Spatial-temporal graph-guided global attention network for video-based person re-identification
Machine Vision and Applications(2023)
摘要
Global attention learning has been extensively applied in video-based person re-identification due to its superiority in capturing contextual correlations. However, existing global attention learning methods usually adopt the conventional neural network to model non-Euclidean contextual correlations, resulting in a limited representation ability. Inspired by the graph-structure property of the contextual correlations, we propose a spatial-temporal graph-guided global attention network (STG 3 A) for video-based person re-identification. STG 3 A comprises two graph-guided attention modules to capture the spatial contexts within a frame and temporal contexts across all frames in a sequence for global attention learning. Furthermore, the graphs from both modules are encoded as graph representations, which combine with weighted representations to grasp the spatial-temporal contextual information adequately for video feature learning. To reduce the effect of noisy graph nodes and learn robust graph representations, a graph node attention is developed to trade-off the importance of each graph node, leading to noise-tolerant graph models. Finally, we design a graph-guided fusion scheme to integrate the representations output by these two attentive modules for a more compact video feature. Extensive experiments on MARS and DukeMTMCVideoReID datasets demonstrate the superior performance of the STG 3 A.
更多查看译文
关键词
Person Re-identification,Global attention learning,Graph,Spatial-temporal
AI 理解论文
溯源树
样例
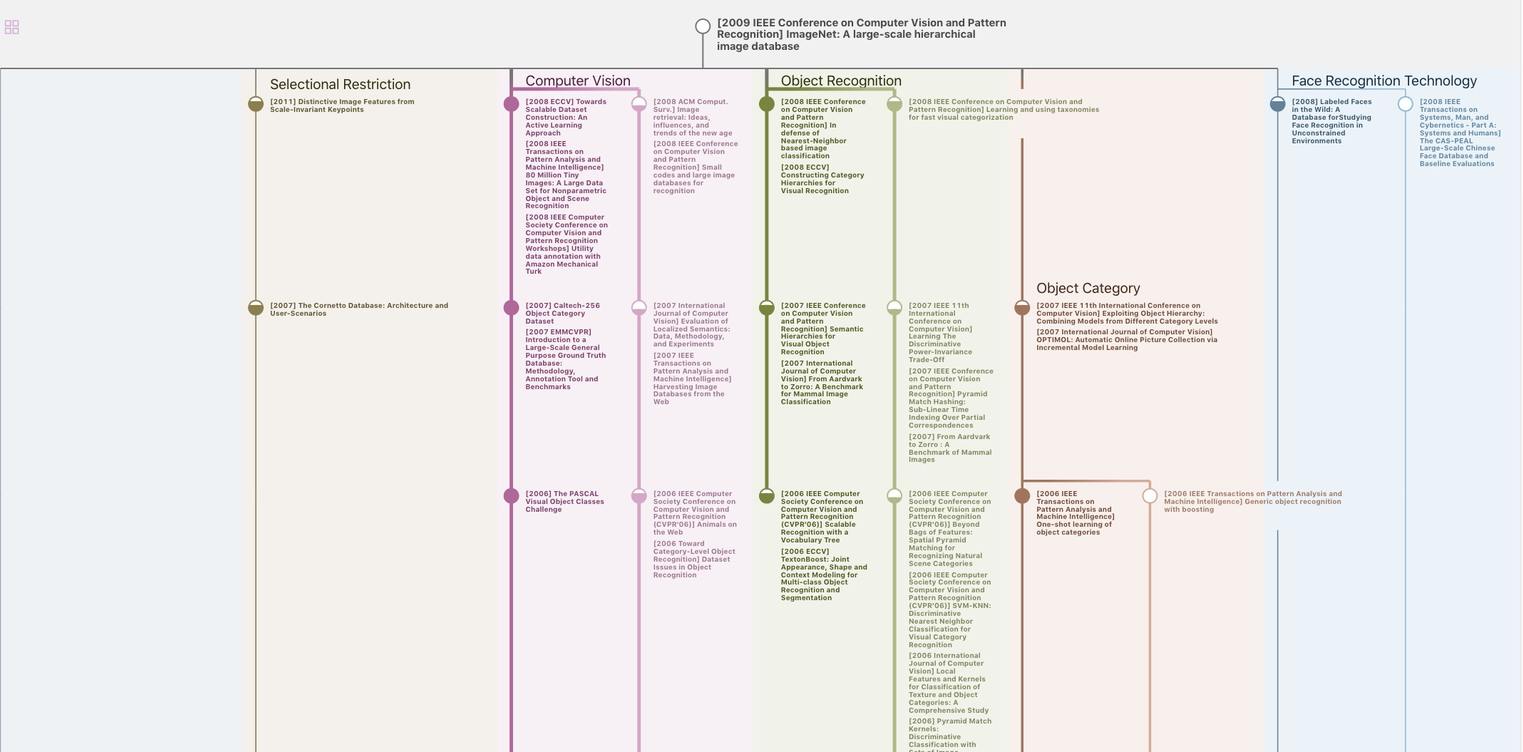
生成溯源树,研究论文发展脉络
Chat Paper
正在生成论文摘要