Offloading Dependent Tasks in Edge Computing With Unknown System-Side Information
IEEE TRANSACTIONS ON SERVICES COMPUTING(2023)
摘要
We consider the problem of dependent task offloading in edge computing with unknown system-side information (e.g., edge transmission rate and computation resources). In this problem, tasks have complicated dependency relationships and have no prior knowledge of system-side information to assist offloading decision-making. Although existing learning-based approaches can help to address unknown system-side information, the impact of inherent task dependency on such approaches has not been formally explored. To bridge the gap, we first use a breadth-first-search (BFS) method to decouple task dependency, and then leverage the Lyapunov optimization technique to transfer the long-term offloading problem to an online optimization problem. Furthermore, we employ the multi-armed bandit (MAB) theory to develop the online learning-based dependent task offloading algorithm, called OL-DTO. The algorithm can address the unknown system-side information and is augmented with task dependency awareness. We present a rigorous theoretical analysis to evaluate the performance of this algorithm in terms of application delay and UD energy consumption. Our extensive experimental results demonstrate that the OL-DTO algorithm significantly reduces application delay while satisfying the long-term energy budget constraint of the UD.
更多查看译文
关键词
Unknown system-side information,task dependency,concurrent-enhanced offloading,learning-based offloading,multi-armed bandit theory
AI 理解论文
溯源树
样例
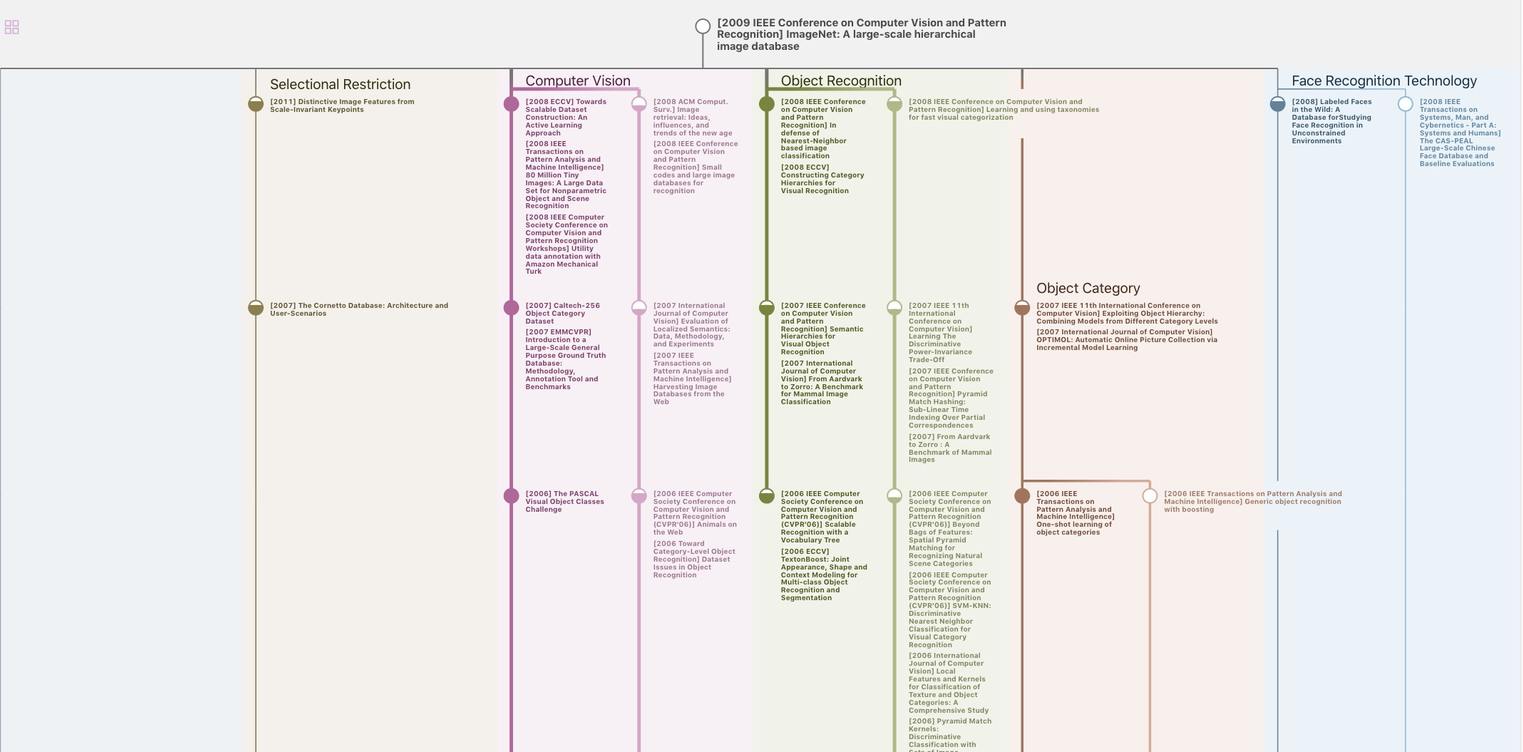
生成溯源树,研究论文发展脉络
Chat Paper
正在生成论文摘要