Double-level View-correlation Multi-view Subspace Clustering
KNOWLEDGE-BASED SYSTEMS(2024)
摘要
In recent years, significant progress has been made in Multi-view Subspace Clustering (MSC). Most existing MSC methods attempt to explore and exploit the view correlations of multi-view data to boost the clustering performance. They achieve the subspace matrices of different views from the original feature space directly. However, the diversity view-correlation and consistency-view correlation of multi-view data are two antago-nistic properties, which are improper and challenging to be captured in such a straightforward process. To simultaneously and properly investigate the two antagonistic properties of multi-view data, a novel Double -level View-correlation Multi-view Subspace Clustering method, named DV-MSC, is introduced in this paper. To be specific, DV-MSC adopts a strategy that deals with the diversity view-correlation and consistency view -correlation in different levels: (1) low-level, which excavates the diversity view-correlation in the feature space, and (2) high-level, which explores the consistency view-correlation in subspace representations. The underlying assumption is that different views should be diverse in the feature space while having the same clustering results, in other words, the proposed method explores the Diversity in Low-level Feature Content (DLFC) and the Consistency in High-level Clustering Structure (CHCS). Experimental results show the promising and competitive clustering performance of DV-MSC, compared to several existing state-of-the-arts.
更多查看译文
关键词
Multi-view clustering,Subspace clustering,View-correlation
AI 理解论文
溯源树
样例
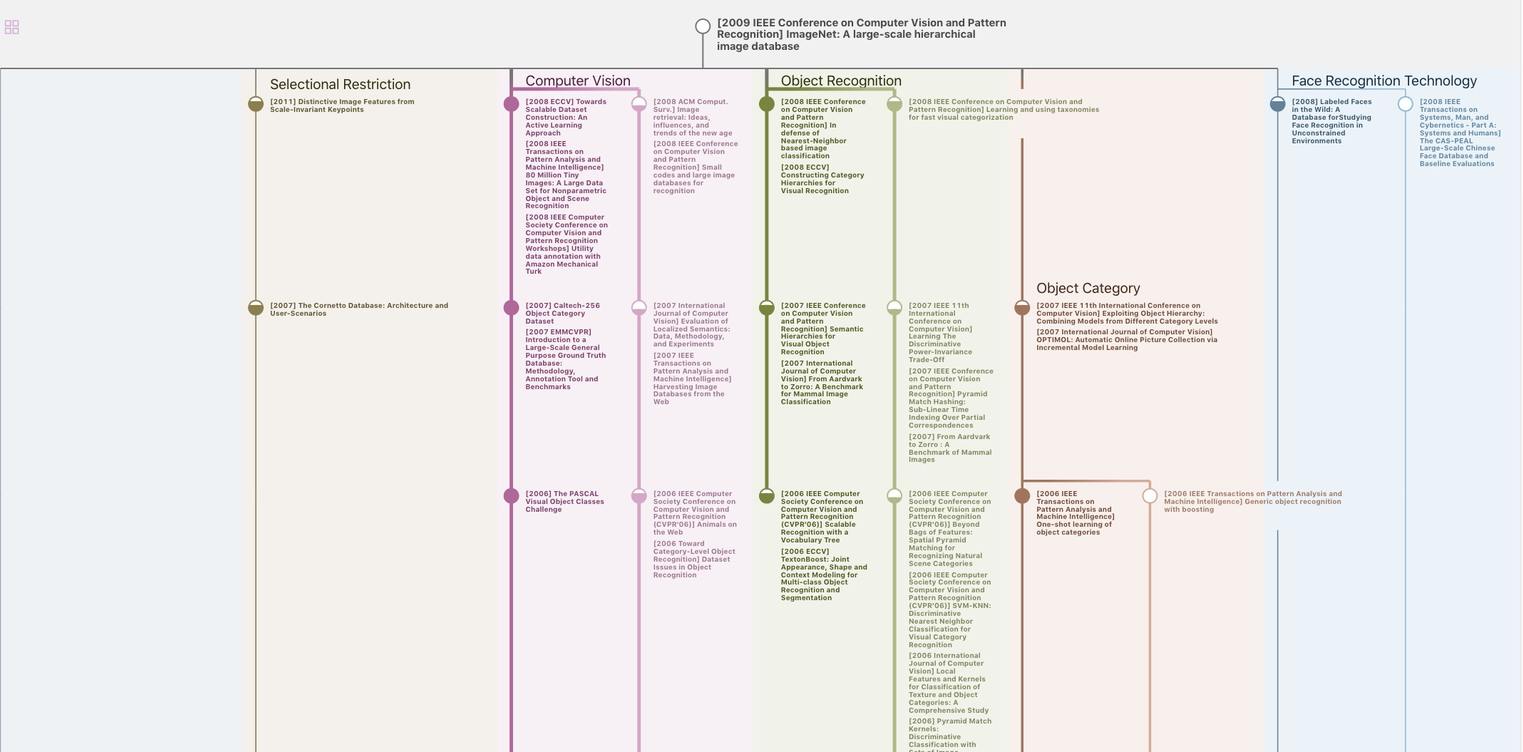
生成溯源树,研究论文发展脉络
Chat Paper
正在生成论文摘要