Exploring the Impact of the Signal-to-Noise Ratio Assumption on the Time Series Bootstrap Pairwise Dependence Hypothesis Test.
2023 14th International Conference on Information, Intelligence, Systems & Applications (IISA)(2023)
摘要
Distance correlation (dCorr) is a test statistic that can identify non-linear dependence patterns between random variables. Variations of dCorr have been applied in sequences of dependent random variables, including time series. The necessity of exploring dependencies between lags of time series or cross-dependence between distinct time series has resulted in closed forms of dCorr distributions for the case of null hypothesis—independence. Nonetheless, their assumptions and complex expressions hinder their application to a variety of forecasting applications. Consequently, non-parametric dependence hypothesis tests procedures—e.g., bootstraps—appear as an adequate alternative. However, consistent bootstrap approaches require stationary time series and, as a result, signal transformations. On the one hand, there is a plethora of such transformations; on the other, each of these silently imply different assumptions regarding the ratio of the deterministic elements over “noisy” elements of the time series, i.e., the signal-to-noise ratio. The aim of this paper is to explore the effect of the implied signal-to-noise ratio on the hypothesis p-values as derived by a bootstrap hypothesis test procedure. The relationship of these p-values with the stationary transformations (and the corresponding signal-to-noise ratio) is demonstrated with currency exchange-related time series.
更多查看译文
关键词
time series,pairwise dependence,bootstrap procedure,distance correlation,currency exchange rates
AI 理解论文
溯源树
样例
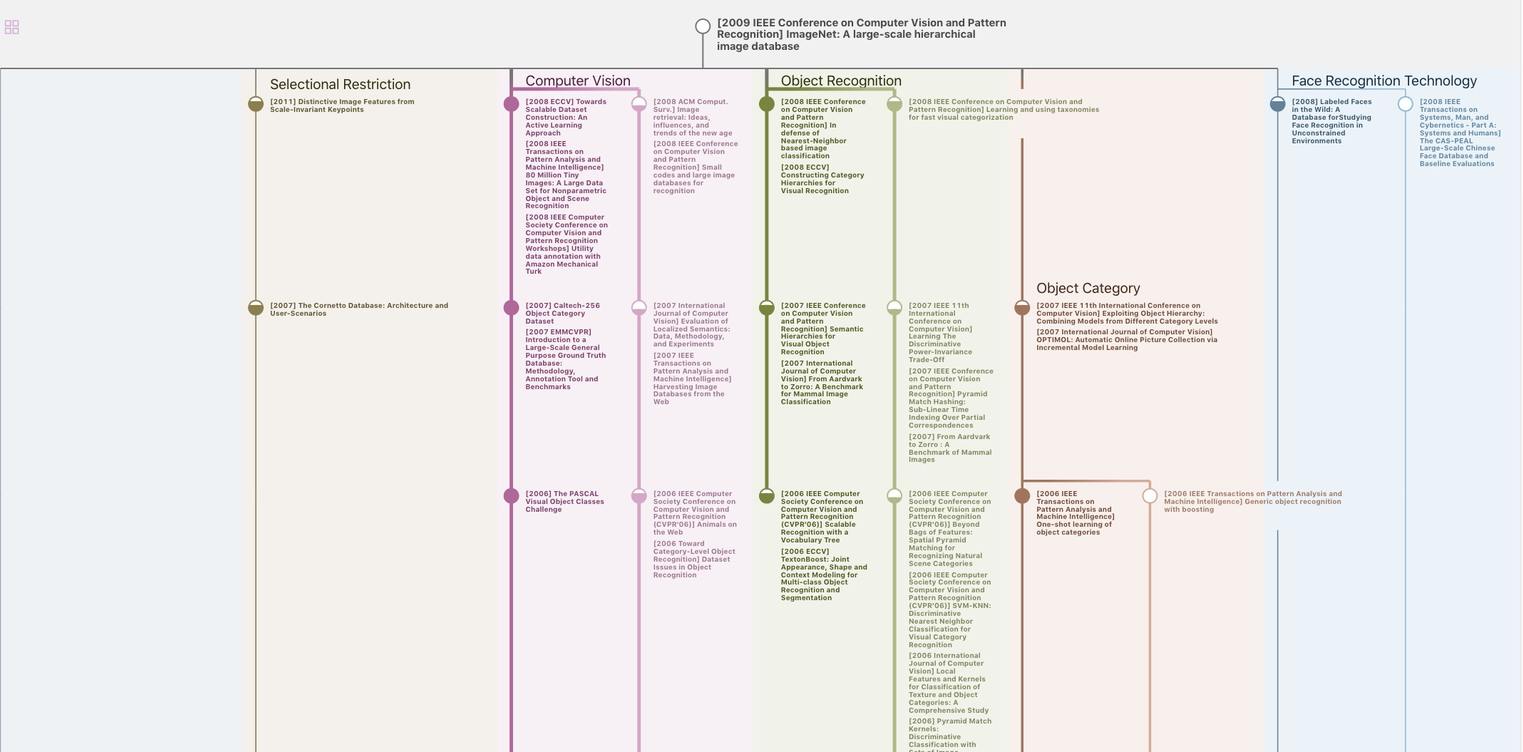
生成溯源树,研究论文发展脉络
Chat Paper
正在生成论文摘要