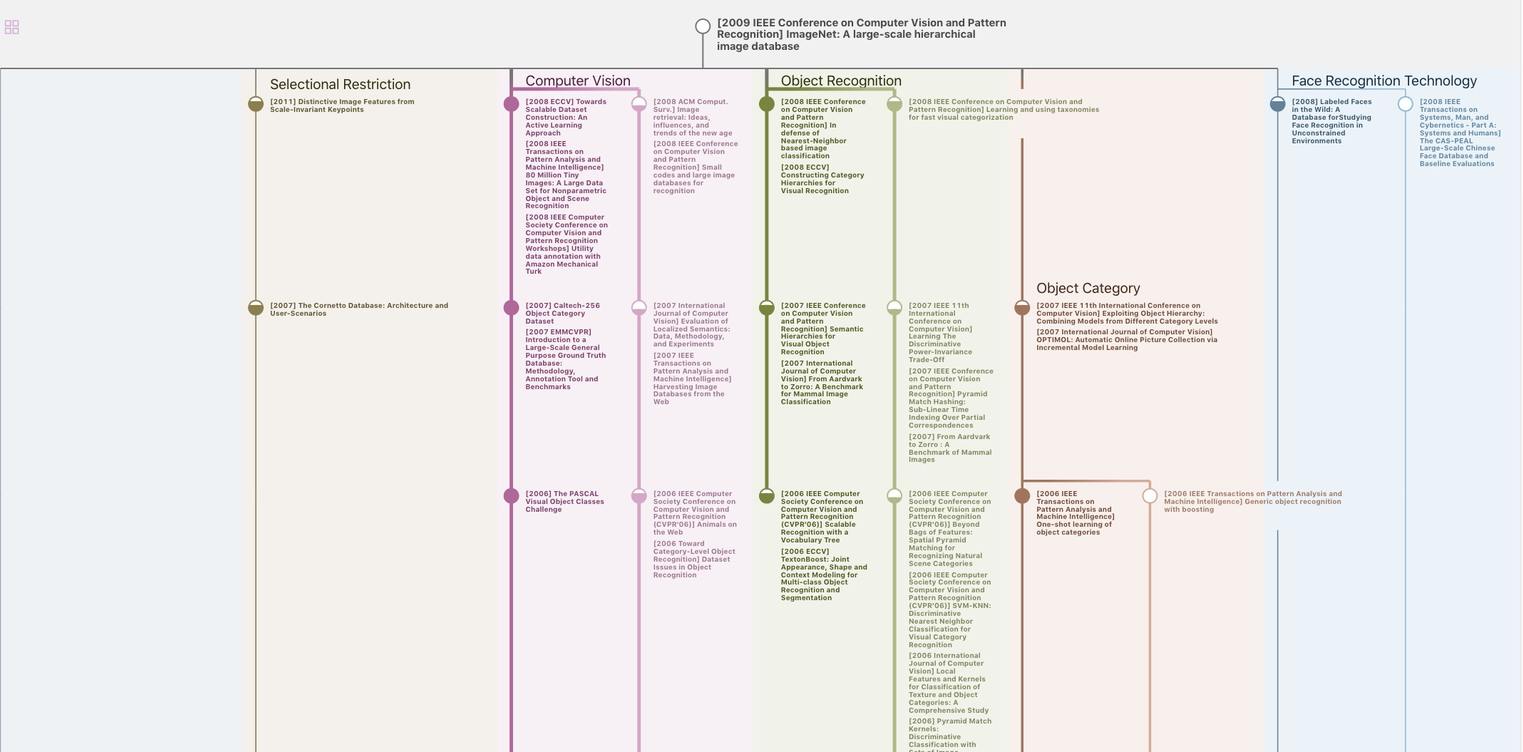
生成溯源树,研究论文发展脉络
Chat Paper
正在生成论文摘要