REAL-X-Robot Open-Ended Autonomous Learning Architecture: Building Truly End-to-End Sensorimotor Autonomous Learning Systems
IEEE TRANSACTIONS ON COGNITIVE AND DEVELOPMENTAL SYSTEMS(2023)
摘要
Open-ended learning is a core research field of developmental robotics and AI aiming to build learning machines and robots that can autonomously acquire knowledge and skills incrementally as infants. The first contribution of this work is to highlight the challenges posed by the previously proposed benchmark "REAL competition" fostering the development of truly open-ended learning robots. The benchmark involves a simulated camera-arm robot that: 1) in a first "intrinsic phase" acquires sensorimotor competence by autonomously interacting with objects and 2) in a second "extrinsic phase" is tested with tasks, unknown in the intrinsic phase, to measure the quality of previously acquired knowledge. The benchmark requires the solution of multiple challenges usually tackled in isolation, in particular exploration, sparse-rewards, object learning, generalization, task/goal self-generation, and autonomous skill learning. As a second contribution, the work presents a "REAL-X" architecture. Different systems implementing the architecture can solve different versions of the benchmark progressively releasing initial simplifications. The REAL-X systems are based on a planning approach that dynamically increases abstraction and on intrinsic motivations to foster exploration. Some systems achieves a good performance level in very demanding conditions. Overall, the REAL benchmark is shown to represent a valuable tool for studying open-ended learning in its hardest form.
更多查看译文
关键词
Autonomous robot,benchmark,competition,intrinsic motivation,open-ended learning,planning,simulation
AI 理解论文
溯源树
样例
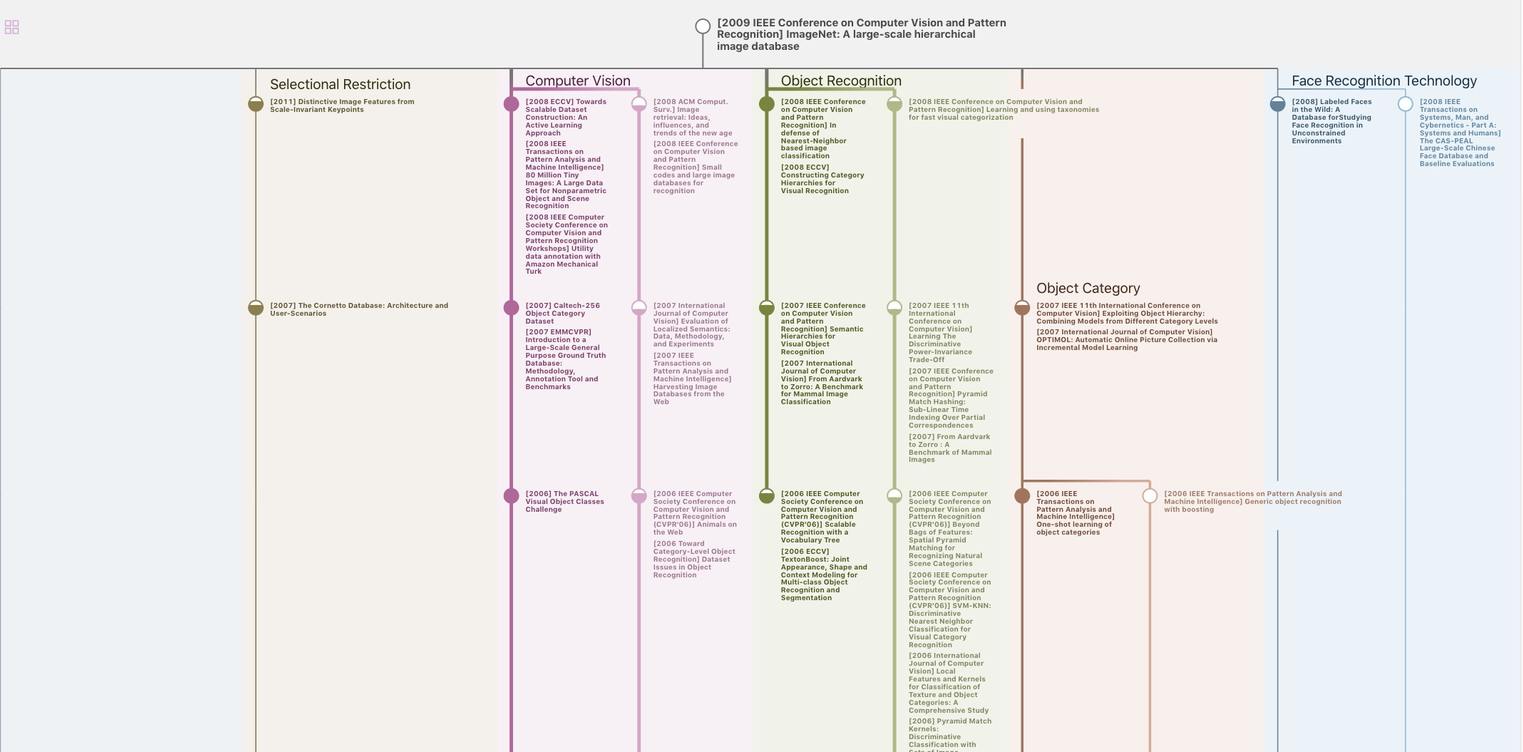
生成溯源树,研究论文发展脉络
Chat Paper
正在生成论文摘要