Identifying Autism Spectrum Disorder Using Brain Networks: Challenges and Insights.
2023 14th International Conference on Information, Intelligence, Systems & Applications (IISA)(2023)
摘要
Autism Spectrum Disorder (ASD) affects a large portion of the global population both directly and indirectly. The biological etiology of the disorder is not sufficiently understood, and current diagnoses rely on behavioural indicators which do not provide a reliable basis for diagnosis until about 2 years of age. Identifying a biological marker of ASD would aid in understanding the disorder and potentially allow for earlier, more objective diagnoses and treatments to improve the quality of life of individuals possessing ASD. The analysis of functional connectivity in the brain using functional Magnetic Resonance Imaging (fMRI) has been identified as a promising method for discovering such biological markers. This study recreated a prominent state-of-the-art work in explainable classification of brain networks, but found results inconsistent with what was claimed. The methods were modified in various ways to improve accuracy and performance. A new, simpler method named Discriminative Edges (DE) was developed which achieved similar accuracies with improved performance and explainability. DE was also adapted to receive raw correlation matrices as well as thresholded correlation matrices representing brain networks, and it was found that raw correlation matrices provided more useful information for classification. An imple-mentation package was provided to aid future researchers in validating and improving upon these results. Suggestions for future work based on the findings of this study were provided, the most important being to procure more datasets, discover data-driven subcategories of ASD, and maintain reproducibility in studies.
更多查看译文
关键词
neuroimaging,fMRI,machine learning,diagnosis,brain networks
AI 理解论文
溯源树
样例
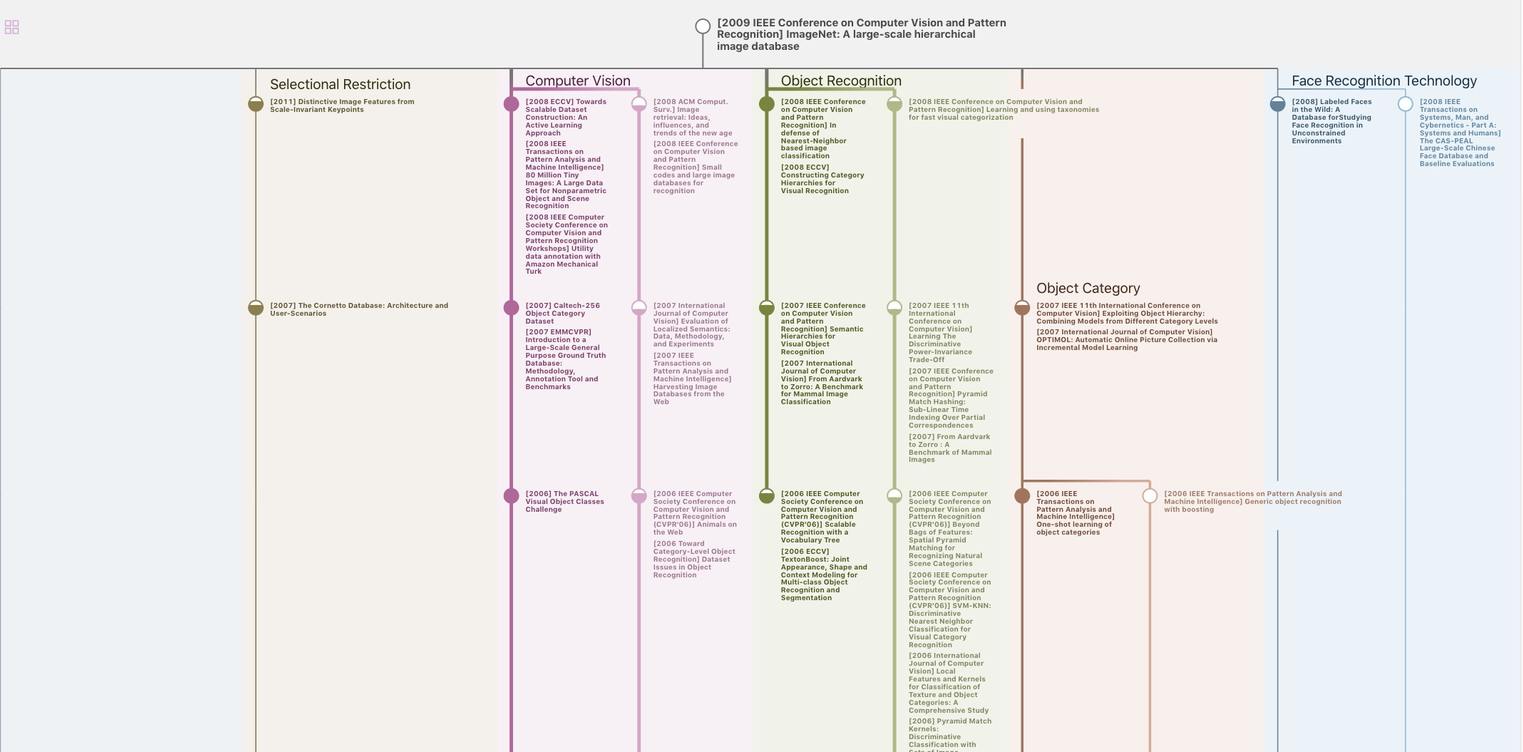
生成溯源树,研究论文发展脉络
Chat Paper
正在生成论文摘要