Multi-label feature selection via adaptive dual-graph optimization
EXPERT SYSTEMS WITH APPLICATIONS(2024)
摘要
As in single-label learning, multi-label learning (MLL) also suffers from the problem of "the curse of dimensionality"due to the redundancy of features in the original data. To address this problem, extensive research has been conducted to explore the various feature selection algorithms aiming to eliminate irrelevant and redundant features. Among these, the manifold learning based methods have gained significant attention for their ability to capture local label correlations. However, previous approaches have mainly focused on extracting information from data space, overlooking valuable information in feature space. In addition, these methods conduct manifold learning and feature selection independently, thereby degrading the learning performance. To tackle this problem, we propose a novel multi-label feature selection method based on adaptive dual-graph optimization (MFS-ADGO) to learn the geometric structure of the data and select discriminative feature subsets simultaneously. Specifically, a dual-graph manifold regularization is constructed first, to locally capture label correlations in the data and feature spaces for discriminative features selection. A constraint is then imposed on the similarity matrices to adaptively learn the local geometric structure of the data during the feature selection, thereby capturing more reliable information from the original data. To facilitate feature selection, an objective function incorporating the l(2,1-2)-norm is proposed, along with an iterative updating algorithm. Extensive experiments unequivocally demonstrated the efficacy of MFS-ADGO in enhancing state-of-the-art feature selection methods in the realm of MLL.
更多查看译文
关键词
Multi-label learning,Feature selection,Adaptive dual-graph learning,Non-convex sparse regression
AI 理解论文
溯源树
样例
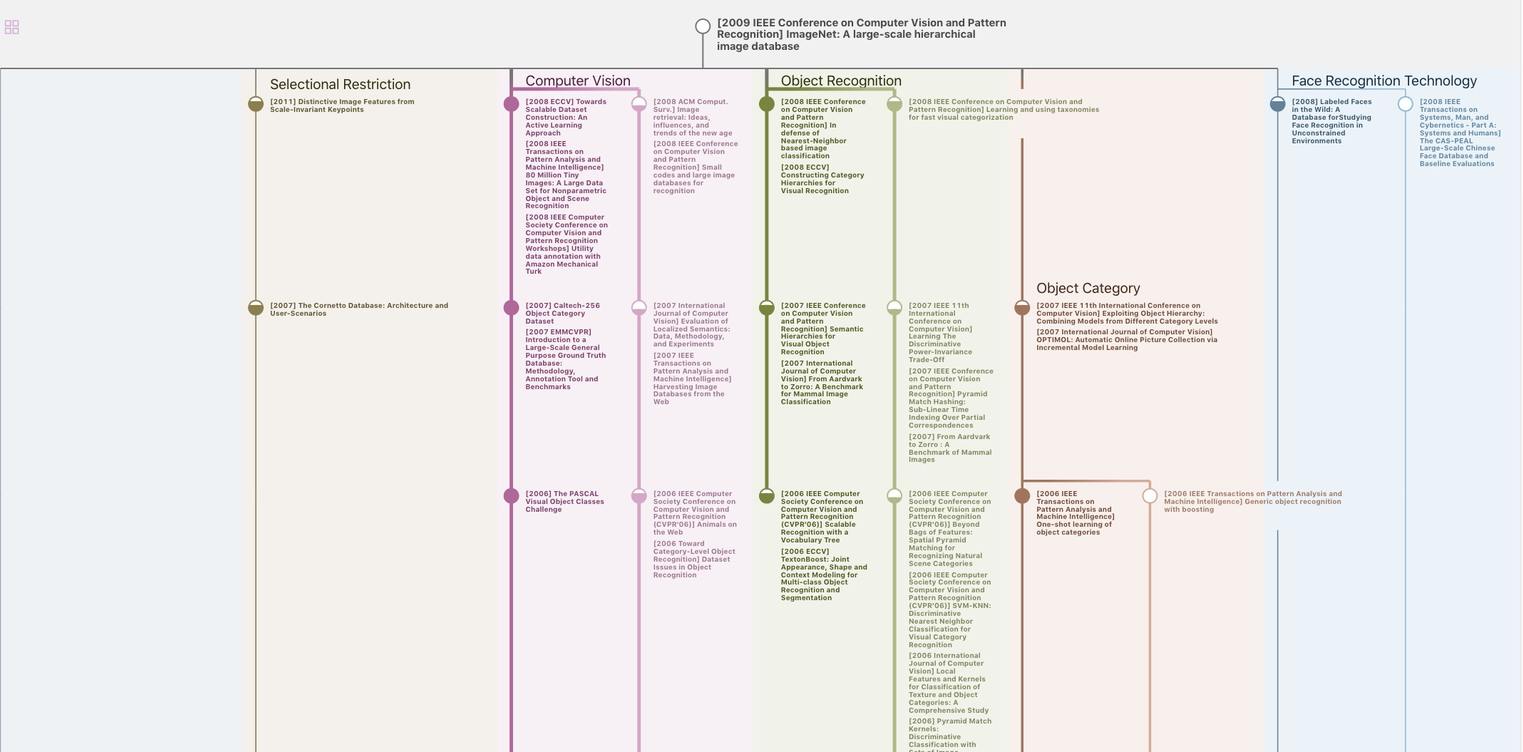
生成溯源树,研究论文发展脉络
Chat Paper
正在生成论文摘要