Extraction of Motion Change Points Based on the Physical Characteristics of Objects
2023 IEEE 4th International Conference on Pattern Recognition and Machine Learning (PRML)(2023)
摘要
Recently, it has become increasingly important for artificial intelligence to understand the real world through the ability of intuitive physics, which is our innate ability to understand the real world. Many previous studies aiming at real-world understanding have based image inference for real world recognition, usually based on inference from image features or recognition of objects in an image. In contrast, we propose a model to obtain and predict the change points of real-world motions represented by the potential hierarchical structure of the physical relations of the observed objects represented by graph embeddings. We conducted experiments on the CLEVRER dataset [1] to detect motion change points and extract predictive change points, and found that the proposed model is correctly trained as a predictive change point extraction model.
更多查看译文
关键词
graph embeddings,physical characteristics,latent hierarchical structure of physical relationships
AI 理解论文
溯源树
样例
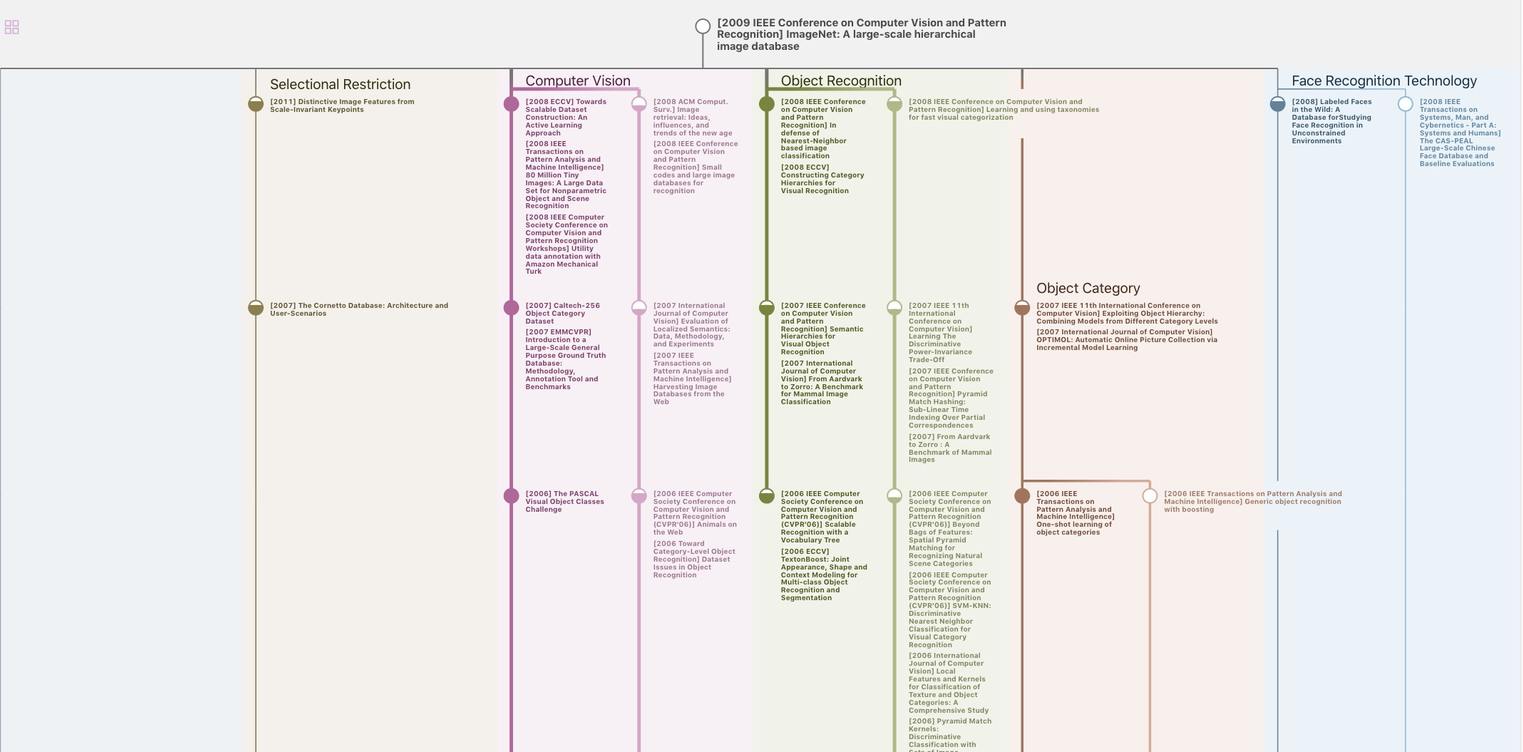
生成溯源树,研究论文发展脉络
Chat Paper
正在生成论文摘要