Modelling and validation of liquefaction potential index of fine-grained soils using ensemble learning paradigms
SOIL DYNAMICS AND EARTHQUAKE ENGINEERING(2024)
摘要
This study explores the utilization of ensemble-based soft computing techniques for predicting the liquefaction potential of fine-grained soils. Generally, deterministic methods are used to assess the possibility of liquefactions. However, recent advancements have highlighted the implementation of machine learning techniques considering the diverse geotechnical characteristics for liquefaction susceptibility analysis. The current study predicts liquefaction potential index (LPI) of soils using five ensemble-based soft computing paradigms viz., decision tree, random forest, gradient boosting, AdaBoost, and XGBoost regressors. Various influential factors including stress parameters, depth of soil stratum, peak ground acceleration, earthquake magnitude, cyclic stress ratio, fine content, and SPT- N value, were used for estimating the LPI of soils. Experimental results of the employed models were assessed using multiple statistical indices. The results demonstrate that the employed XGBoost model achieved higher predictive accuracy with 99 % accuracy (based on R2 index) during the testing phase. Overall results exhibit that the employed XGBoost paradigm can be utilized as a viable alternative to empirical approaches, enabling an early assessment of liquefaction susceptibility in different engineering projects. This will expedite identification and evaluation of liquefaction hazards, allowing engineers to make more informed decisions.
更多查看译文
关键词
Soil Liquefaction,Liquefaction potential index,Seismic vulnerability,Geotechnical engineering,Artificial intelligence,Ensemble learning
AI 理解论文
溯源树
样例
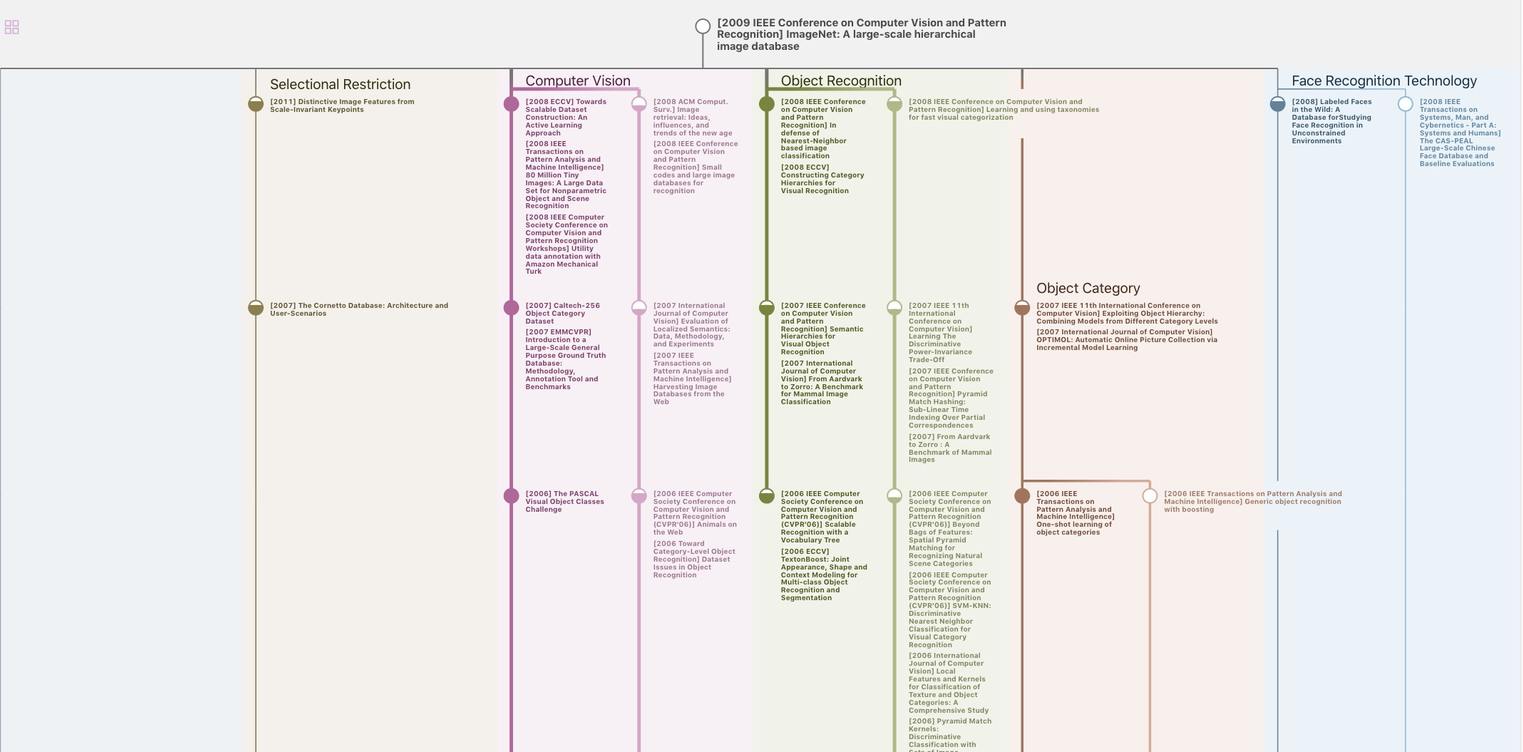
生成溯源树,研究论文发展脉络
Chat Paper
正在生成论文摘要