A Pruning Method with Adaptive Adjustment of Channel Pruning Rate
2023 IEEE 4th International Conference on Pattern Recognition and Machine Learning (PRML)(2023)
摘要
Convolutional neural networks have different channel pruning sensitivities for different layers, and the existing network pruning methods cannot perform adaptive pruning of channels within each layer according to the sensitivity, resulting in irreversible damage to the network during the pruning process. To solve this problem, a network pruning method with adaptive adjustment of channel pruning rate is proposed in this paper, which disassembles the dynamic adjustment process of channel pruning rate in each layer into an iterative optimization problem and finally obtains a network structure with high compression rate and high accuracy. This method performs iterative pruning of the network under the supervision of loss constraint according to the preset pruning initial step for the benchmark model, and then chooses to reduce the pruning step or skip the pruning of this layer according to the accuracy loss of the network. Finally achieves the pruning of each layer in the network with Appropriate pruning rates after multiple iterations. Experimental results show that this method can maintain 93.64% recognition accuracy in CIFAR10-VGG16 pruning task with 87.45% reduction of parameter amount, which has higher compression rate and less accuracy loss compared with PEEC, FPGM, RFP and other pruning algorithms.
更多查看译文
关键词
network pruning,channel pruning rate,adaptive adjustment,loss constraint,iterative method
AI 理解论文
溯源树
样例
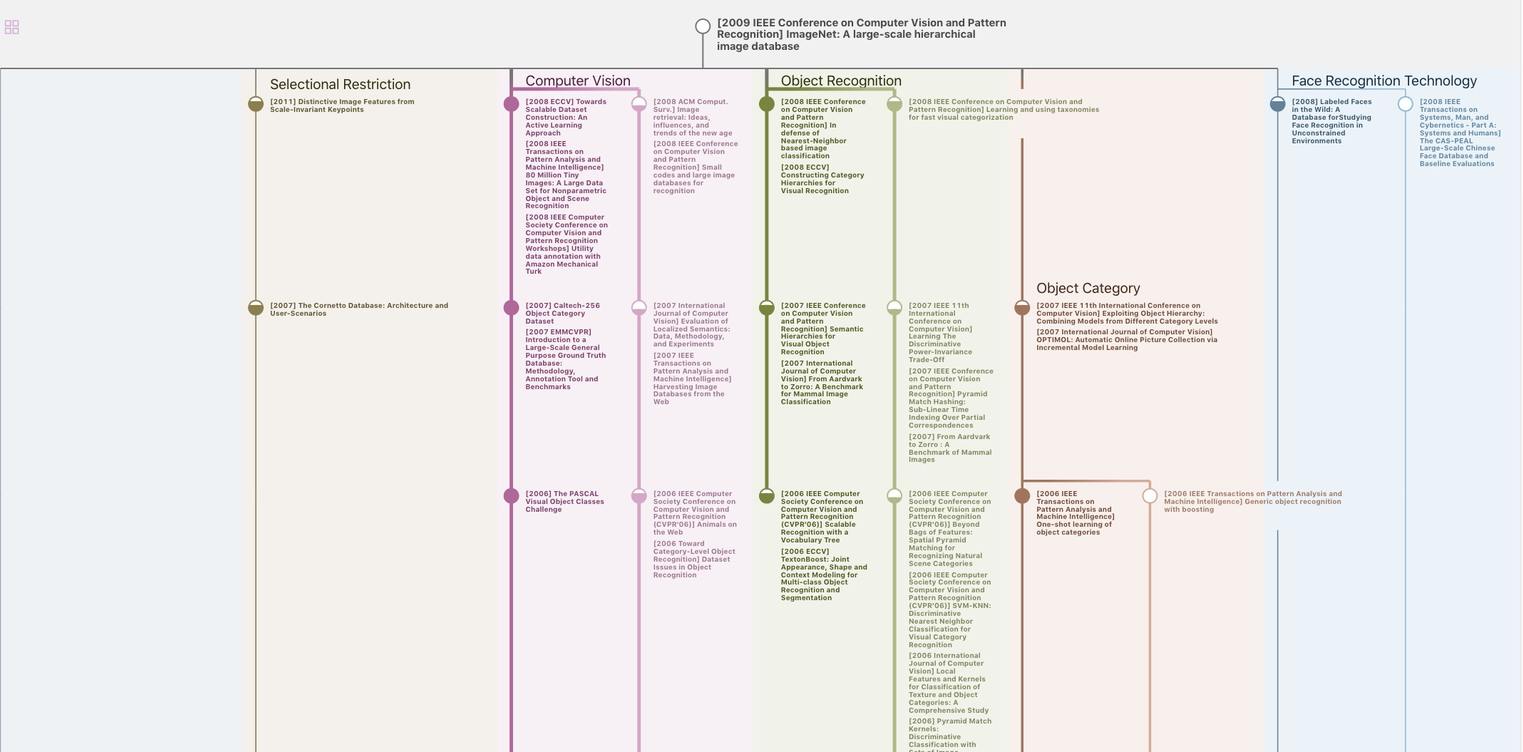
生成溯源树,研究论文发展脉络
Chat Paper
正在生成论文摘要